Markov Chain Monte Carlo-Based Bayesian Inference For Learning Finite And Infinite Inverted Beta-Liouville Mixture Models
IEEE ACCESS(2021)
摘要
Recently Inverted Beta-Liouville mixture models have emerged as an efficient paradigm for proportional positive vectors modeling and unsupervised learning. However, little attention has been devoted to investigate these generative models within discriminative classifiers. Our aim here is to reveal the structure of non-Gaussian data by generating new probabilistic SVM kernels from inverted-Beta Liouville mixture models. The inverted Beta-Liouville has a more general covariance structure and a smaller number of parameters than the inverted Dirichlet and generalized inverted Dirichlet, respectively, which makes it more practical and useful. A principled Bayesian learning algorithm is developed to accurately estimate the model's parameters. To cope with the problem of selecting the optimal number of components, we further propose a nonparametric Bayesian learning algorithm based on an extended infinite mixture model which may have better modelling and clustering capabilities than the finite model for some applications. Finally, the resulting generative model is exploited to build several efficient probabilistic SVM kernels in order to enhance the expected clustering and modeling performance. Through a number of experimental evaluations involving visual scenes classification, text categorization and texture images discrimination, we prove the merits of the proposed work.
更多查看译文
关键词
Mixture models, Hidden Markov models, Data models, Bayes methods, Modeling, Support vector machines, Task analysis, Finite and infinite mixture models, inverted-Beta Liouville, Bayesian learning, nonparametric inference, MCMC, SVM, text categorization, texture classification
AI 理解论文
溯源树
样例
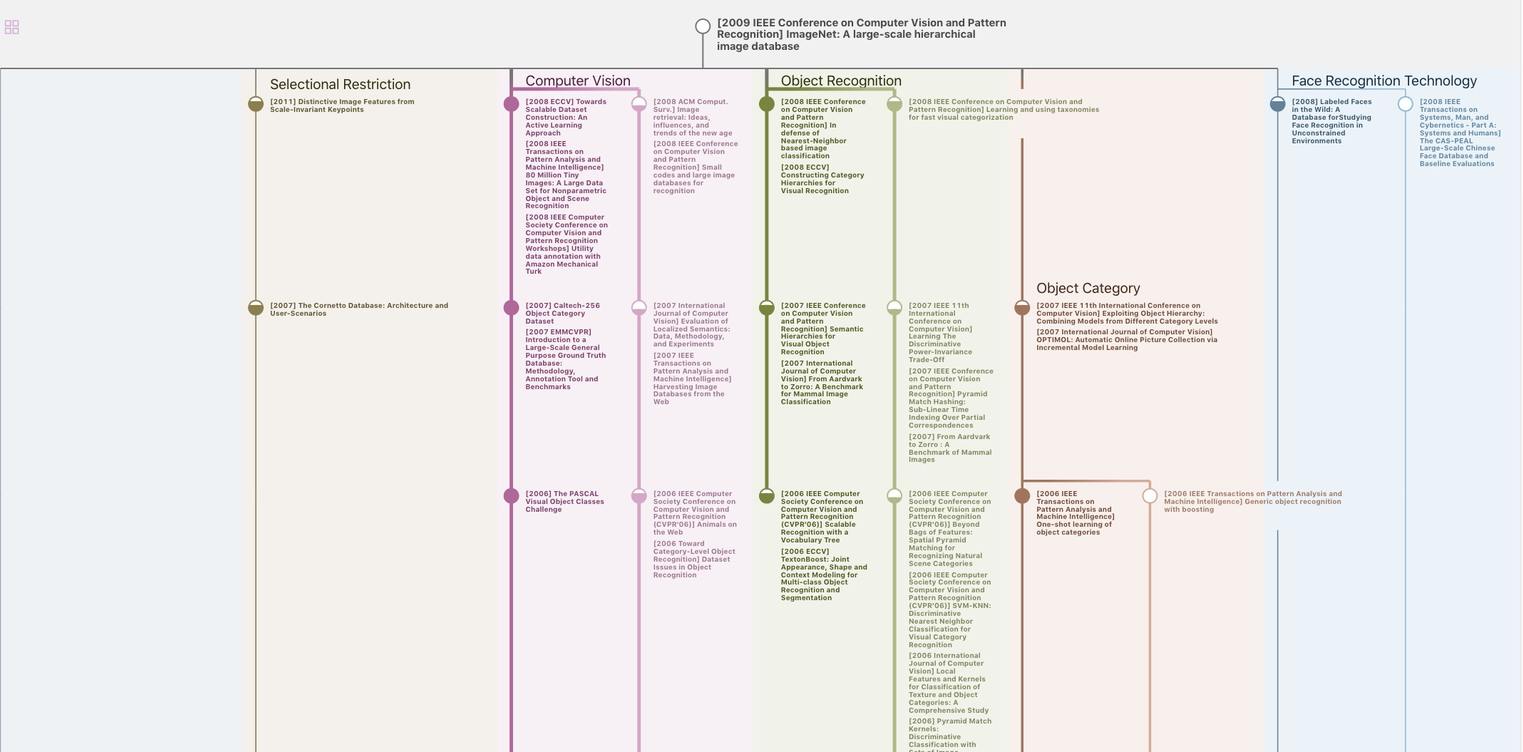
生成溯源树,研究论文发展脉络
Chat Paper
正在生成论文摘要