FAST MANIFOLD LANDMARKING USING EXTREME EIGEN-PAIRS
2021 IEEE INTERNATIONAL CONFERENCE ON ACOUSTICS, SPEECH AND SIGNAL PROCESSING (ICASSP 2021)(2021)
摘要
Manifold landmarking is the problem of selecting a subset of discrete locations on a continuous manifold for label assignment, in order to reduce interpolation error of subsequent semi-supervised learning. In this paper, we select landmarks to minimize the condition number (lambda(max)/lambda(min)) of a submatrix of an alignment matrix Phi, which is equivalent to minimizing an interpolation error bound. Specifically, we design an efficient greedy scheme, where at each iteration t + 1 we choose one landmark i (thus deleting the corresponding row and column i of Phi(t)) so that the resulting submatrix Phi(t+1) has the smallest condition number. Towards fast landmark selection, at iteration t + 1, we first compute the two extreme eignevectors v(1) and v(N) corresponding to lambda(min) and lambda(max) of Phi(t) via known methods like LOBPCG. We show that lambda(min) (lambda(max)) of submatrix Phi(t+1), from deleting the chosen row-column pair, can be approximated by an upper (lower) bound that is an easily computable function of eigen-pair {v(1), lambda(min)} ({v(N), lambda(max)}) of Phi(t). The error bounds of the obtained approximations can be numerically computed during the greedy step. Leveraging these proofs, we minimize a bound of the condition number for submatrix Phi(t+1) at each greedy step t + 1. Experiments on synthetic and real-world manifold data demonstrate the superiority of our proposed landmarking algorithm compared to several state-of-the-art schemes.
更多查看译文
关键词
Manifold landmarking, fast eigenvector computation, Rayleigh quotient theorem
AI 理解论文
溯源树
样例
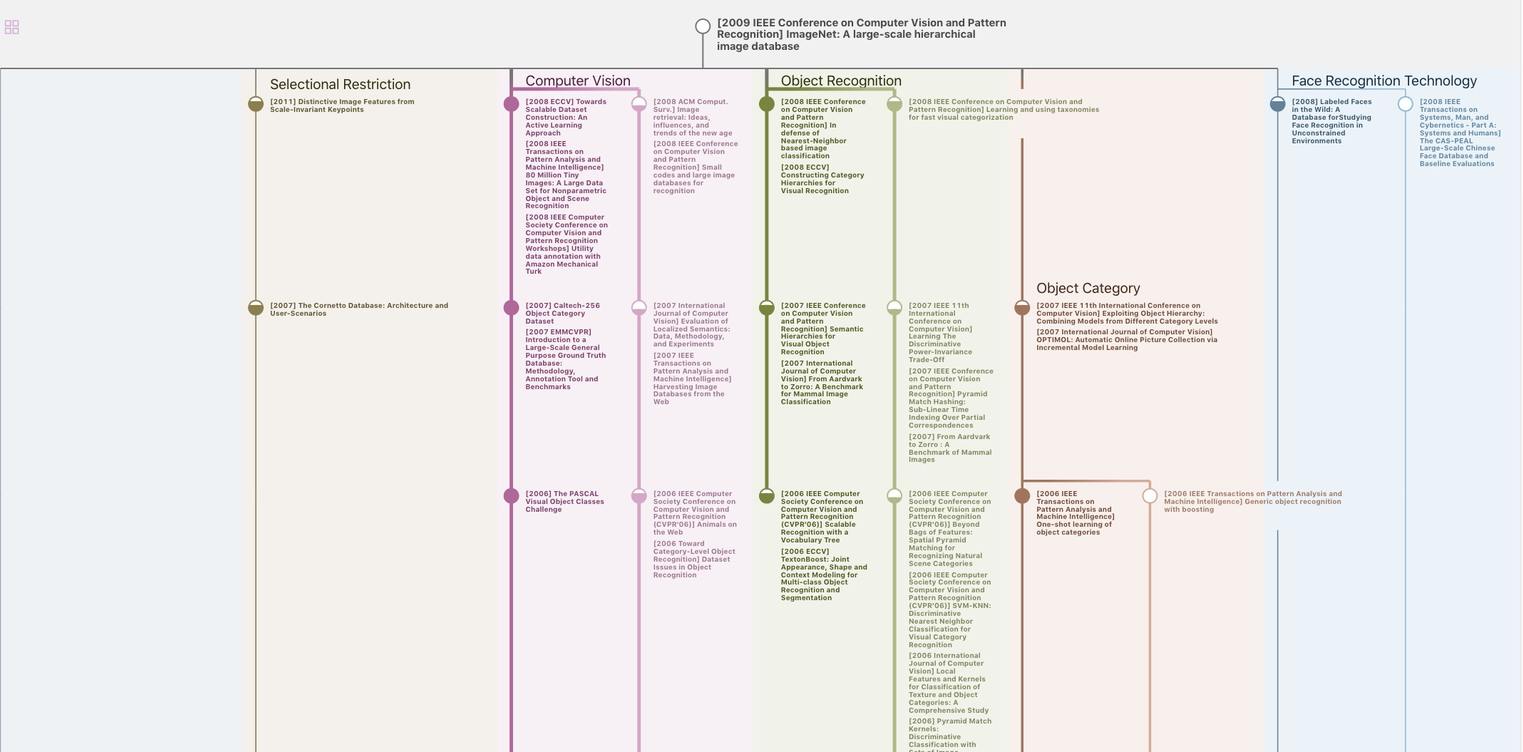
生成溯源树,研究论文发展脉络
Chat Paper
正在生成论文摘要