Fuzzy Graph Learning Regularized Sparse Filtering For Visual Domain Adaptation
APPLIED SCIENCES-BASEL(2021)
摘要
Distribution mismatch can be easily found in multi-sensor systems, which may be caused by different shoot angles, weather conditions and so on. Domain adaptation aims to build robust classifiers using the knowledge from a well-labeled source domain, while applied on a related but different target domain. Pseudo labeling is a prevalent technique for class-wise distribution alignment. Therefore, numerous efforts have been spent on alleviating the issue of mislabeling. In this paper, unlike existing selective hard labeling works, we propose a fuzzy labeling based graph learning framework for matching conditional distribution. Specifically, we construct the cross-domain affinity graph by considering the fuzzy label matrix of target samples. In order to solve the problem of representation shrinkage, the paradigm of sparse filtering is introduced. Finally, a unified optimization method based on gradient descent is proposed. Extensive experiments show that our method achieves comparable or superior performance when compared to state-of-the-art works.
更多查看译文
关键词
domain adaptation, fuzzy graph regularization, sparse filtering
AI 理解论文
溯源树
样例
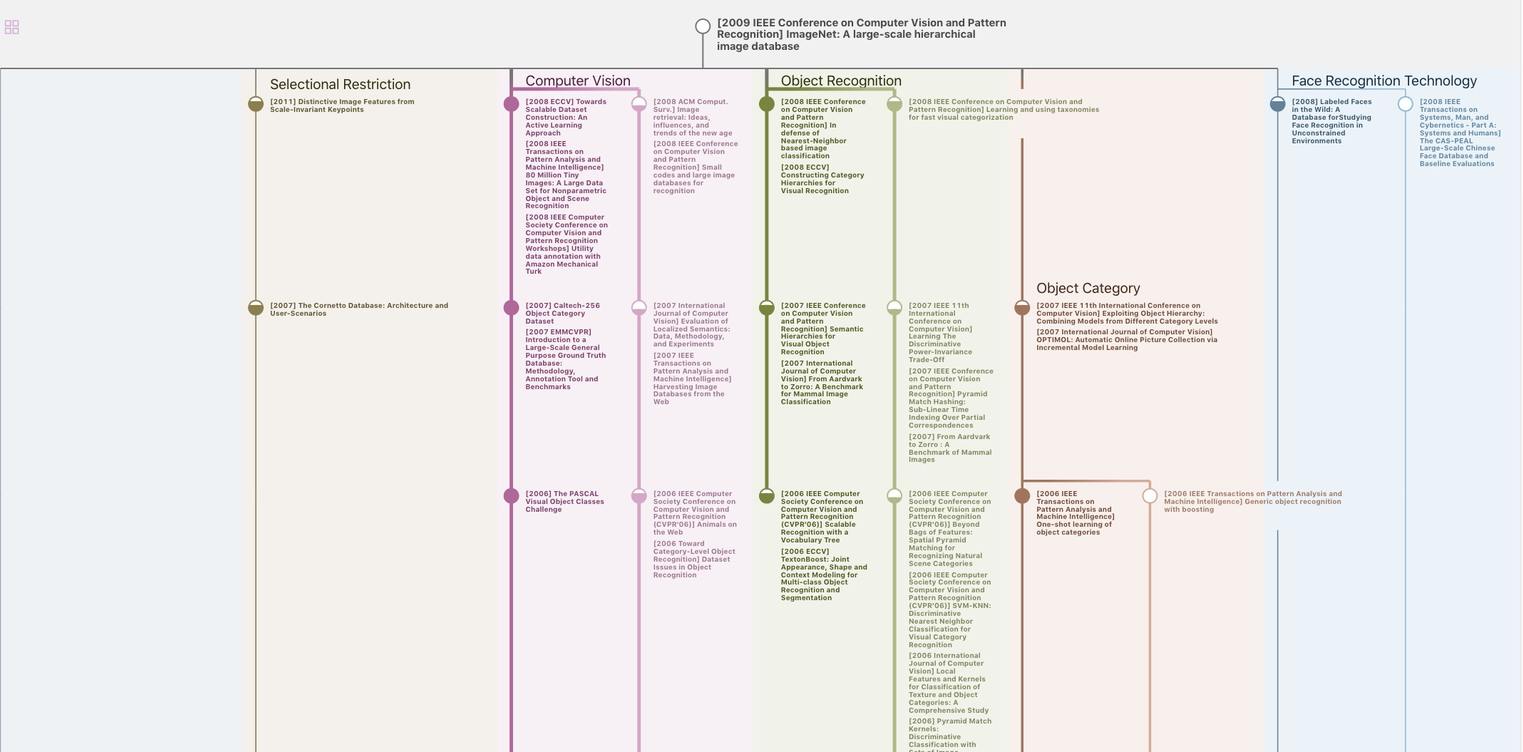
生成溯源树,研究论文发展脉络
Chat Paper
正在生成论文摘要