FDEN: MINING EFFECTIVE INFORMATION OF FEATURES IN DETECTING NETWORK ANOMALIES
2021 IEEE INTERNATIONAL CONFERENCE ON ACOUSTICS, SPEECH AND SIGNAL PROCESSING (ICASSP 2021)(2021)
摘要
Network anomaly detection is important for detecting and reacting to the presence of network attacks. In this paper, we propose a novel method to effectively leverage the features in detecting network anomalies, named FDEn, consisting of flow-based Feature Derivation (FD) and prior knowledge incorporated Ensemble models (En(pk)). To mine the effective information in features, 149 features are derived to enrich the feature set of the original data with covering more characteristics of network traffic. To leverage these features effectively, an ensemble model En(pk), including CatBoost and XGBoost, based on the bagging strategy is proposed to first detect anomalies by combining numerical features and categorical features. And then, En(pk) adjusts the predicted label of specific data by incorporating the prior knowledge of network security. We conduct empirically experiments on the data set provided by the Network Anomaly Detection Challenge (NADC), in which we obtain average improvement up to 61.6%, 31.7%, 50.2%, and 45.0%, in terms of the cost score, precision, recall and F1-score, respectively.
更多查看译文
关键词
Network anomaly detection, Feature derivation, CatBoost, XGBoost, Prior knowledge
AI 理解论文
溯源树
样例
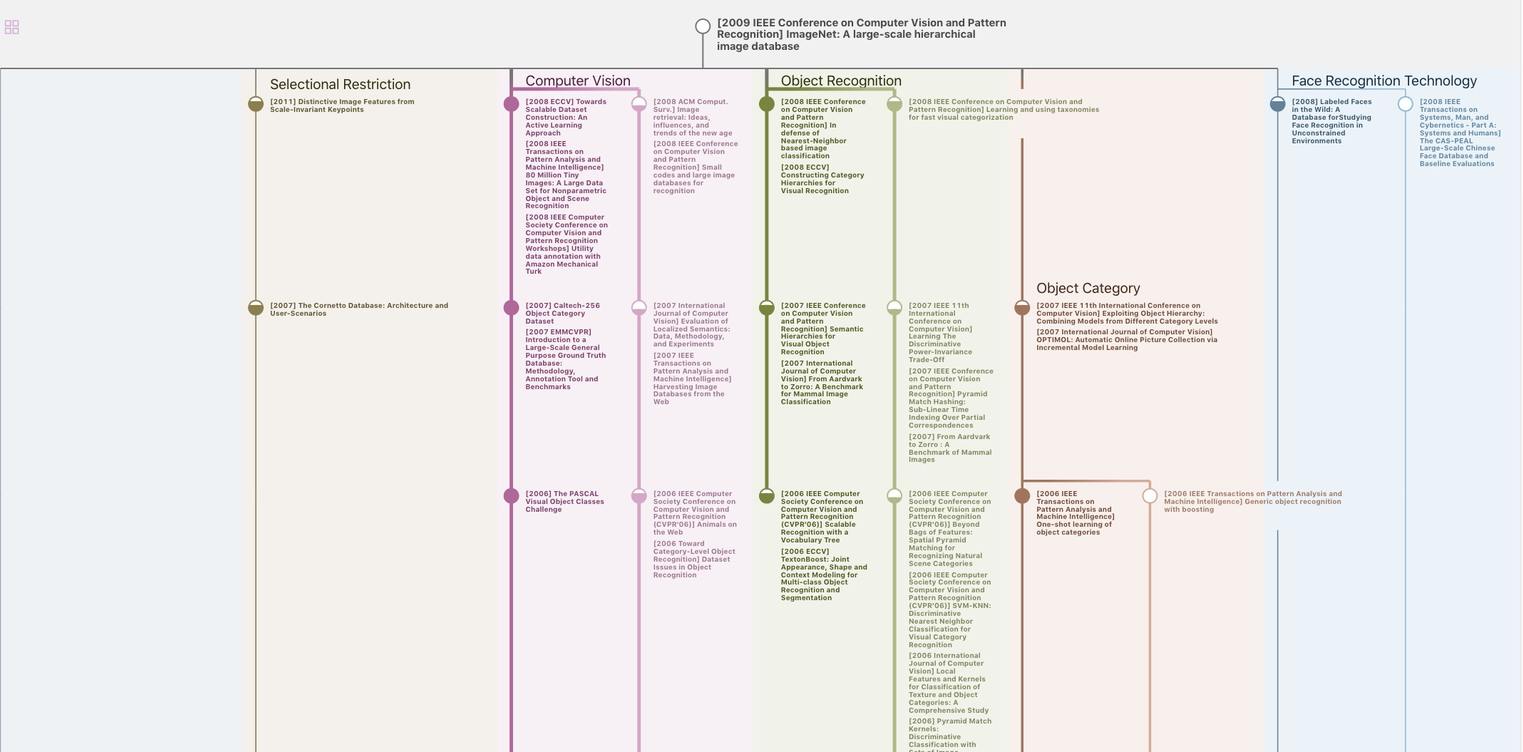
生成溯源树,研究论文发展脉络
Chat Paper
正在生成论文摘要