Emotion recognition using support vector machine and one-dimensional convolutional neural network
MULTIMEDIA TOOLS AND APPLICATIONS(2021)
摘要
Emotion recognition is the most regularly reviewed concept in examining behavioural perception and human-computer interface. The proposed system considers happy, normal and surprise categories of emotions. The dataset comprises mouth images containing emotions in the form of video frames. The Haar-based cascade classifier is used to segment the mouth region in the face images and the video frames are extracted at 20 frames per second. The histogram of oriented gradients (HOG) and local binary pattern (LBP) are applied to capture the edge and local information from the emotion image by extracting the gradient information and finally combined into a single histogram to form the features where each histogram represents a mouth image. The speeded-up robust features (SURF) and scale-invariant feature transform (SIFT) methods are employed to extract unique points in emotion image with varying number of key-points as features. The support vector machine (SVM) and one-dimensional convolutional neural network (1D-CNN) are trained using these texture features. Trained models are utilized to detect emotions in testing video frames. The experimental results depict that SVM and 1D-CNN achieve an accuracy of 97.44% and 98.51% respectively.
更多查看译文
关键词
Convolutional neural network (CNN), Feature extraction, Mouth detection, Histogram of oriented gradients (HOG), Local binary pattern (LBP), Scale-invariant feature transform (SIFT), Speeded-up robust features (SURF), Support vector machine (SVM)
AI 理解论文
溯源树
样例
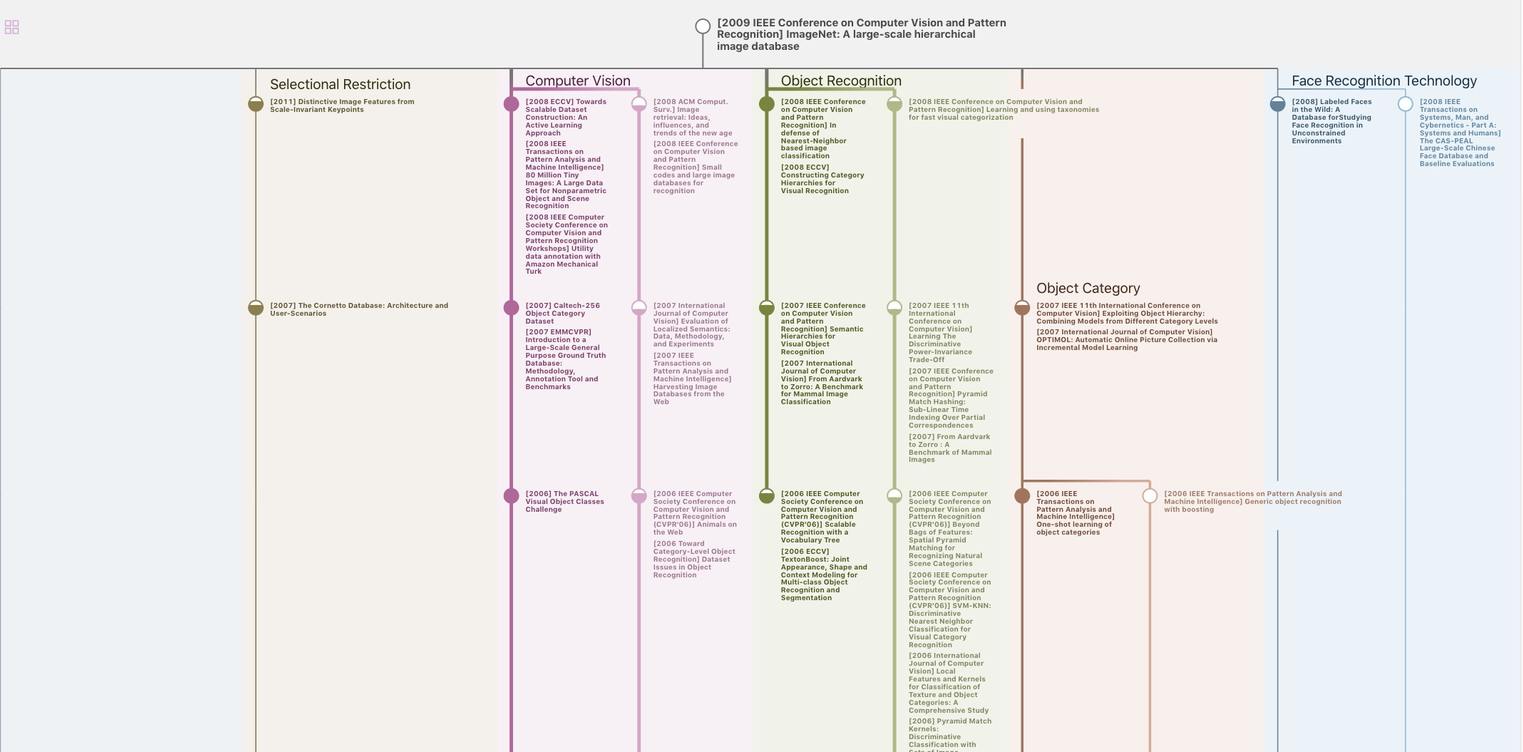
生成溯源树,研究论文发展脉络
Chat Paper
正在生成论文摘要