A DECENTRALIZED VARIANCE-REDUCED METHOD FOR STOCHASTIC OPTIMIZATION OVER DIRECTED GRAPHS
2021 IEEE INTERNATIONAL CONFERENCE ON ACOUSTICS, SPEECH AND SIGNAL PROCESSING (ICASSP 2021)(2021)
摘要
In this paper, we propose a decentralized first-order stochastic optimization method Push-SAGA for finite-sum minimization over a strongly connected directed graph. This method features local variance reduction to remove the uncertainty caused by random sampling of the local gradients, global gradient tracking to address the distributed nature of the data, and push-sum consensus to handle the imbalance caused by the directed nature of the underlying graph. We show that, for a sufficiently small step-size, Push-SAGA linearly converges to the optimal solution for smooth and strongly convex problems, making it the first linearly-convergent stochastic algorithm over arbitrary strongly-connected directed graphs. We illustrate the behavior and convergence properties of Push-SAGA with the help of numerical experiments for strongly convex and non-convex problems.
更多查看译文
关键词
Stochastic optimization, first-order methods, variance reduction, decentralized algorithms, directed graphs
AI 理解论文
溯源树
样例
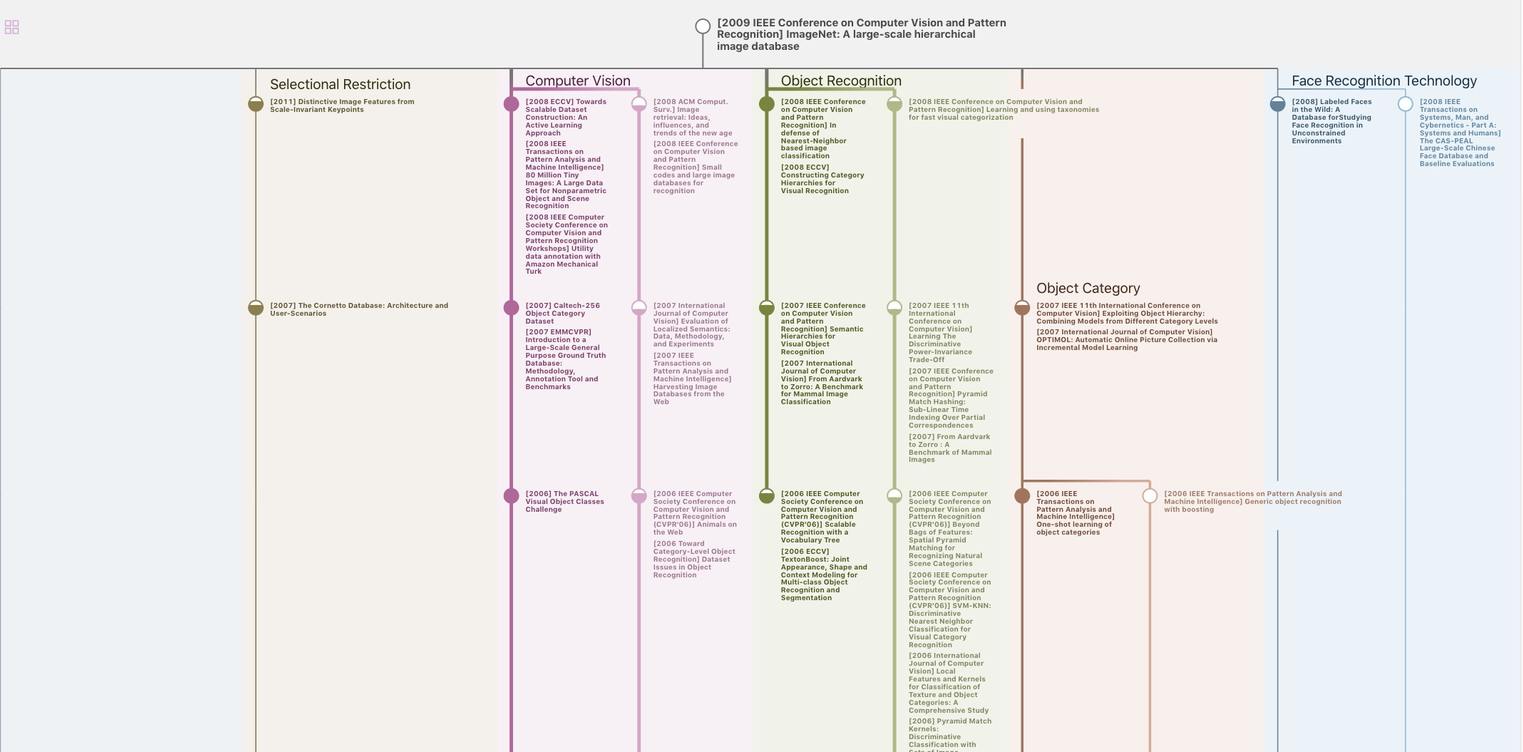
生成溯源树,研究论文发展脉络
Chat Paper
正在生成论文摘要