Adaptive Thresholding Technique Based Classification Of Red Blood Cell And Sickle Cell Using Naive Bayes Classifier And K-Nearest Neighbor Classifier
BIOMEDICAL SIGNAL PROCESSING AND CONTROL(2021)
摘要
Detection of anomalous cells by analyzing the microscopic blood smear plays a key role in identification of various blood diseases in medical field. It becomes easier with robust segmentation steps followed by a classifier for automatic recognition of normal as well as anomalous blood cells. Sickle cell anemia is a disease that changes shape of the red blood cell (RBC) into sickle cell which can be detected by use of automatic classifier. In this reported work, a novel robust hybrid segmentation method is introduced for segmenting normal RBCs and sickle cells. The hybrid segmentation process proposed here is a combination of two segmentation methods namely fuzzy C-means and adaptive (local) thresholding. Four different adaptive thresholding methods are experimented here in combination with fuzzy C-means. The features extracted for carrying out this analysis are major axis, minor axis, aspect ratio, area, perimeter, form factor (metric value), eccentricity and solidity of each of the cells present in the sample blood smear. These eight features are used for training and testing the classifiers. For classification, two supervised classifiers, i.e. Naive Bayes classifier and K-nearest neighbor classifier have been demonstrated on a dataset of 10 image data samples and the evaluated results are compared for each of the hybrid combinations. The comparison shows that the proposed hybrid combination of fuzzy C-means and NICK's thresholding alongwith K-nearest neighbor classifier gives the best performance in terms of the considered performance parameters. This combination gives a significant classification accuracy of 98.87% with lowest computational time.
更多查看译文
关键词
Sickle cell anemia, Adaptive thresholding, Supervised classifier, Naive Bayes classifier, K-nearest neighbor classifier
AI 理解论文
溯源树
样例
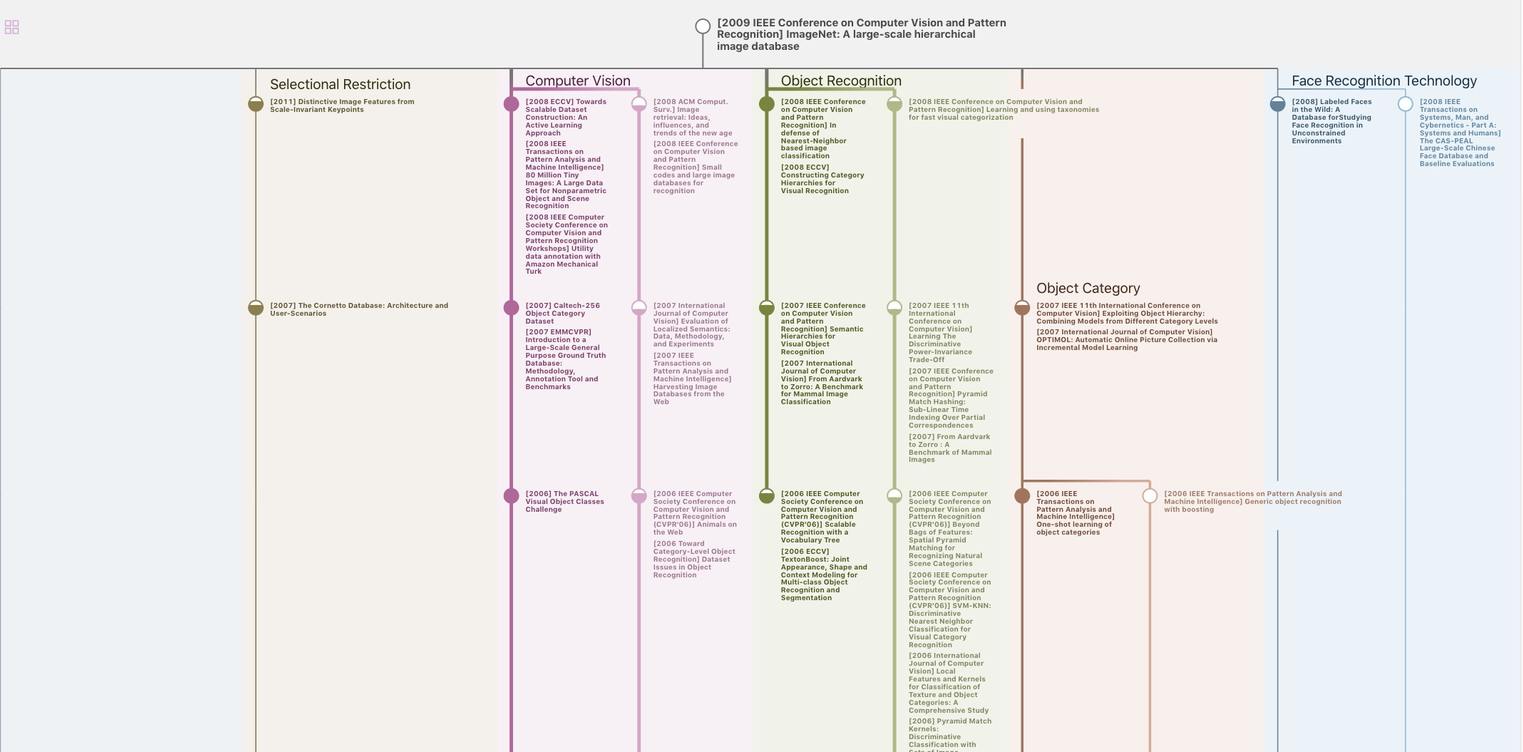
生成溯源树,研究论文发展脉络
Chat Paper
正在生成论文摘要