Personalized Regularization Learning for Fairer Matrix Factorization
ADVANCES IN KNOWLEDGE DISCOVERY AND DATA MINING, PAKDD 2021, PT II(2021)
摘要
Matrix factorization is a canonical method for modeling user preferences for items. Regularization of matrix factorization models often uses a single hyperparameter tuned globally based on metrics evaluated on all data. However, due to the differences in the structure of per-user data, a globally optimal value may not be locally optimal for each individual user, leading to an unfair disparity in performance. Therefore, we propose to tune individual regularization parameters for each user. Our approach, personalized regularization learning (PRL), solves a secondary learning problem of finding the per-user regularization parameters by back-propagating through alternating least squares. Experiments on a benchmark dataset with different user group splits show that PRL outperforms existing methods in improving model performance for disadvantaged groups. We also analyze the learned parameters, finding insights into the effect of regularization on subpopulations with varying properties.
更多查看译文
关键词
Matrix factorization, Fairness, Error disparity, Personalized regularization
AI 理解论文
溯源树
样例
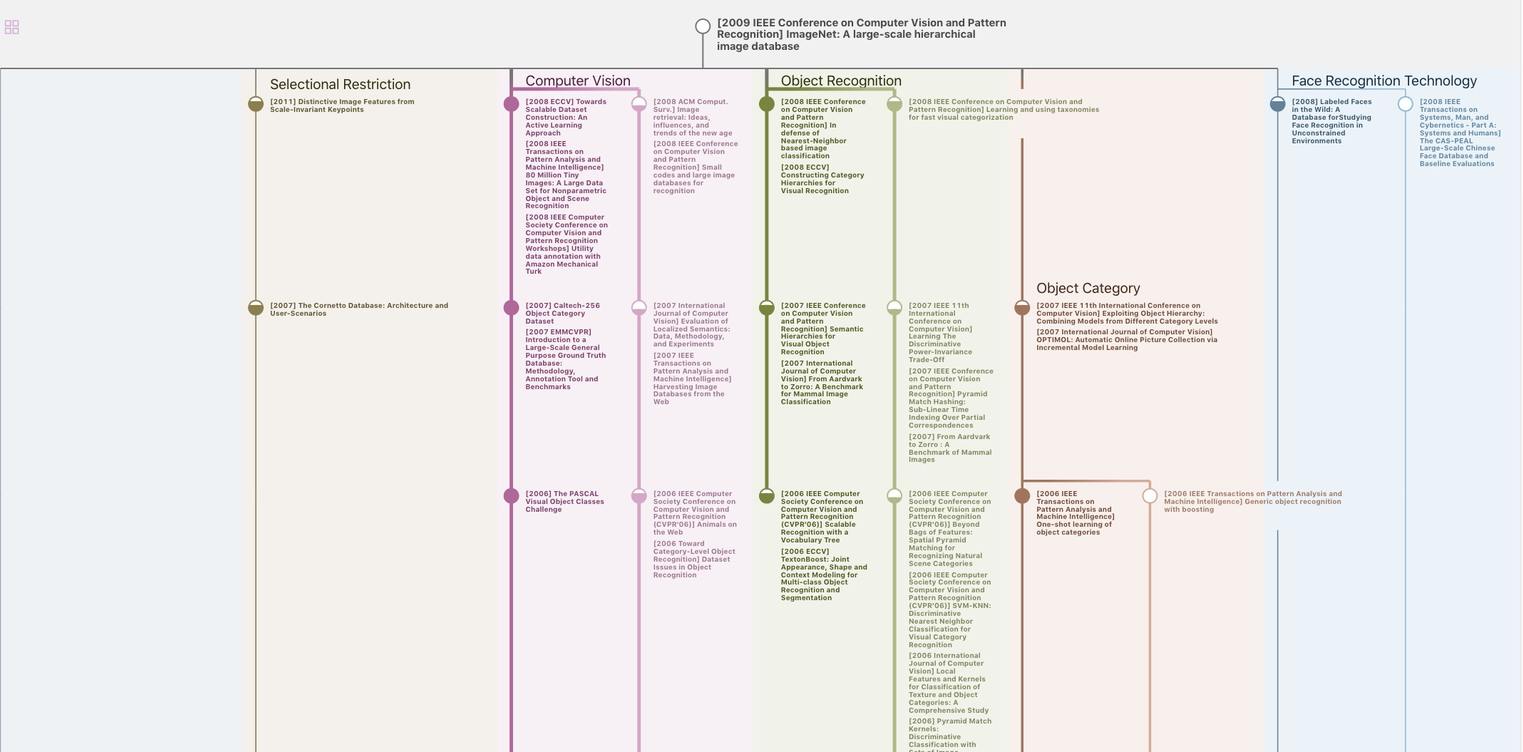
生成溯源树,研究论文发展脉络
Chat Paper
正在生成论文摘要