Collaborative filtering via factorized neural networks
APPLIED SOFT COMPUTING(2021)
摘要
Plenty of deep learning models have been extensively studied over recent years to meet the needs of personalized services. In order to improve the recommendation quality, neural networks are devised more and more complex. As a result, a large number of parameters emerge in the network which significantly increases the burden of computation and storage. When these deep learning models run on the resource-limited devices, their applications are immensely restrained. In consequence, to reduce parameters in the network is of great importance in the aspect of application. In this paper, the factorized neural network model (FNN) is proposed to solve such a problem. The conventional matrix factorization method is used to decompose the weight matrix of each layer in the fully-connected network and the regularization is applied to place constraints on parameters. By our method, more than 95% of parameters in the weighted matrices can be reduced and there is a less than 1% accuracy drop. (c) 2021 Elsevier B.V. All rights reserved. Superscript/Subscript Available 更多
查看译文
关键词
Collaborative filtering, Deep learning, Network compression, Matrix factorization
AI 理解论文
溯源树
样例
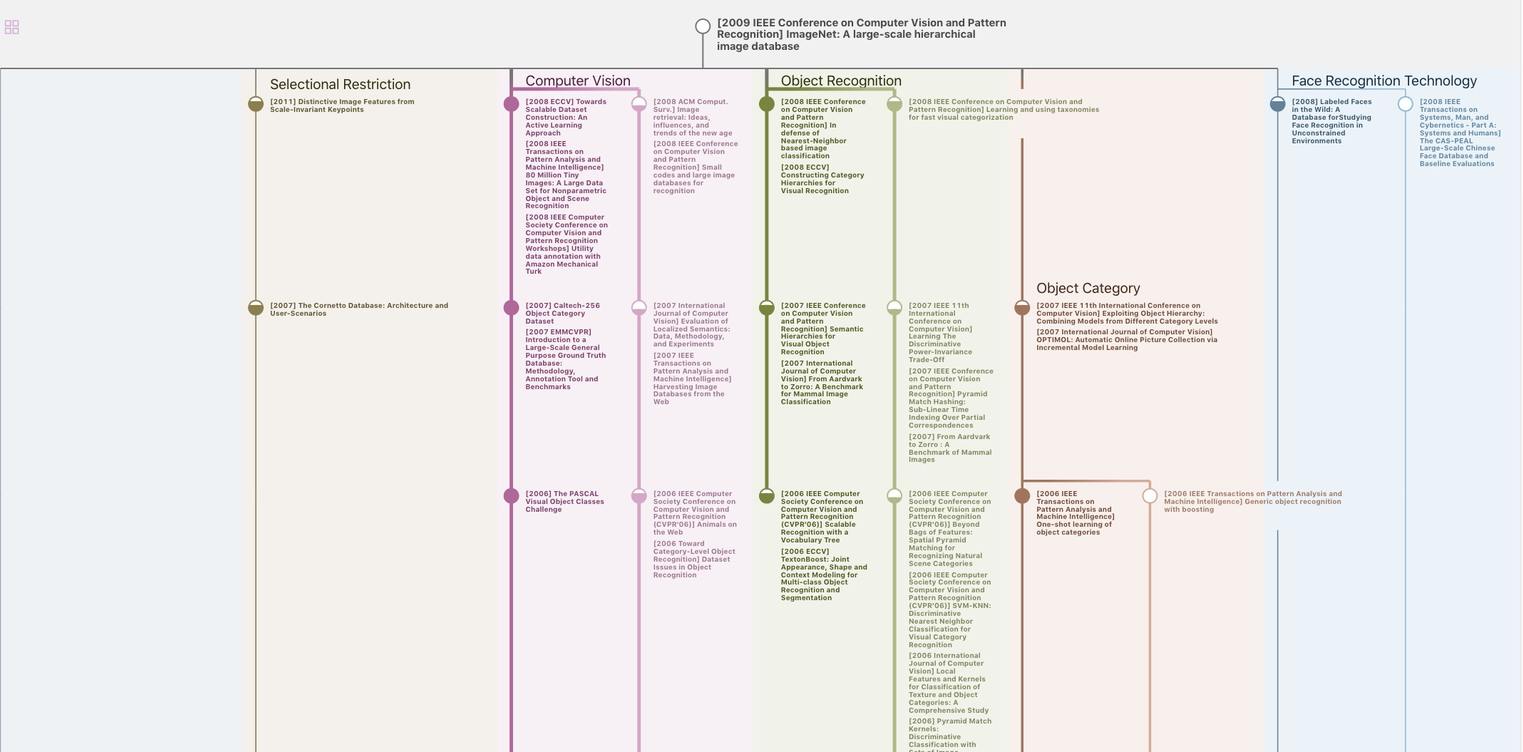
生成溯源树,研究论文发展脉络
Chat Paper
正在生成论文摘要