A Novel Deep Neural Network Based On An Unsupervised Feature Learning Method For Rotating Machinery Fault Diagnosis
MEASUREMENT SCIENCE AND TECHNOLOGY(2021)
摘要
As a simple and unsupervised feature learning method, sparse filtering has shown potential in rotating machinery fault diagnosis. However, sparse filtering has the following deficiencies: (a) the optimal sparsity of the learned features cannot be determined. (b) As a shallow network, sparse filtering has a limited capability of learning discriminative features under varying loads. (c) The diagnostic accuracy and robustness are insufficient. To overcome these deficiencies, variant sparse filtering (VSF), which can determine the optimal sparsity, is developed. Then, a deep variant sparse filtering network (DVSFN) is constructed by using stacked VSF to enhance the capability of learning discriminative features. Finally, a novel fault diagnosis method using the DVSFN is presented and verified by using rolling bearing and planetary gearbox datasets. The optimal sparsity of the learned features is determined by parametric analysis. The experimental results show that the DVSFN can adaptively learn discriminative features, irrespective of the varying loads, and the developed diagnostic method can achieve higher testing accuracy and stronger robustness in comparison to classic data-driven methods.
更多查看译文
关键词
fault diagnosis, deep learning, feature learning, rotating machinery, variant sparse filtering
AI 理解论文
溯源树
样例
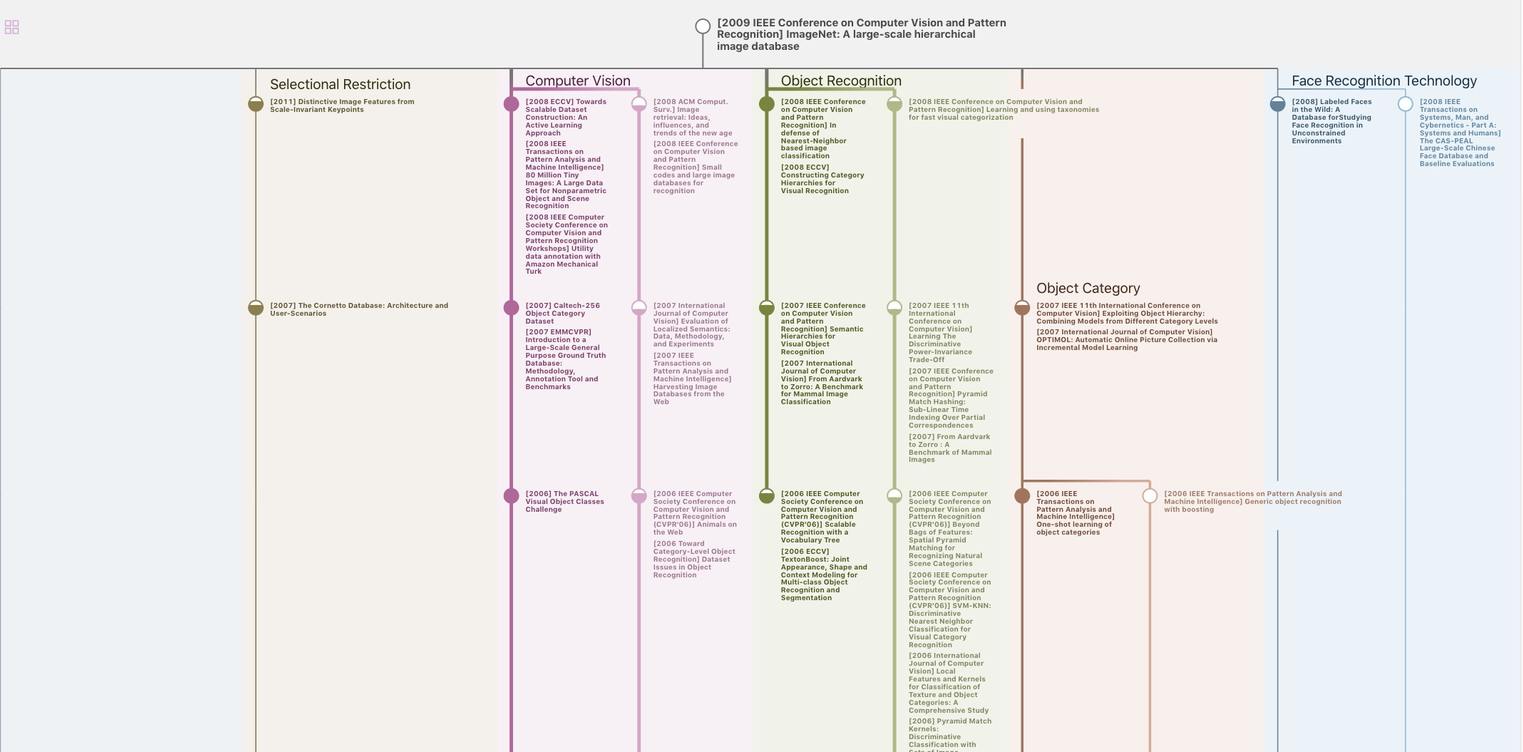
生成溯源树,研究论文发展脉络
Chat Paper
正在生成论文摘要