Neural Network For Metal Detection Based On Magnetic Impedance Sensor
SENSORS(2021)
摘要
The efficiency of the metal detection method using deep learning with data obtained from multiple magnetic impedance (MI) sensors was investigated. The MI sensor is a passive sensor that detects metal objects and magnetic field changes. However, when detecting a metal object, the amount of change in the magnetic field caused by the metal is small and unstable with noise. Consequently, there is a limit to the detectable distance. To effectively detect and analyze this distance, a method using deep learning was applied. The detection performances of a convolutional neural network (CNN) and a recurrent neural network (RNN) were compared from the data extracted from a self-impedance sensor. The RNN model showed better performance than the CNN model. However, in the shallow stage, the CNN model was superior compared to the RNN model. The performance of a deep-learning-based (DLB) metal detection network using multiple MI sensors was compared and analyzed. The network was detected using long short-term memory and CNN. The performance was compared according to the number of layers and the size of the metal sheet. The results are expected to contribute to sensor-based DLB detection technology.
更多查看译文
关键词
convolutional neural network, deep learning, magnetic impedance, metal detection, recurrent neural network, sensor, signal processing
AI 理解论文
溯源树
样例
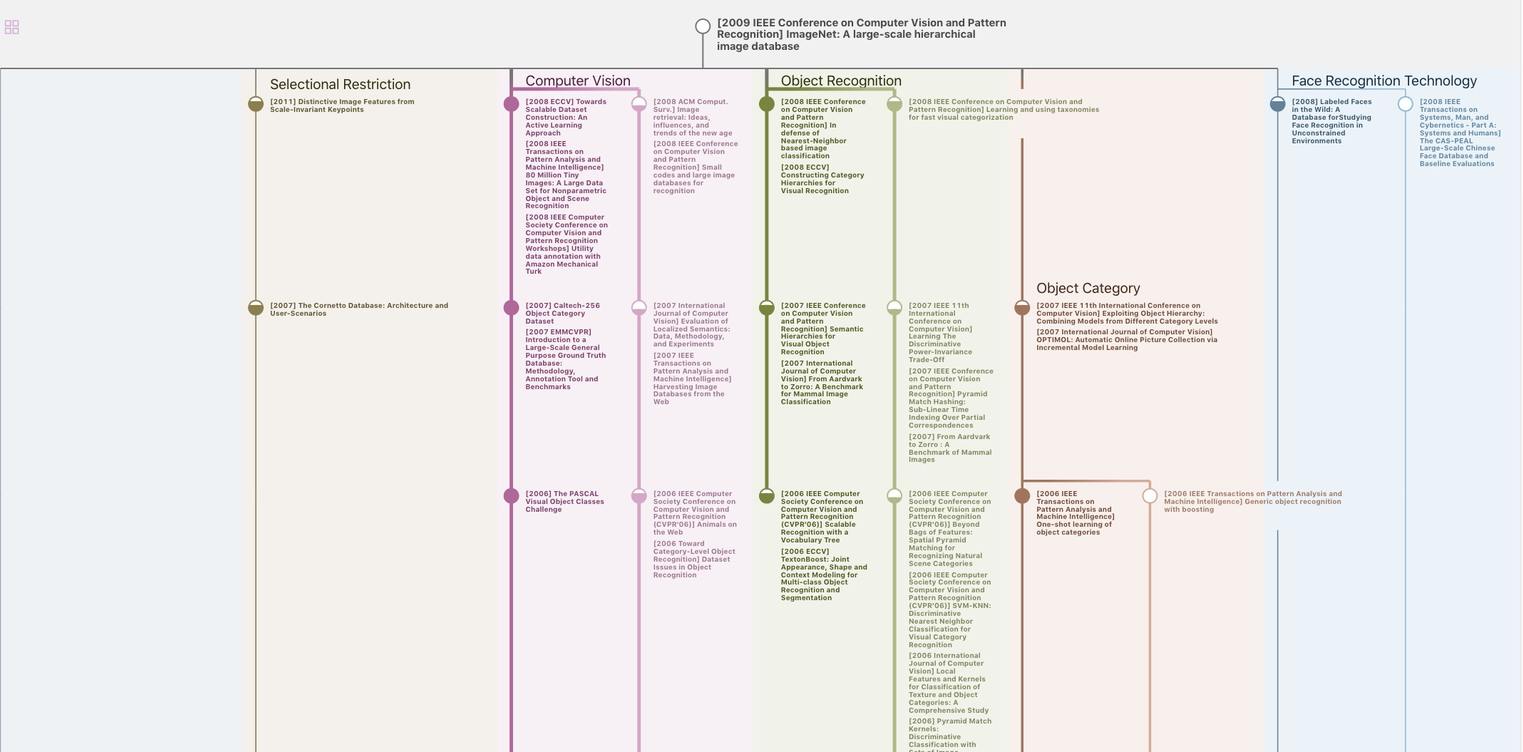
生成溯源树,研究论文发展脉络
Chat Paper
正在生成论文摘要