Vision-Based Tunnel Lining Health Monitoring Via Bi-Temporal Image Comparison And Decision-Level Fusion Of Change Maps
SENSORS(2021)
摘要
Tunnel structural health inspections are predominantly done through periodic visual observations, requiring humans to be physically present on-site, possibly exposing them to hazardous environments. These surveys are subjective (relying on the surveyor experience), time-consuming, and may demand operation shutdown. These issues can be mitigated through accurate automatic monitoring and inspection systems. In this work, we propose a remotely operated machine vision change detection application to improve the structural health monitoring of tunnels. The vision-based sensing system acquires the data from a rig of cameras hosted on a robotic platform that is driven parallel to the tunnel walls. These data are then pre-processed using image processing and deep learning techniques to reduce nuisance changes caused by light variations. Image fusion techniques are then applied to identify the changes occurring in the tunnel structure. Different pixel-based change detection approaches are used to generate temporal change maps. Decision-level fusion methods are then used to combine these change maps to obtain a more reliable detection of the changes that occur between surveys. A quantitative analysis of the results achieved shows that the proposed change detection system achieved a recall value of 81%, a precision value of 93% and an F1-score of 86.7%.
更多查看译文
关键词
computer vision, data fusion, tunnel lining inspections
AI 理解论文
溯源树
样例
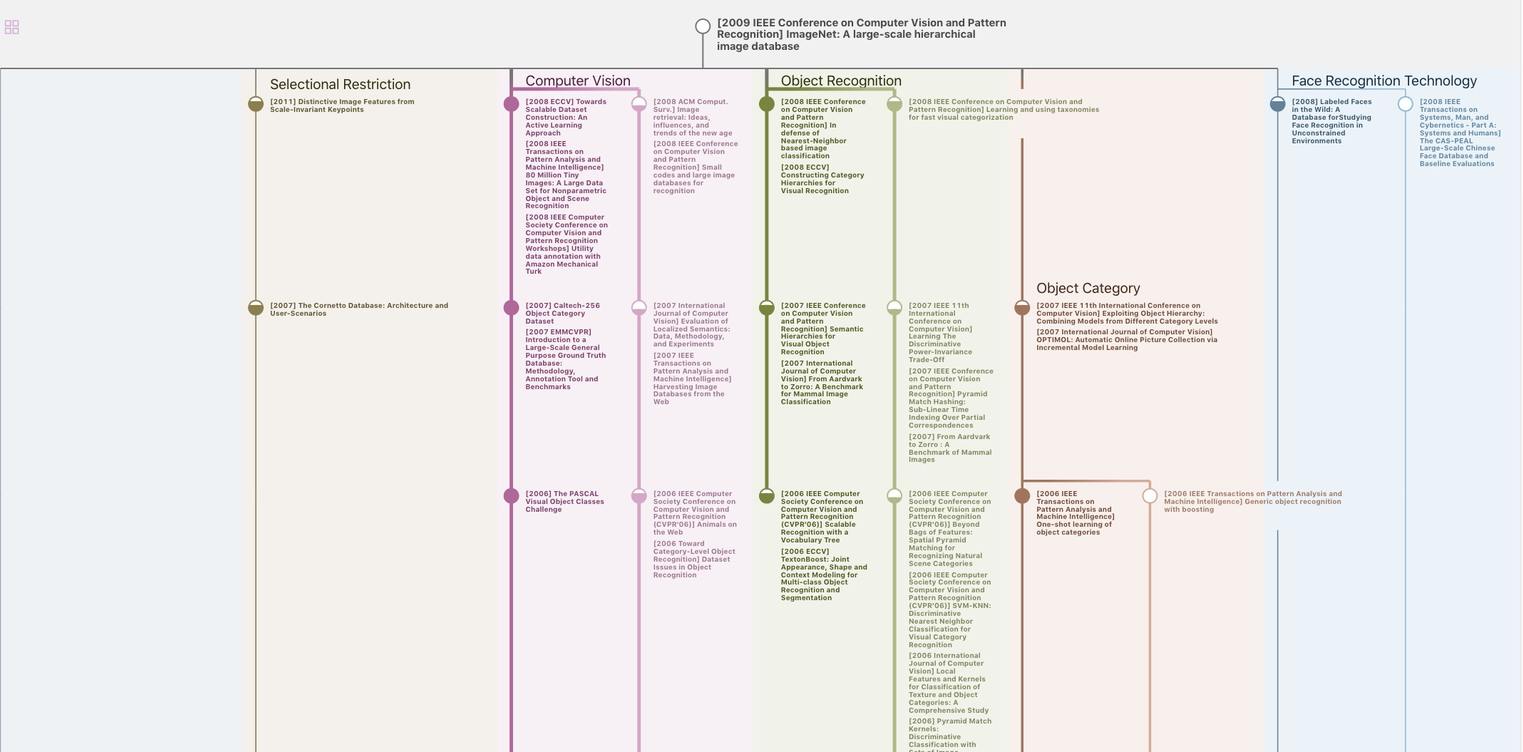
生成溯源树,研究论文发展脉络
Chat Paper
正在生成论文摘要