Experimental Study and Comparison of Imbalance Ensemble Classifiers with Dynamic Selection Strategy.
ENTROPY(2021)
摘要
Imbalance ensemble classification is one of the most essential and practical strategies for improving decision performance in data analysis. There is a growing body of literature about ensemble techniques for imbalance learning in recent years, the various extensions of imbalanced classification methods were established from different points of view. The present study is initiated in an attempt to review the state-of-the-art ensemble classification algorithms for dealing with imbalanced datasets, offering a comprehensive analysis for incorporating the dynamic selection of base classifiers in classification. By conducting 14 existing ensemble algorithms incorporating a dynamic selection on 56 datasets, the experimental results reveal that the classical algorithm with a dynamic selection strategy deliver a practical way to improve the classification performance for both a binary class and multi-class imbalanced datasets. In addition, by combining patch learning with a dynamic selection ensemble classification, a patch-ensemble classification method is designed, which utilizes the misclassified samples to train patch classifiers for increasing the diversity of base classifiers. The experiments' results indicate that the designed method has a certain potential for the performance of multi-class imbalanced classification.
更多查看译文
关键词
dynamic selection, ensemble classification, imbalanced data classification, multi-class classification
AI 理解论文
溯源树
样例
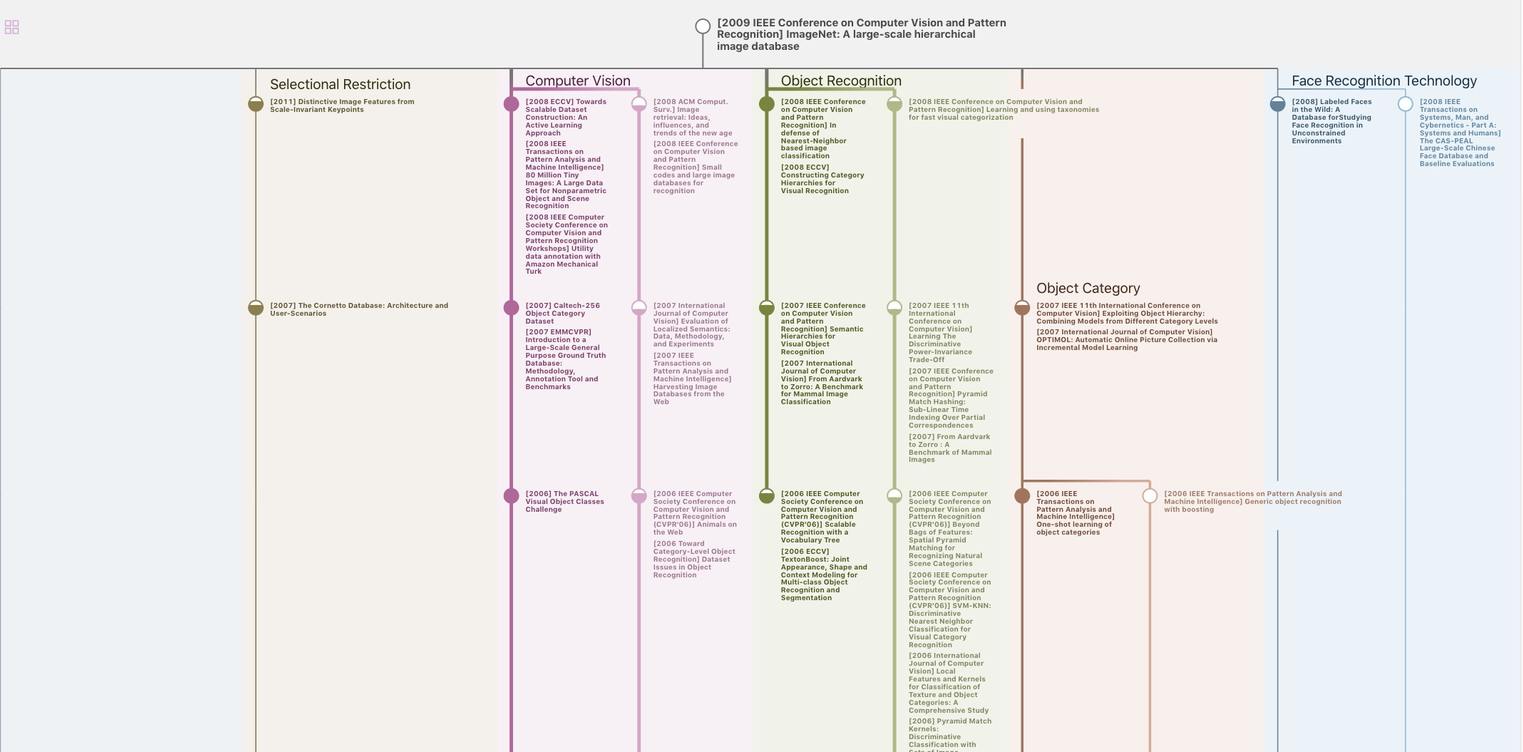
生成溯源树,研究论文发展脉络
Chat Paper
正在生成论文摘要