Spotting adversarial samples for speaker verification by neural vocoders
arxiv(2021)
摘要
Automatic speaker verification (ASV), one of the most important technology for biometric identification, has been widely adopted in security-critic applications, including transaction authentication and access control. However, previous works have shown ASV is seriously vulnerable to recently emerged adversarial attacks, yet effective countermeasures against them are limited. In this paper, we adopt neural vocoders to spot adversarial samples for ASV. We use neural vocoder to re-synthesize audio and find that the difference between the ASV scores for the original and re-synthesized audio is a good indicator to distinguish genuine and adversarial samples. As the very beginning work in this direction of detecting adversarial samples for ASV, there is no reliable baseline for comparison. So we first implement Griffin-Lim for detection and set it as our baseline. The proposed method accomplishes effective detection performance and outperforms all the baselines in all the settings. We also show the neural vocoder adopted in the detection framework is dataset independent. Our codes will be made open-source for future works to do comparison.
更多查看译文
关键词
speaker verification,adversarial samples,neural vocoders
AI 理解论文
溯源树
样例
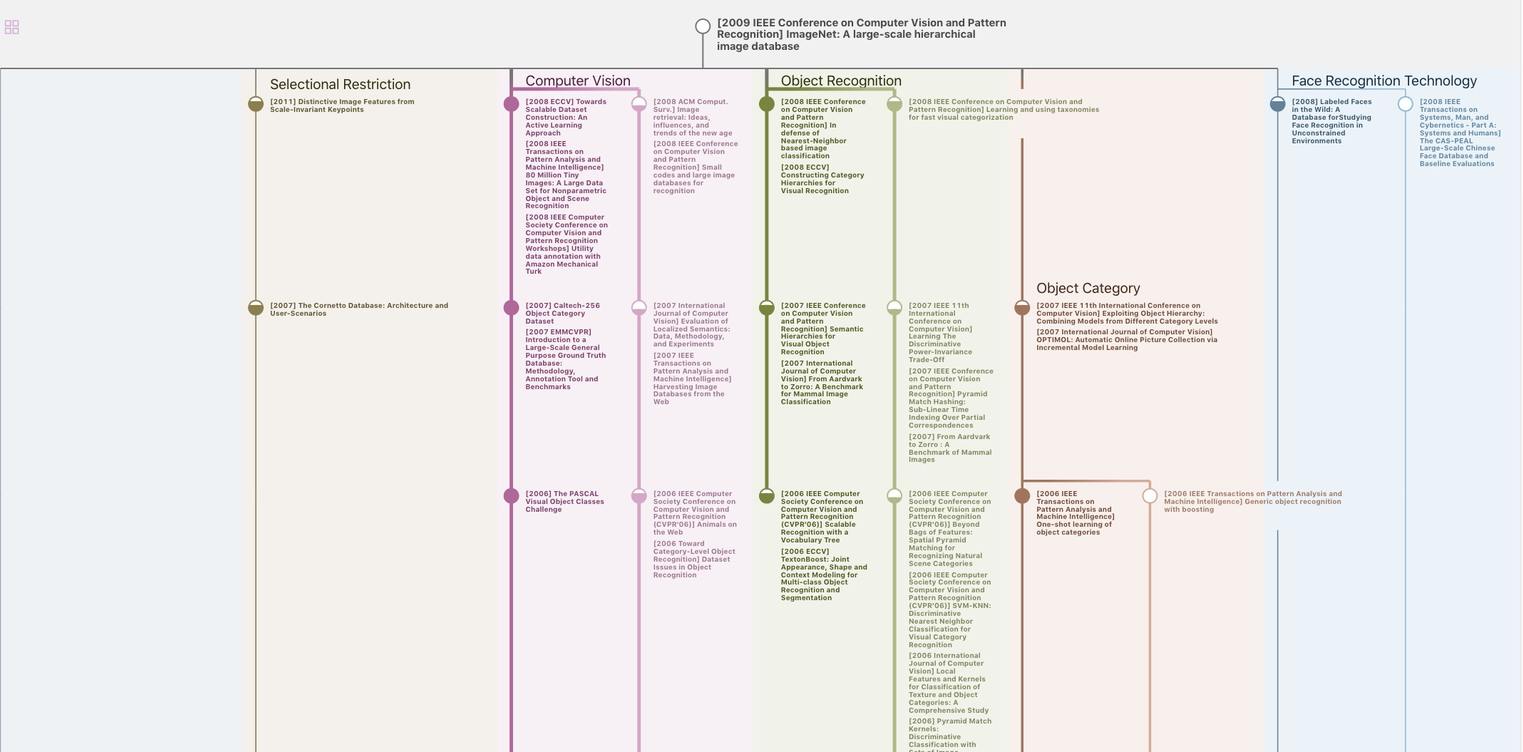
生成溯源树,研究论文发展脉络
Chat Paper
正在生成论文摘要