A Few Interactions Improve Distributed Nonparametric Estimation, Optimally
IEEE TRANSACTIONS ON INFORMATION THEORY(2023)
摘要
Consider the problem of nonparametric estimation of an unknown beta-H & ouml;lder smooth density pXY at a given point, where X and Y are both d dimensional. An infinite sequence of i.i.d. samples (X-i, Y-i) are generated according to this distribution, and two terminals observe (Xi) and (Yi), respectively. They are allowed to exchange k bits either in oneway or interactively in order for Bob to estimate the unknown density. We show that the minimax mean square risk is order ((k)/(log k))(-)(2 beta)/d+2 beta for one-way protocols and k(-)(2 beta)/d+2 beta for interactive k protocols. The logarithmic improvement is nonexistent in the parametric counterparts, and therefore can be regarded as a consequence of nonparametric nature of the problem. Moreover, a few rounds of interactions achieve the interactive minimax rate: the number of rounds can grow as slowly as the super logarithm (i.e., inverse tetration) of k. The proof of the upper bound is based on a novel multi-round scheme for estimating the joint distribution of a pair of biased Bernoulli variables, and the lower bound is built on a sharp estimate of a symmetric strong data processing constant for biased Bernoulli variables.
更多查看译文
关键词
Density estimation,communication complexity,nonparametric statistics,learning with system constraints,strong data processing constant
AI 理解论文
溯源树
样例
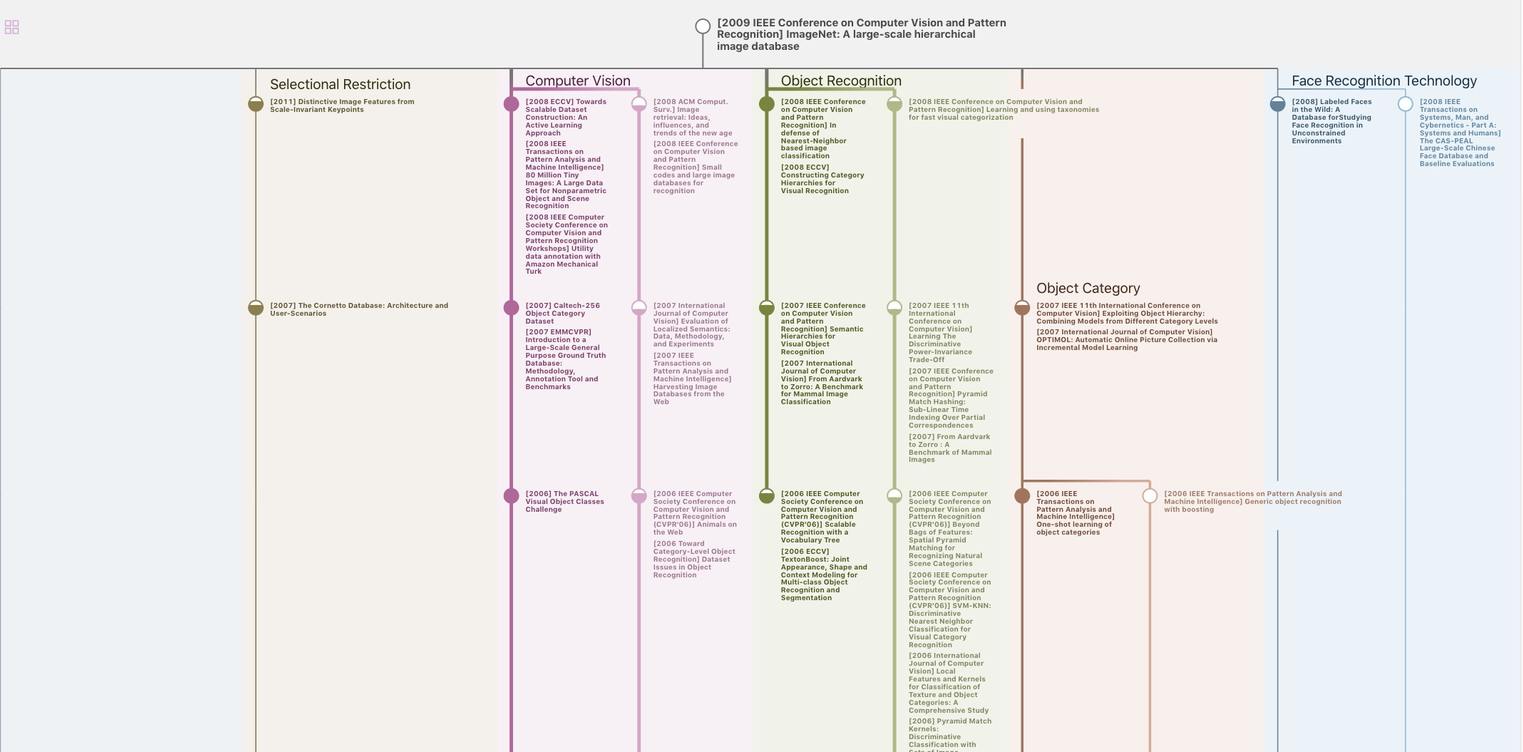
生成溯源树,研究论文发展脉络
Chat Paper
正在生成论文摘要