Parallel graph coloring algorithms for distributed GPU environments
PARALLEL COMPUTING(2022)
摘要
Graph coloring is often used in parallelizing scientific computations that run in distributed and multi-GPU environments; it identifies sets of independent data that can be updated in parallel. Many algorithms exist for graph coloring on a single GPU or in distributed memory, but to the best of our knowledge, hybrid MPI+GPU algorithms have been unexplored until this work. We present several MPI+GPU coloring approaches based on the distributed coloring algorithms of Gebremedhin et al. and the shared-memory algorithms of Deveci et al. The on-node parallel coloring uses implementations in KokkosKernels, which provide parallelization for both multicore CPUs and GPUs. We further extend our approaches to compute distance-2 and partial distance-2 colorings, giving the first known distributed, multi-GPU algorithm for these problems. In addition, we propose a novel heuristic to reduce communication for recoloring in distributed graph coloring. Our experiments show that our approaches operate efficiently on inputs too large to fit on a single GPU and scale up to graphs with 76.7 billion edges running on 128 GPUs.
更多查看译文
关键词
Graph coloring,Distributed algorithms,GPU,Combinatorial scientific computing
AI 理解论文
溯源树
样例
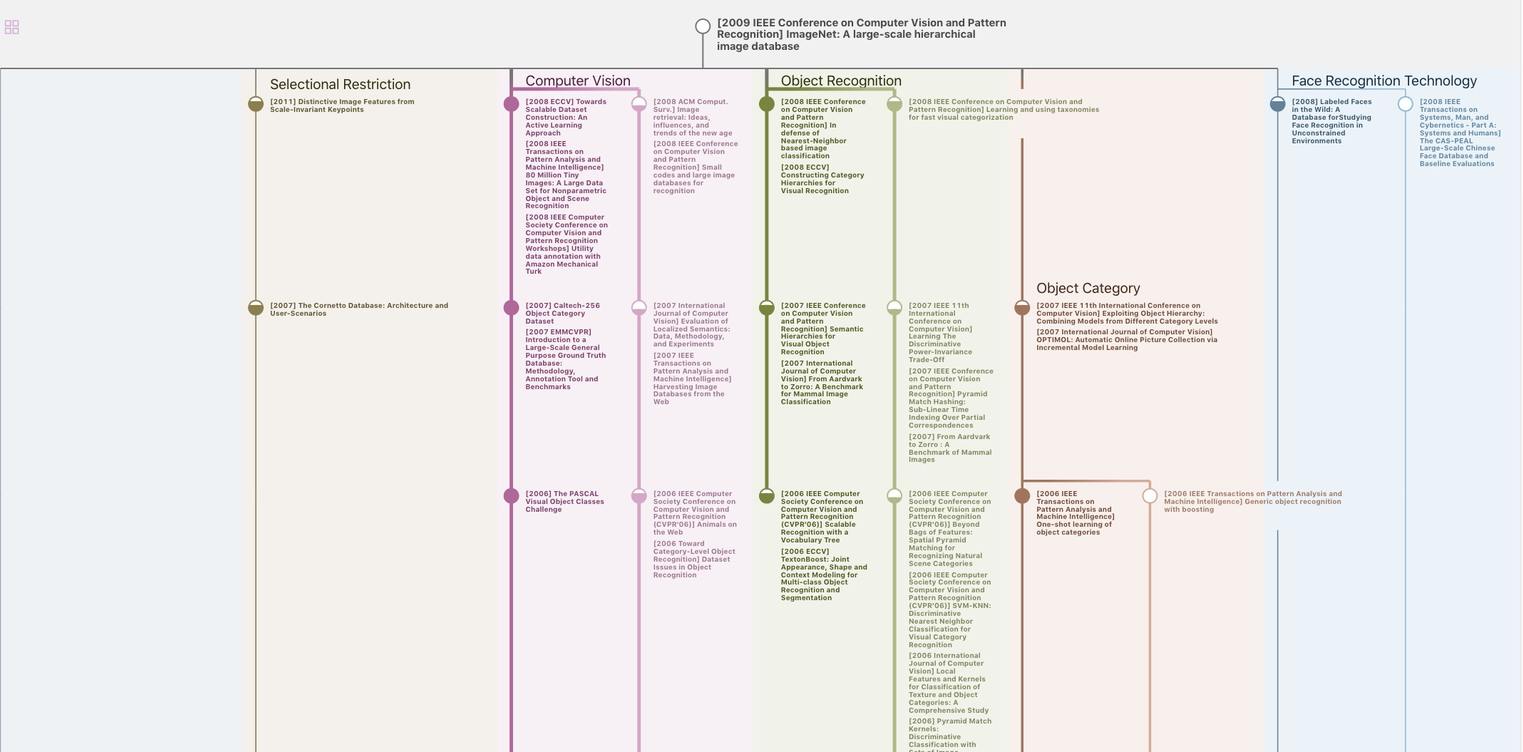
生成溯源树,研究论文发展脉络
Chat Paper
正在生成论文摘要