Learning from Miscellaneous Other-Class Words for Few-shot Named Entity Recognition
Annual Meeting of the Association for Computational Linguistics(2021)
摘要
Few-shot Named Entity Recognition (NER) exploits only a handful of annotations to identify and classify named entity mentions. Prototypical network shows superior performance on few-shot NER. However, existing prototypical methods fail to differentiate rich semantics in other-class words, which will aggravate overfitting under few shot scenario. To address the issue, we propose a novel model, Mining Undefined Classes from Other-class (MUCO), that can automatically induce different undefined classes from the other class to improve few-shot NER. With these extra-labeled undefined classes, our method will improve the discriminative ability of NER classifier and enhance the understanding of predefined classes with stand-by semantic knowledge. Experimental results demonstrate that our model outperforms five state-of-the-art models in both 1-shot and 5-shots settings on four NER benchmarks. We will release the code upon acceptance. The source code is released on https: //github.com/shuaiwa16/OtherClassNER.git.
更多查看译文
关键词
entity,learning,recognition,other-class,few-shot
AI 理解论文
溯源树
样例
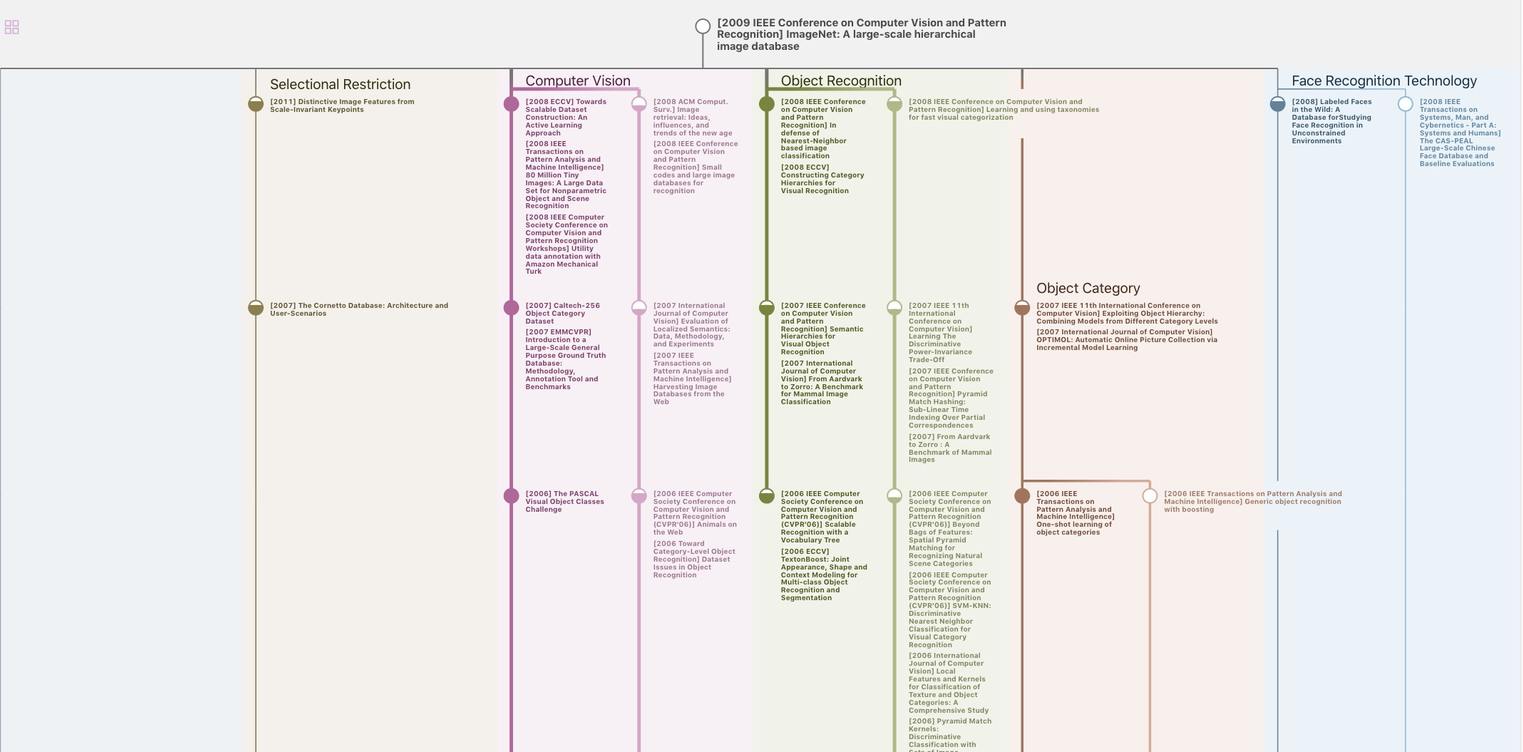
生成溯源树,研究论文发展脉络
Chat Paper
正在生成论文摘要