Cross-domain error minimization for unsupervised domain adaptation
arxiv(2021)
摘要
Unsupervised domain adaptation aims to transfer knowledge from a labeled source domain to an unlabeled target domain. Previous methods focus on learning domain-invariant features to decrease the discrepancy between the feature distributions as well as minimizing the source error and have made remarkable progress. However, a recently proposed theory reveals that such a strategy is not sufficient for a successful domain adaptation. It shows that besides a small source error, both the discrepancy between the feature distributions and the discrepancy between the labeling functions should be small across domains. The discrepancy between the labeling functions is essentially the cross-domain errors which are ignored by existing methods. To overcome this issue, in this paper, a novel method is proposed to integrate all the objectives into a unified optimization framework. Moreover, the incorrect pseudo labels widely used in previous methods can lead to error accumulation during learning. To alleviate this problem, the pseudo labels are obtained by utilizing structural information of the target domain besides source classifier and we propose a curriculum learning based strategy to select the target samples with more accurate pseudo-labels during training. Comprehensive experiments are conducted, and the results validate that our approach outperforms state-of-the-art methods.
更多查看译文
关键词
Transfer learning,Domain adaptation,Cross-domain errors
AI 理解论文
溯源树
样例
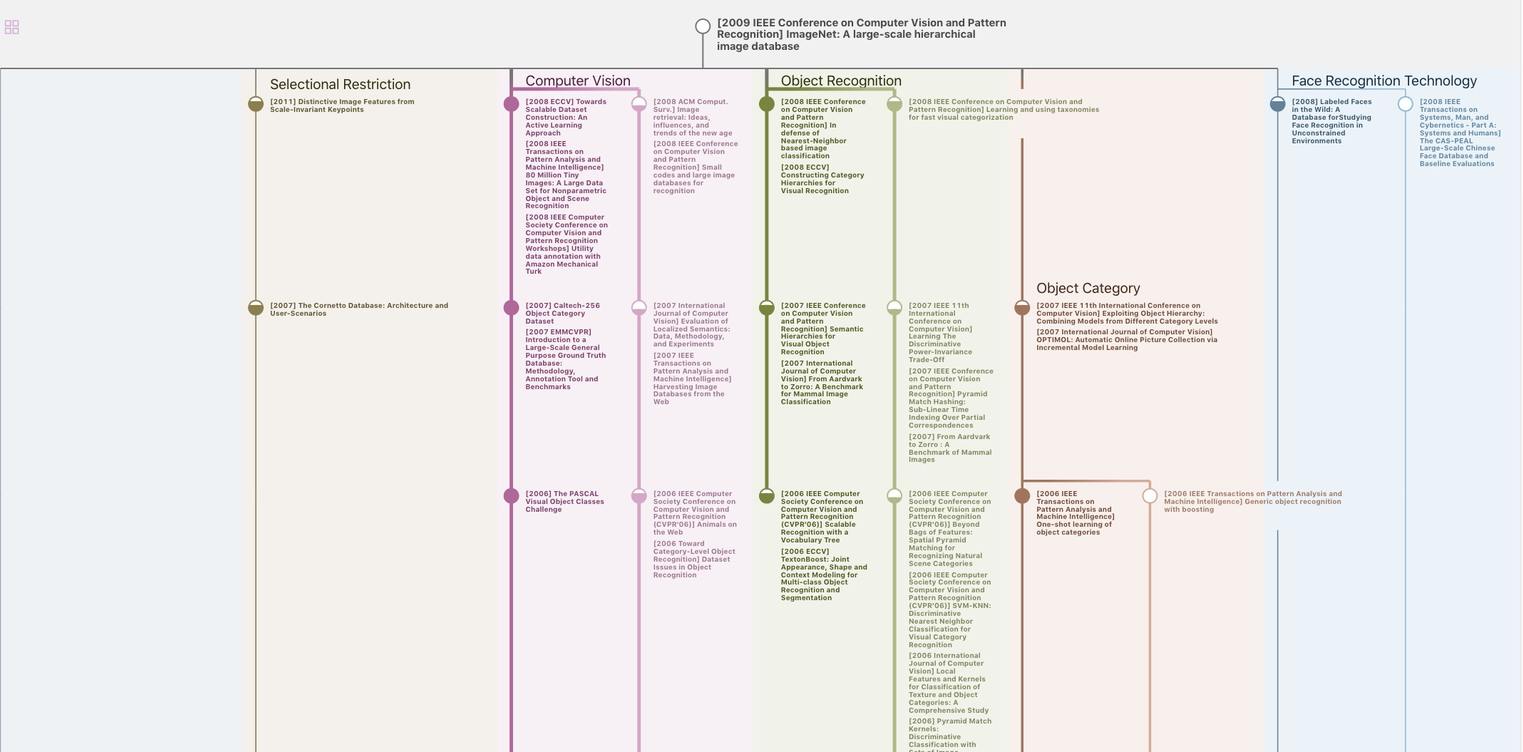
生成溯源树,研究论文发展脉络
Chat Paper
正在生成论文摘要