Variance Reduction for Matrix Computations with Applications to Gaussian Processes.
VALUETOOLS(2021)
摘要
In addition to recent developments in computing speed and memory, methodological advances have contributed to significant gains in the performance of stochastic simulation. In this paper, we focus on variance reduction for matrix computations via matrix factorization. We provide insights into existing variance reduction methods for estimating the entries of large matrices. Popular methods do not exploit the reduction in variance that is possible when the matrix is factorized. We show how computing the square root factorization of the matrix can achieve in some important cases arbitrarily better stochastic performance. In addition, we propose a factorized estimator for the trace of a product of matrices and numerically demonstrate that the estimator can be up to 1,000 times more efficient on certain problems of estimating the log-likelihood of a Gaussian process. Additionally, we provide a new estimator of the log-determinant of a positive semi-definite matrix where the log-determinant is treated as a normalizing constant of a probability density.
更多查看译文
关键词
stochastic simulation, Variance reduction, Gaussian processes
AI 理解论文
溯源树
样例
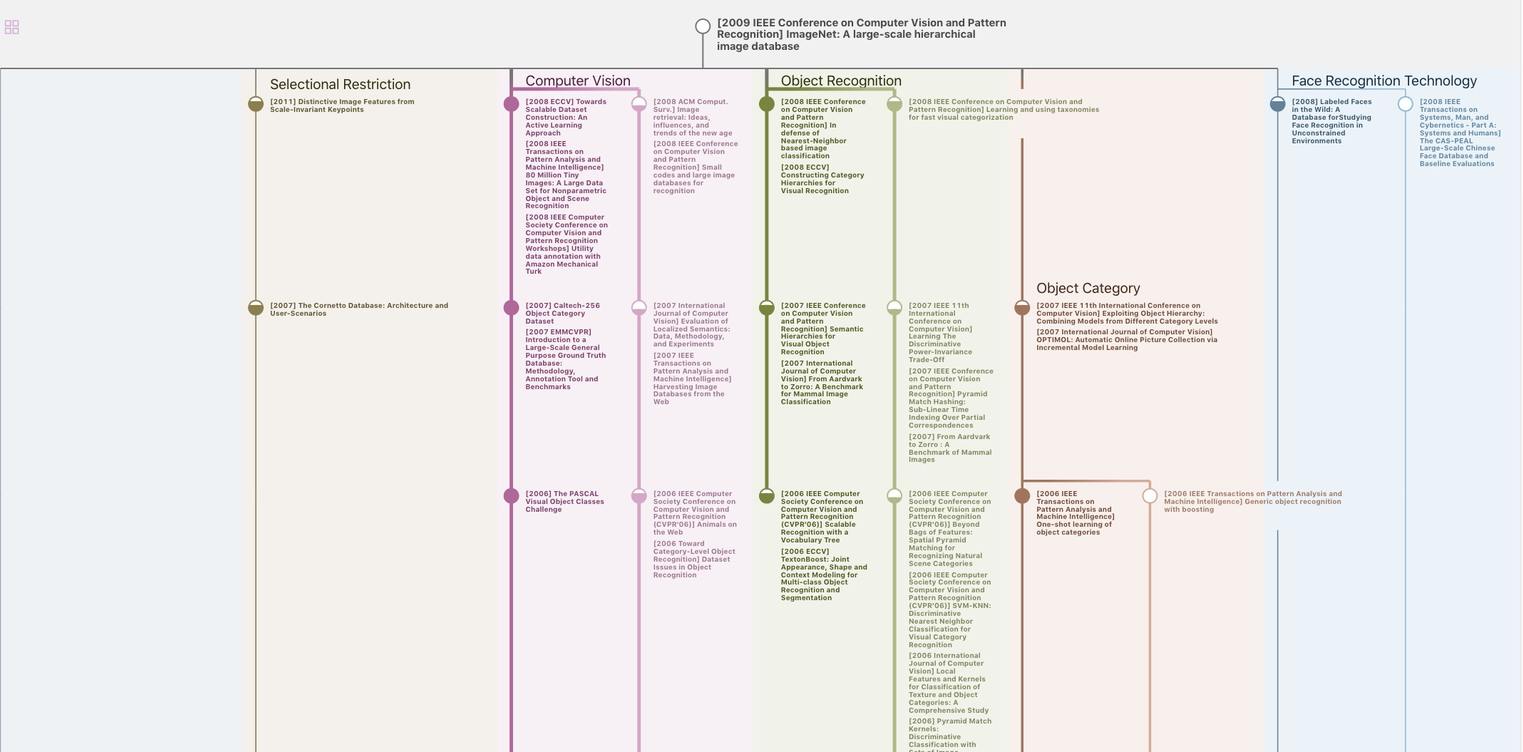
生成溯源树,研究论文发展脉络
Chat Paper
正在生成论文摘要