False Negative Reduction in Video Instance Segmentation using Uncertainty Estimates
2021 IEEE 33RD INTERNATIONAL CONFERENCE ON TOOLS WITH ARTIFICIAL INTELLIGENCE (ICTAI 2021)(2021)
摘要
Instance segmentation of images is an important tool for automated scene understanding. Neural networks are usually trained to optimize their overall performance in terms of accuracy. Meanwhile, in applications such as automated driving, an overlooked pedestrian seems more harmful than a falsely detected one. In this work, we present a false negative detection method for image sequences based on inconsistencies in time series of tracked instances given the availability of image sequences in online applications. As the number of instances can be greatly increased by this algorithm, we apply a false positive pruning using uncertainty estimates aggregated over instances. To this end, instance-wise metrics are constructed which characterize uncertainty and geometry of a given instance or are predicated on depth estimation. The proposed method serves as a post-processing step applicable to any neural network that can also be trained on single frames only. In our tests, we obtain an improved trade-off between false negative and false positive instances by our fused detection approach in comparison to the use of an ordinary score value provided by the instance segmentation network during inference.
更多查看译文
关键词
deep neural networks, instance segmentation, false negative reduction, time series, automated driving
AI 理解论文
溯源树
样例
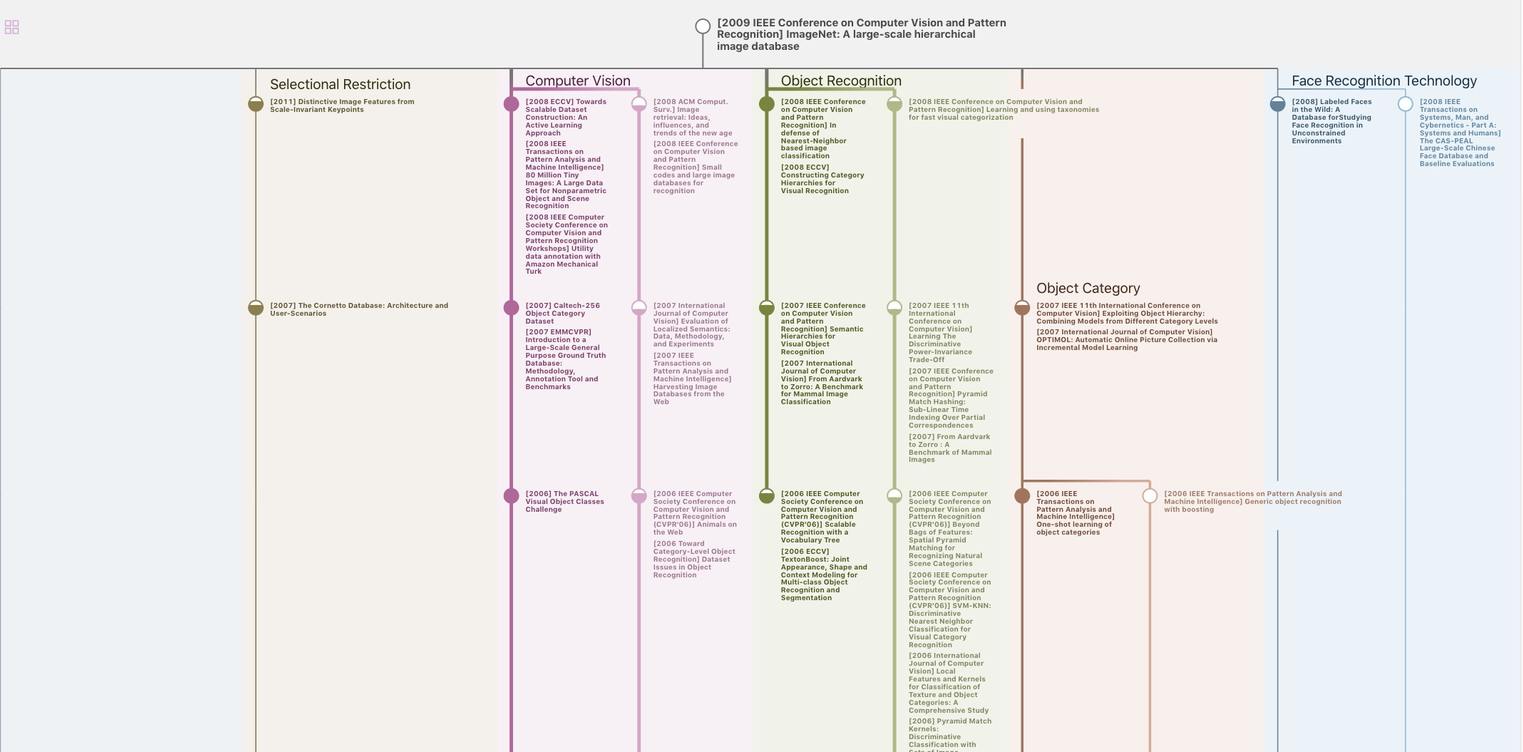
生成溯源树,研究论文发展脉络
Chat Paper
正在生成论文摘要