Knee osteoarthritis severity prediction using an attentive multi-scale deep convolutional neural network
Multimedia Tools and Applications(2024)
摘要
Knee Osteoarthritis (OA) is a destructive joint disease identified by joint stiffness, pain, and functional disability concerning millions of lives across the globe. It is generally assessed by evaluating physical symptoms, medical history, and other joint screening tests like radiographs, Magnetic Resonance Imaging (MRI), and Computed Tomography (CT) scans. Unfortunately, the conventional methods are very subjective, which forms a barrier in detecting the disease progression at an early stage. This paper presents a deep learning-based framework, namely OsteoHRNet, that automatically assesses the Knee OA severity in terms of Kellgren and Lawrence (KL) grade classification from X-rays. As a primary novelty, the proposed approach is built upon one of the most recent deep models, called the High-Resolution Network (HRNet), to capture the multi-scale features of knee X-rays. In addition, an attention mechanism has been incorporated to filter out the counterproductive features and boost the performance further. Our proposed model has achieved the best multi-class accuracy of 71.74% and MAE of 0.311 on the baseline cohort of the OAI dataset, which is a remarkable gain over the existing best-published works. Additionally, Gradient-based Class Activation Maps (Grad-CAMs) have been employed to justify the proposed network learning.
更多查看译文
关键词
Classification,Deep learning,Hrnet,Kellgren lawrence grade,Knee osteoarthritis,Knee x-ray,Osteo hrnet
AI 理解论文
溯源树
样例
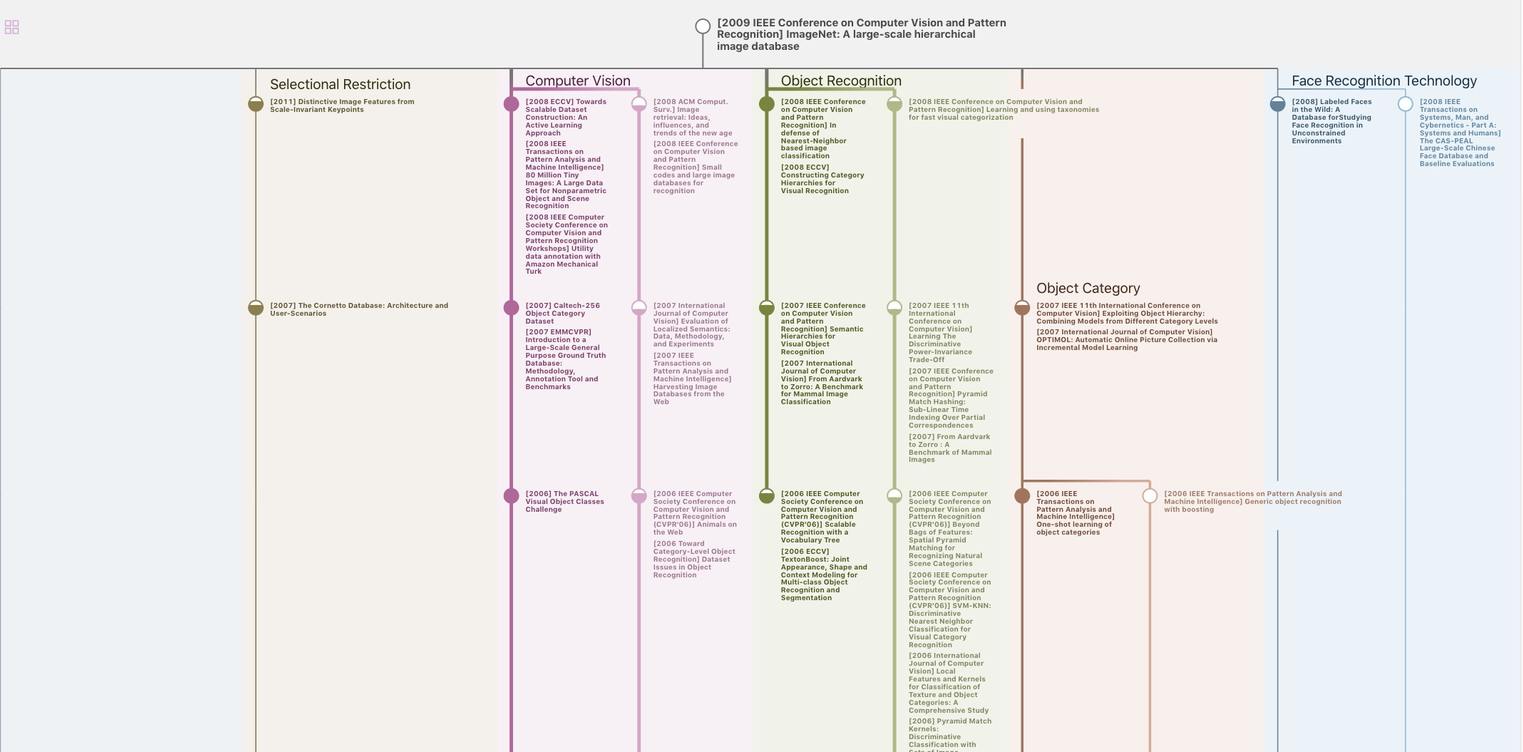
生成溯源树,研究论文发展脉络
Chat Paper
正在生成论文摘要