Transflower: probabilistic autoregressive dance generation with multimodal attention
ACM Transactions on Graphics(2021)
摘要
AbstractDance requires skillful composition of complex movements that follow rhythmic, tonal and timbral features of music. Formally, generating dance conditioned on a piece of music can be expressed as a problem of modelling a high-dimensional continuous motion signal, conditioned on an audio signal. In this work we make two contributions to tackle this problem. First, we present a novel probabilistic autoregressive architecture that models the distribution over future poses with a normalizing flow conditioned on previous poses as well as music context, using a multimodal transformer encoder. Second, we introduce the currently largest 3D dance-motion dataset, obtained with a variety of motion-capture technologies, and including both professional and casual dancers. Using this dataset, we compare our new model against two baselines, via objective metrics and a user study, and show that both the ability to model a probability distribution, as well as being able to attend over a large motion and music context are necessary to produce interesting, diverse, and realistic dance that matches the music.
更多查看译文
关键词
Generative models, machine learning, normalising flows, Glow, transformers, dance
AI 理解论文
溯源树
样例
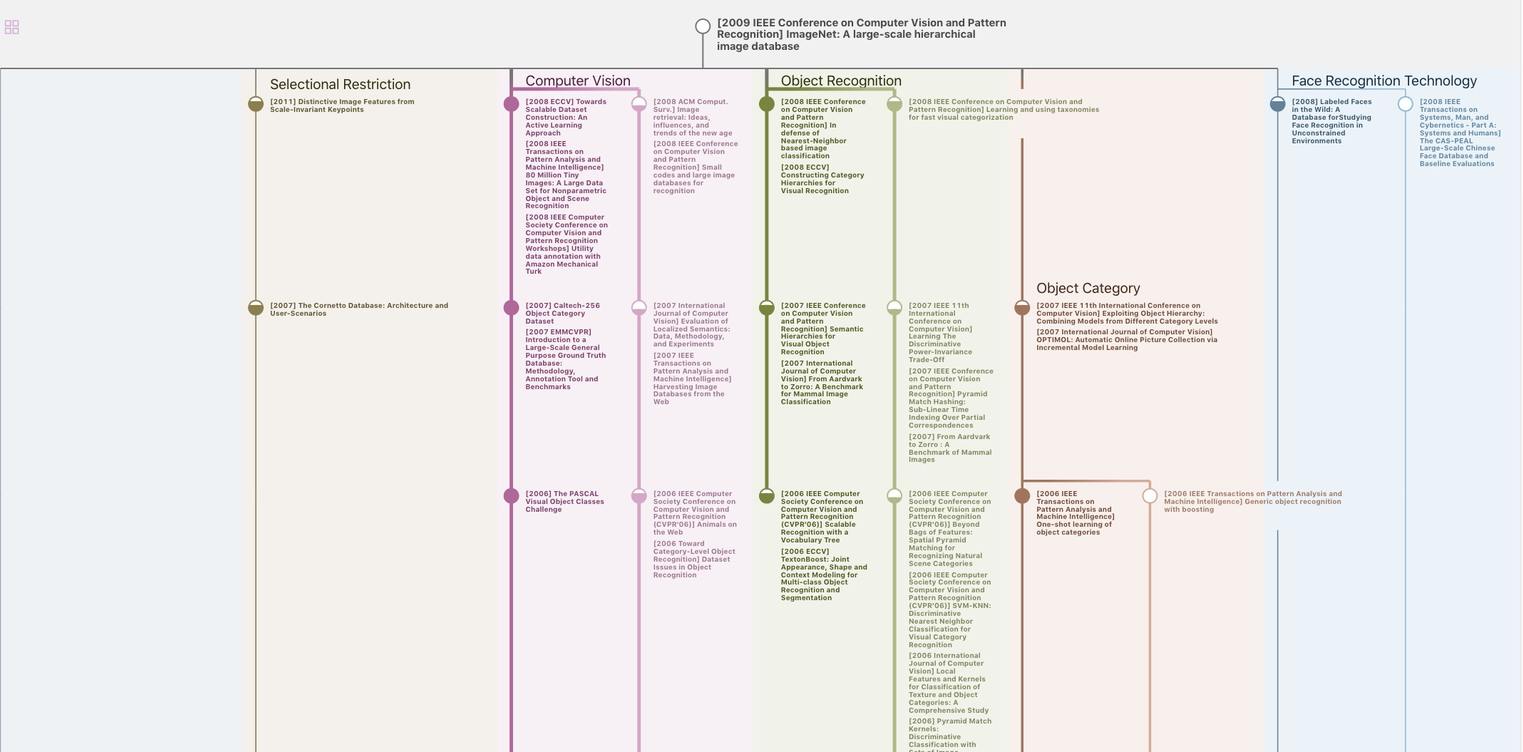
生成溯源树,研究论文发展脉络
Chat Paper
正在生成论文摘要