Accelerated model-based quantitative diffusion MRI: A feasibility study for musculoskeletal application
Zeitschrift für Medizinische Physik(2022)
摘要
Purpose
To develop a model-based reconstruction technique for diffusion quantification based on accelerated two-dimensional echo planar data, obtained with multiple b-weightings. In combination with a dedicated undersampling pattern, acceleration factors above three were proven feasible in a clinical setting.
Methods
The proposed model-based method minimizes a cost function considering the l2-norm of the difference between the Fourier transformation of a synthetic diffusion-model-generated k-space and the measured k-space data. Further regularization is performed by introduction of a total variation (TV) constraint to the cost function. Acceleration is achieved by a non-random undersampling pattern using acceleration factors that correspond to the total number of b-values. A rectangular region of variable size, centered in k-space, remains fully sampled for correction of phase variations, introduced by the different diffusion-encoding strengths.
Results
Qualitative analysis of the resulting images (S0 and ADC) demonstrates the potential of the suggested undersampling pattern in combination with a model-based iterative reconstruction. An edge analysis highlights the preservation of high-frequency information for all investigated undersampling factors. In comparison to a conventional SENSE-accelerated reconstruction, the quantitative analysis of the ADC maps revealed a significantly (P<0.05) superior performance of the suggested technique, enabling acceleration factors of R=3.65 without compromising diffusion data fidelity.
Conclusion
The presented work shows the potential of model-based ADC quantification, which, in combination with a suited undersampling pattern for multiple b-values, enables more than three-fold acceleration using two-dimensional EPI without sacrificing ADC fidelity.
更多查看译文
关键词
Diffusion,Model-based reconstruction,Iterative reconstruction,ADC
AI 理解论文
溯源树
样例
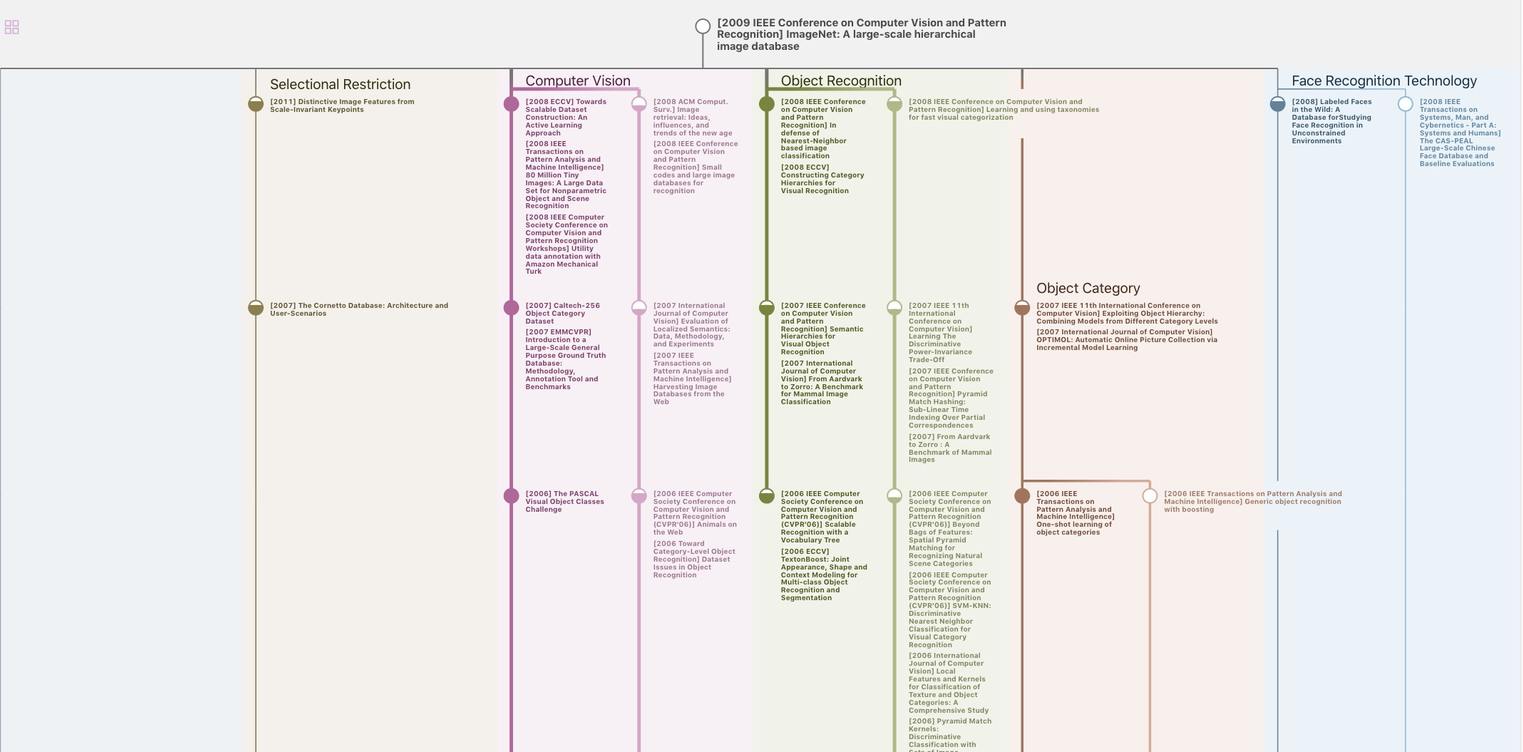
生成溯源树,研究论文发展脉络
Chat Paper
正在生成论文摘要