Task-wise Split Gradient Boosting Trees for Multi-center Diabetes Prediction
Knowledge Discovery and Data Mining(2021)
摘要
ABSTRACTDiabetes prediction is an important data science application in the social healthcare domain. There exist two main challenges in the diabetes prediction task: data heterogeneity since demographic and metabolic data are of different types, data insufficiency since the number of diabetes cases in a single medical center is usually limited. To tackle the above challenges, we employ gradient boosting decision trees (GBDT) to handle data heterogeneity and introduce multi-task learning (MTL) to solve data insufficiency. To this end, Task-wise Split Gradient Boosting Trees (TSGB) is proposed for the multi-center diabetes prediction task. Specifically, we firstly introduce task gain to evaluate each task separately during tree construction, with a theoretical analysis of GBDT's learning objective. Secondly, we reveal a problem when directly applying GBDT in MTL, i.e., the negative task gain problem. Finally, we propose a novel split method for GBDT in MTL based on the task gain statistics, named task-wise split, as an alternative to standard feature-wise split to overcome the mentioned negative task gain problem. Extensive experiments on a large-scale real-world diabetes dataset and a commonly used benchmark dataset demonstrate TSGB achieves superior performance against several state-of-the-art methods. Detailed case studies further support our analysis of negative task gain problems and provide insightful findings. The proposed TSGB method has been deployed as an online diabetes risk assessment software for early diagnosis.
更多查看译文
关键词
Multi-task Learning, Gradient Tree Boosting, Health Informatics, Diabetes Prediction
AI 理解论文
溯源树
样例
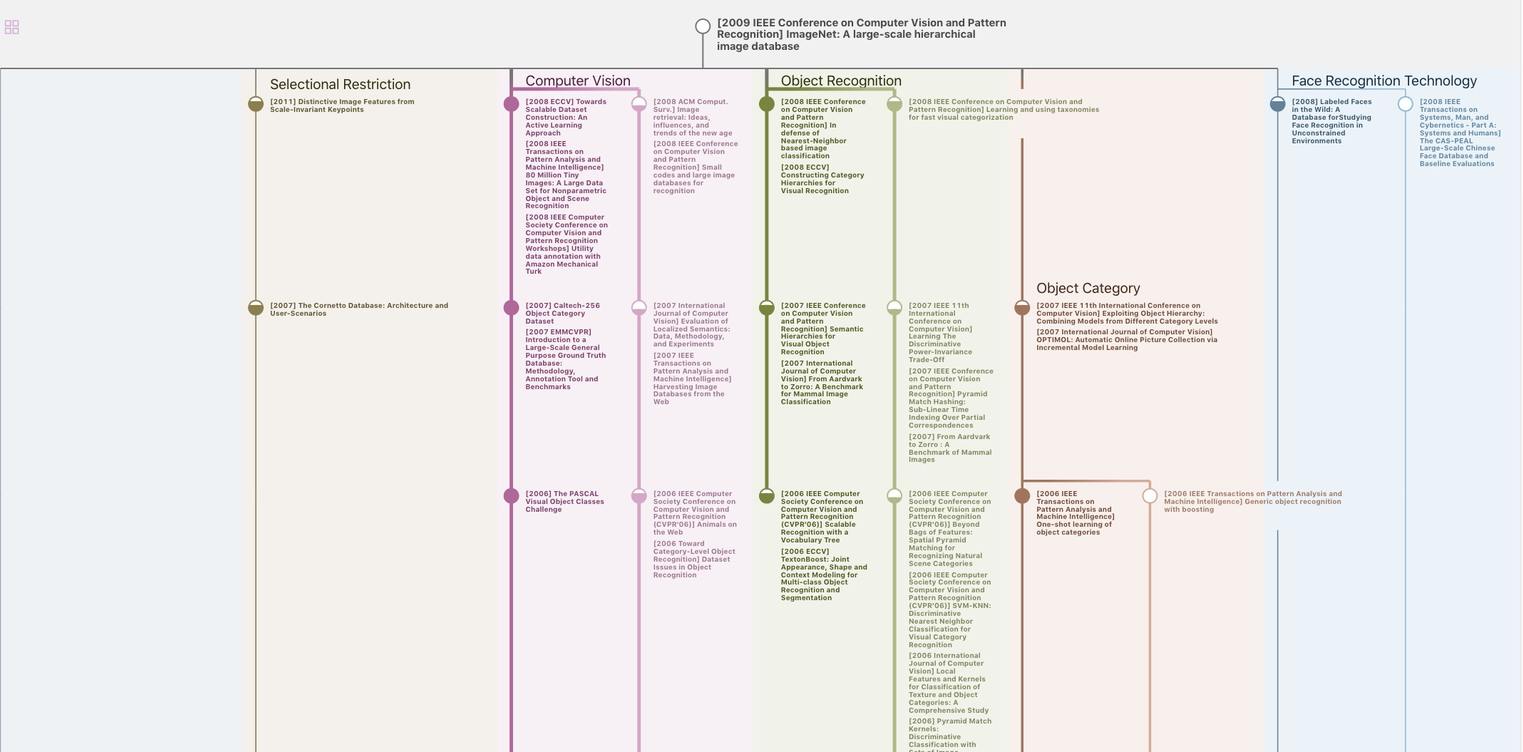
生成溯源树,研究论文发展脉络
Chat Paper
正在生成论文摘要