Supporting COVID-19 Policy Response with Large-scale Mobility-based Modeling
Knowledge Discovery and Data Mining(2021)
摘要
ABSTRACTMobility restrictions have been a primary intervention for controlling the spread of COVID-19, but they also place a significant economic burden on individuals and businesses. To balance these competing demands, policymakers need analytical tools to assess the costs and benefits of different mobility reduction measures. In this paper, we present our work motivated by our interactions with the Virginia Department of Health on a decision-support tool that utilizes large-scale data and epidemiological modeling to quantify the impact of changes in mobility on infection rates. Our model captures the spread of COVID-19 by using a fine-grained, dynamic mobility network that encodes the hourly movements of people from neighborhoods to individual places, with over 3 billion hourly edges. By perturbing the mobility network, we can simulate a wide variety of reopening plans and forecast their impact in terms of new infections and the loss in visits per sector. To deploy this model in practice, we built a robust computational infrastructure to support running millions of model realizations, and we worked with policymakers to develop an interactive dashboard that communicates our model's predictions for thousands of potential policies.
更多查看译文
关键词
Epidemiological modeling, large-scale data, policy tools
AI 理解论文
溯源树
样例
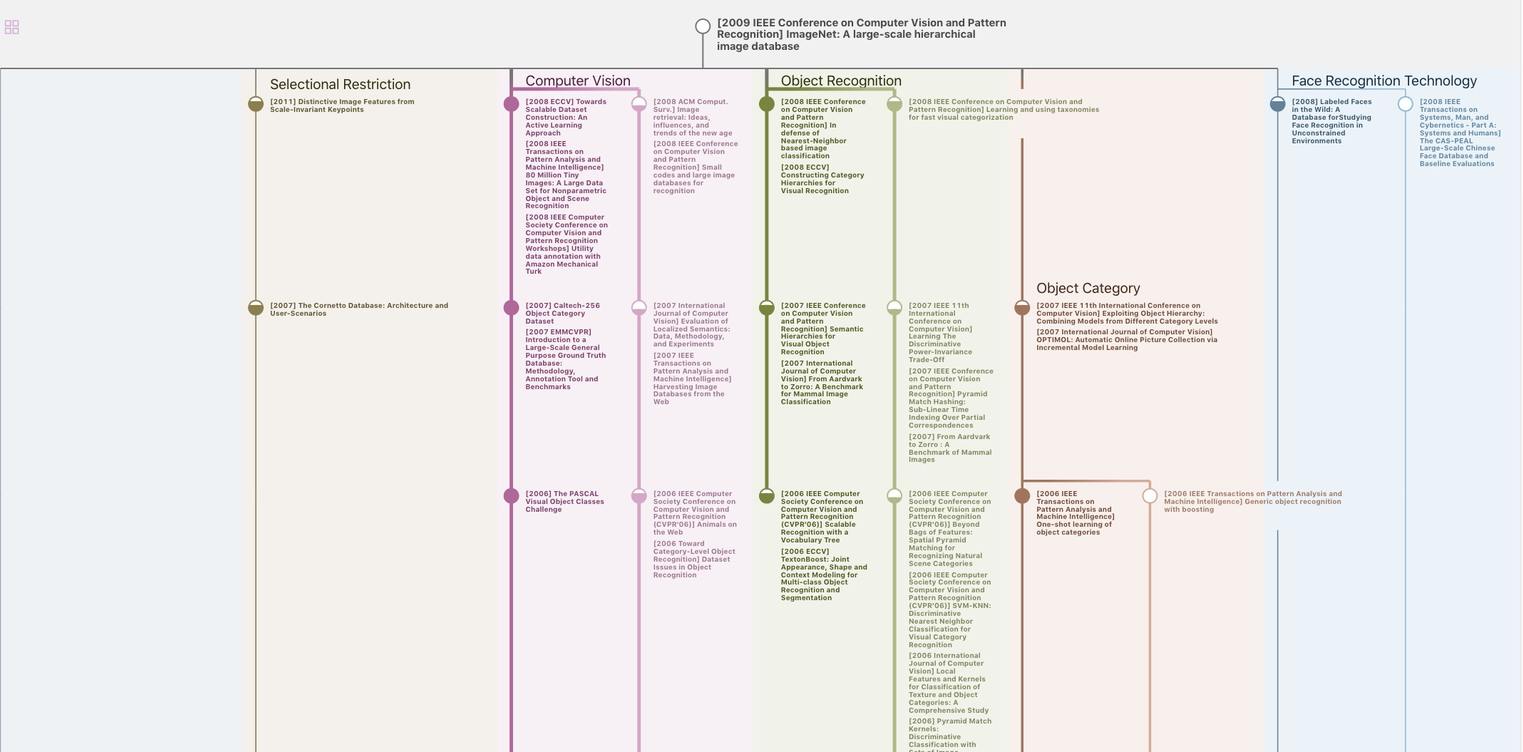
生成溯源树,研究论文发展脉络
Chat Paper
正在生成论文摘要