MEOW: A Space-Efficient Nonparametric Bid Shading Algorithm
Knowledge Discovery and Data Mining(2021)
摘要
ABSTRACTBid Shading has become increasingly important in Online Advertising, with a large amount of commercial [4,12,13,29] and research work [11,20,28] recently published. Most approaches for solving the bid shading problem involve estimating the probability of win distribution, and then maximizing surplus [28]. These generally use parametric assumptions for the distribution, and there has been some discussion as to whether Log-Normal, Gamma, Beta, or other distributions are most effective [8,38,41,44]. In this paper, we show evidence that online auctions generally diverge in interesting ways from classic distributions. In particular, real auctions generally exhibit significant structure, due to the way that humans set up campaigns and inventory floor prices [16,26]. Using these insights, we present a nonparametric method for Bid Shading which enables the exploitation of this deep structure. The algorithm has low time and space complexity, and is designed to operate within the challenging millisecond Service Level Agreements of Real-Time Bid Servers. We deploy it in one of the largest Demand Side Platforms in the United States, and show that it reliably out-performs best in class Parametric benchmarks. We conclude by suggesting some ways that the best aspects of parametric and nonparametric approaches could be combined.
更多查看译文
关键词
online bidding, shading, auction, advertising, bid, optimization
AI 理解论文
溯源树
样例
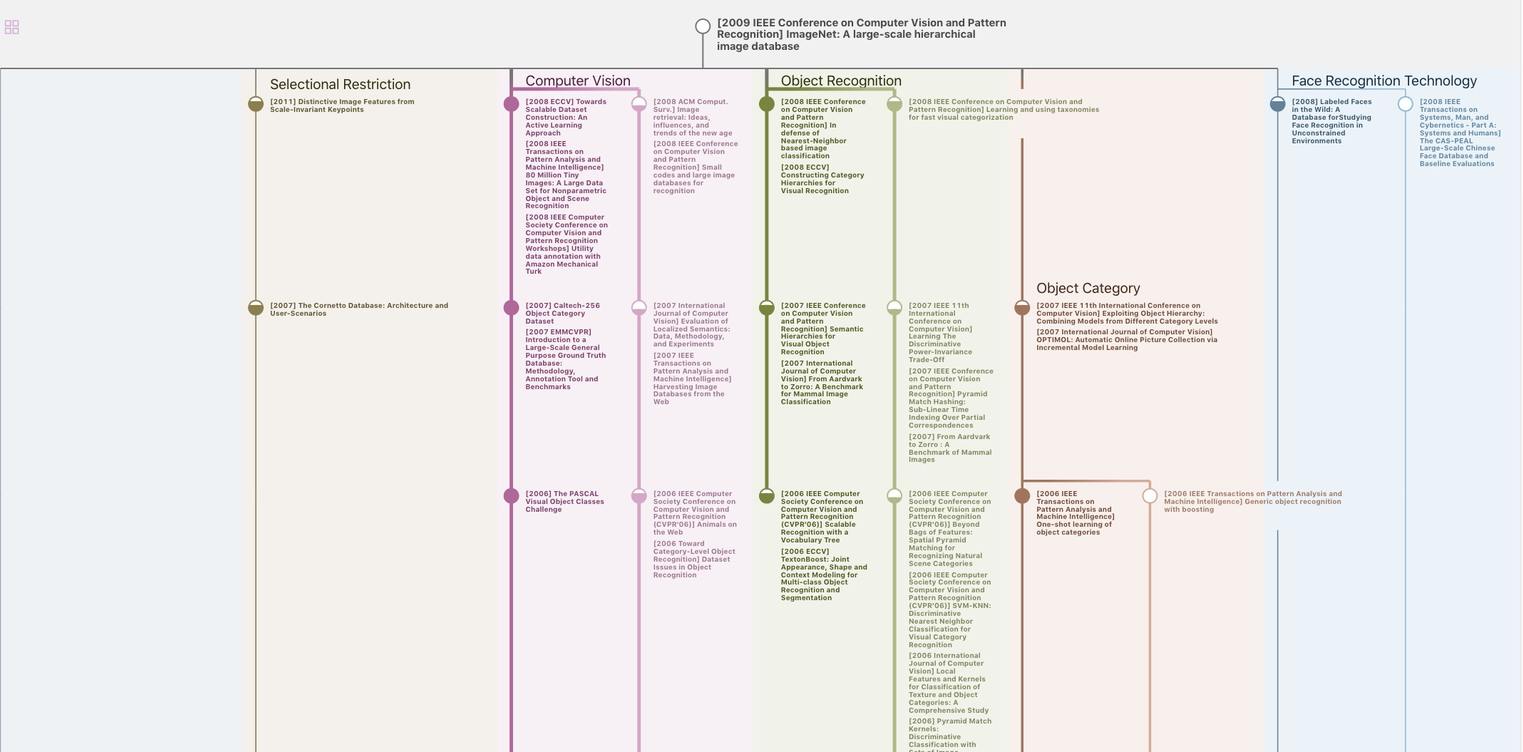
生成溯源树,研究论文发展脉络
Chat Paper
正在生成论文摘要