Debiasing Learning based Cross-domain Recommendation
Knowledge Discovery and Data Mining(2021)
摘要
ABSTRACTAs it becomes prevalent that user information exists in multiple platforms or services, cross-domain recommendation has been an important task in industry. Although it is well known that users tend to show different preferences in different domains, existing studies seldom model how domain biases affect user preferences. Focused on this issue, we develop a casual-based approach to mitigating the domain biases when transferring the user information cross domains. To be specific, this paper presents a novel debiasing learning based cross-domain recommendation framework with causal embedding. In this framework, we design a novel Inverse-Propensity-Score (IPS) estimator designed for cross-domain scenario, and further propose three kinds of restrictions for propensity score learning. Our framework can be generally applied to various recommendation algorithms for cross-domain recommendation. Extensive experiments on both public and industry datasets have demonstrated the effectiveness of the proposed framework.
更多查看译文
关键词
Cross-domain Recommendation, Debiasing Learning, Causal Embedding
AI 理解论文
溯源树
样例
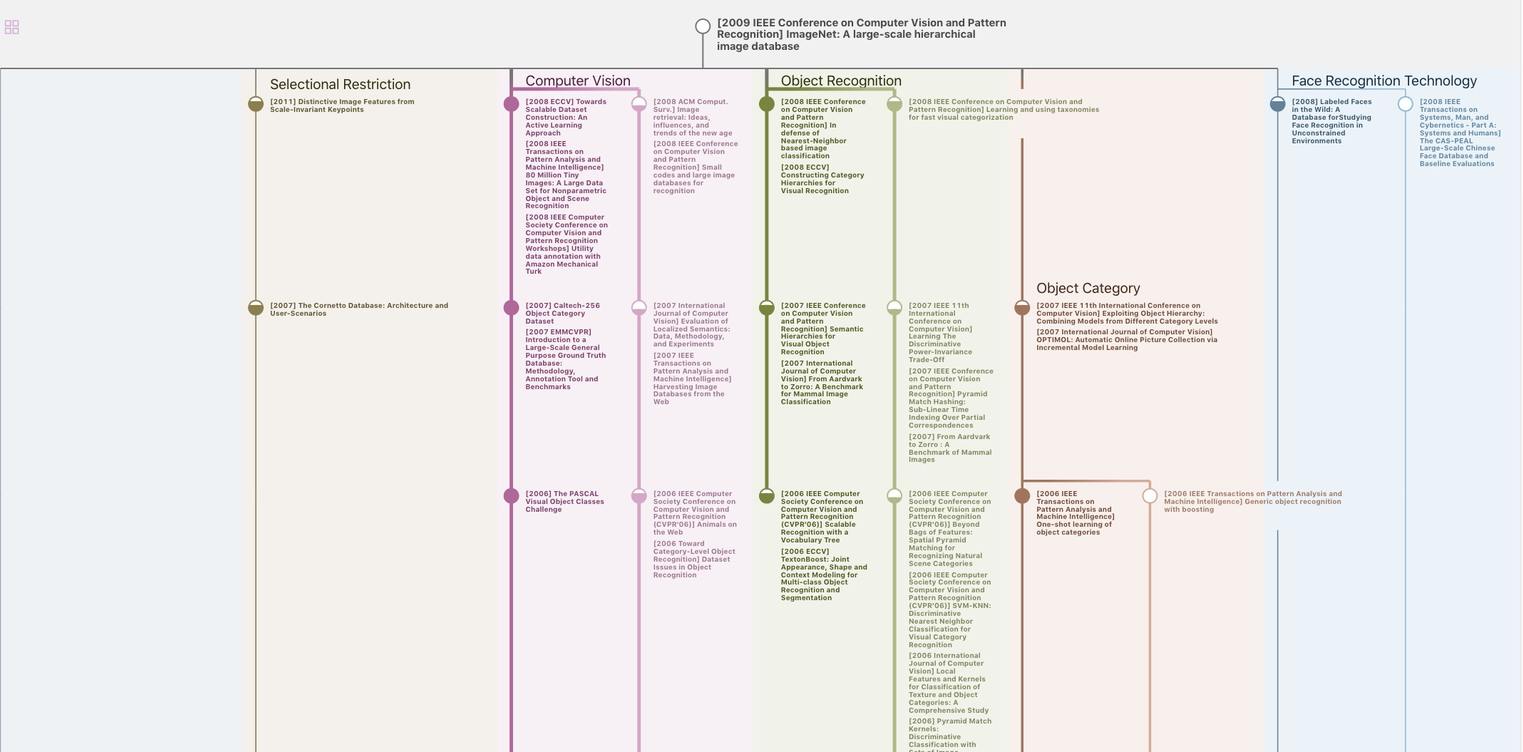
生成溯源树,研究论文发展脉络
Chat Paper
正在生成论文摘要