A Unified Solution to Constrained Bidding in Online Display Advertising
Knowledge Discovery and Data Mining(2021)
摘要
ABSTRACTIn online display advertising, advertisers usually participate in real-time bidding to acquire ad impression opportunities. In most advertising platforms, a typical impression acquiring demand of advertisers is to maximize the sum value of winning impressions under budget and some key performance indicators constraints, (e.g. maximizing clicks with the constraints of budget and cost per click upper bound). The demand can be various in value type (e.g. ad exposure/click), constraint type (e.g. cost per unit value) and constraint number. Existing works usually focus on a specific demand or hardly achieve the optimum. In this paper, we formulate the demand as a constrained bidding problem, and deduce a unified optimal bidding function on behalf of an advertiser. The optimal bidding function facilitates an advertiser calculating bids for all impressions with only m parameters, where m is the constraint number. However, in real application, it is non-trivial to determine the parameters due to the non-stationary auction environment. We further propose a reinforcement learning (RL) method to dynamically adjust parameters to achieve the optimum, whose converging efficiency is significantly boosted by the recursive optimization property in our formulation. We name the formulation and the RL method, together, as Unified Solution to Constrained Bidding (USCB). USCB is verified to be effective on industrial datasets and is deployed in Alibaba display advertising platform.
更多查看译文
关键词
Real-Time Bidding, Display Advertising, Bid Optimization
AI 理解论文
溯源树
样例
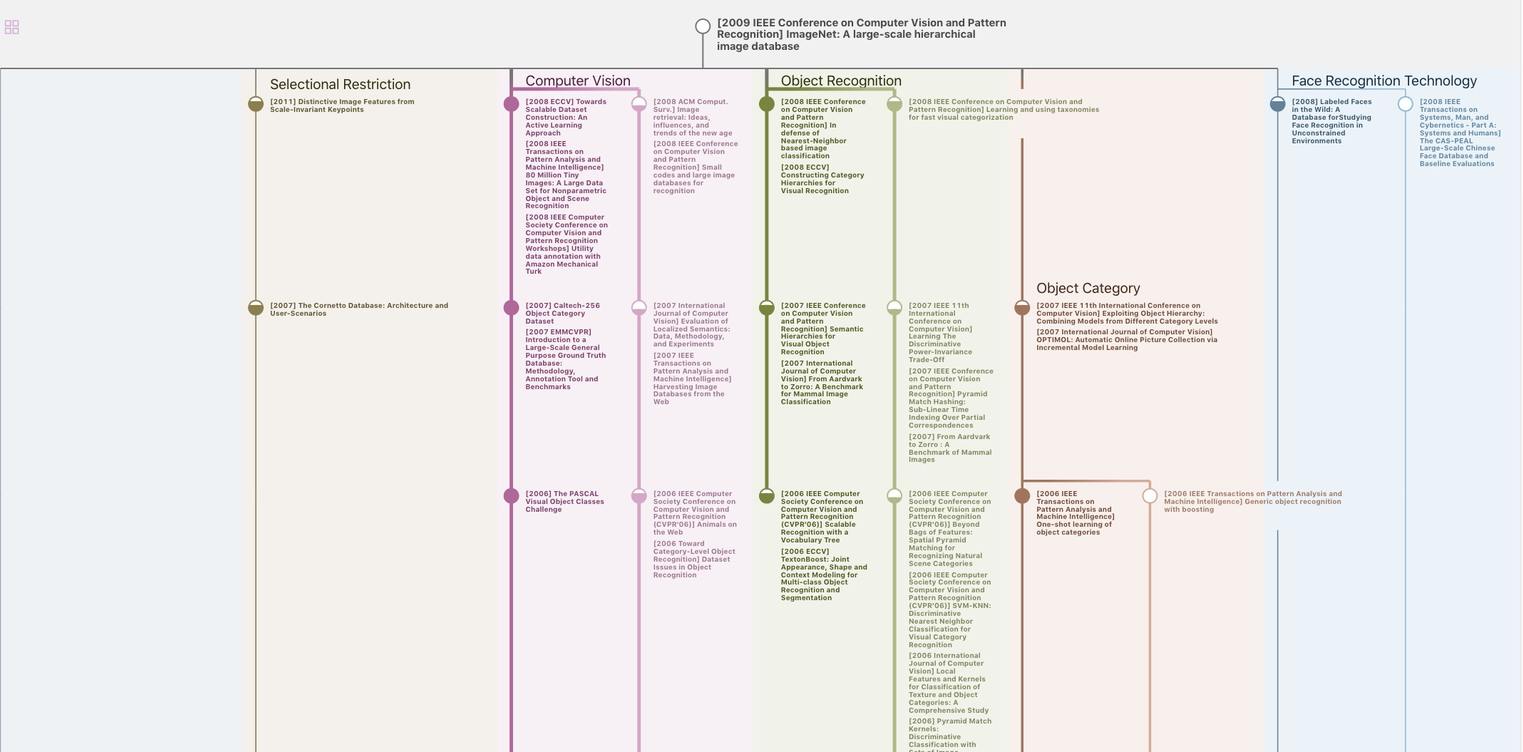
生成溯源树,研究论文发展脉络
Chat Paper
正在生成论文摘要