A PLAN for Tackling the Locust Crisis in East Africa: Harnessing Spatiotemporal Deep Models for Locust Movement Forecasting
Knowledge Discovery and Data Mining(2021)
摘要
ABSTRACTEast Africa is experiencing the worst locust infestation in over 25 years, which has severely threatened the food security of millions of people across the region. The primary strategy adopted by human experts at the United Nations Food and Agricultural Organization (UN-FAO) to tackle locust outbreaks involves manually surveying at-risk geographical areas, followed by allocating and spraying pesticides in affected regions. In order to augment and assist human experts at the UN-FAO in this task, we utilize crowdsourced reports of locust observations collected by PlantVillage (the world's leading knowledge delivery system for East African farmers) and develop PLAN, a Machine Learning (ML) algorithm for forecasting future migration patterns of locusts at high spatial and temporal resolution across East Africa. PLAN's novel spatio-temporal deep learning architecture enables representing PlantVillage's crowdsourced locust observation data using novel image-based feature representations, and its design is informed by several unique insights about this problem domain. Experimental results show that PLAN achieves superior predictive performance against several baseline models - it achieves an AUC score of 0.9 when used with a data augmentation method. PLAN represents a first step in using deep learning to assist and augment human expertise at PlantVillage (and UN-FAO) in locust prediction, and its real-world usability is currently being evaluated by domain experts (including a potential idea to use the heatmaps created by PLAN in a Kenyan TV show). The source code is available at https://github.com/maryam-tabar/PLAN.
更多查看译文
关键词
AI for Social Good, Spatio-temporal Deep Learning, Locust Crisis
AI 理解论文
溯源树
样例
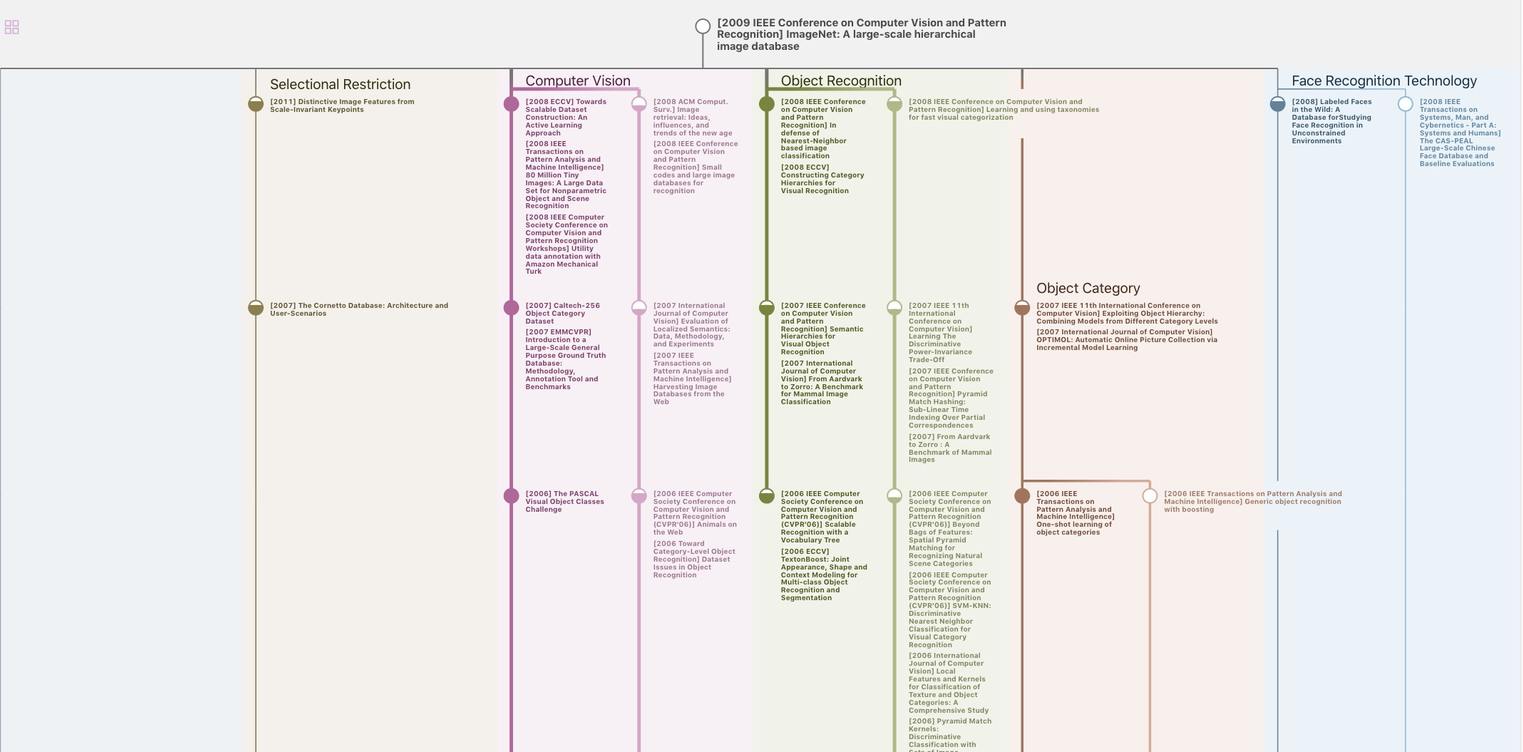
生成溯源树,研究论文发展脉络
Chat Paper
正在生成论文摘要