Uplift Modeling with Generalization Guarantees
Knowledge Discovery and Data Mining(2021)
摘要
ABSTRACTIn this paper, we consider the task of ranking individuals based on the potential benefit of being "treated" (e.g. by a drug or exposure to recommendations or ads), referred to as Uplift Modeling in the literature. This application has gained a surge of interest in recent years and it is found in many applications such as personalized medicine, recommender systems or targeted advertising. In real life scenarios the capacity of models to rank individuals by potential benefit is measured by the Area Under the Uplift Curve (AUUC), a ranking metric related to the well known Area Under ROC Curve. In the case where the objective function, for learning model parameters, is different from AUUC, the capacity of the resulting system to generalize on AUUC is limited. To tackle this issue, we propose to learn a model that directly optimizes an upper bound on AUUC. To find such a model we first develop a generalization bound on AUUC and then derive from it a learning objective called AUUC-max, usable with linear and deep models. We empirically study the tightness of this generalization bound, its effectiveness for hyperparameters tuning and show the efficiency of the proposed learning objective compared to a wide range of competitive baselines on two classical uplift modeling benchmarks using real-world datasets.
更多查看译文
关键词
uplift modeling, generalization bound, AUUC, bipartite ranking
AI 理解论文
溯源树
样例
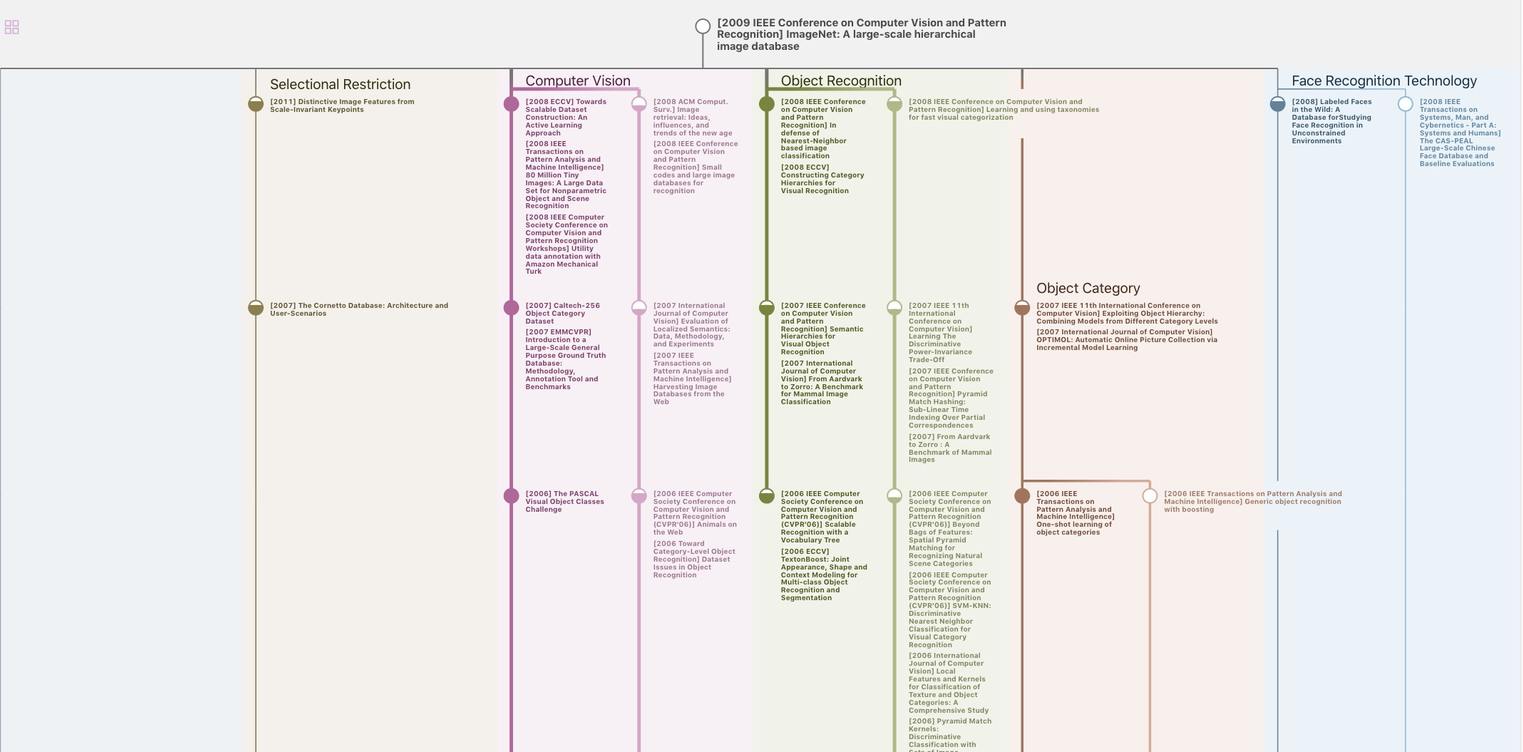
生成溯源树,研究论文发展脉络
Chat Paper
正在生成论文摘要