TopNet: Learning from Neural Topic Model to Generate Long Stories
Knowledge Discovery and Data Mining(2021)
摘要
ABSTRACTLong story generation (LSG) is one of the coveted goals in natural language processing. Different from most text generation tasks, LSG requires to output a long story of rich content based on a much shorter text input, and often suffers from information sparsity. In this paper, we propose TopNet to alleviate this problem, by leveraging the recent advances in neural topic modeling to obtain high-quality skeleton words to complement the short input. In particular, instead of directly generating a story, we first learn to map the short text input to a low-dimensional topic distribution (which is pre-assigned by a topic model). Based on this latent topic distribution, we can use the reconstruction decoder of the topic model to sample a sequence of inter-related words as a skeleton for the story. Experiments on two benchmark datasets show that our proposed framework is highly effective in skeleton word selection and significantly outperforms the state-of-the-art models in both automatic evaluation and human evaluation.
更多查看译文
关键词
Long Story Generation, Story Telling, Topic Model, Natural Language Processing, Deep Learning
AI 理解论文
溯源树
样例
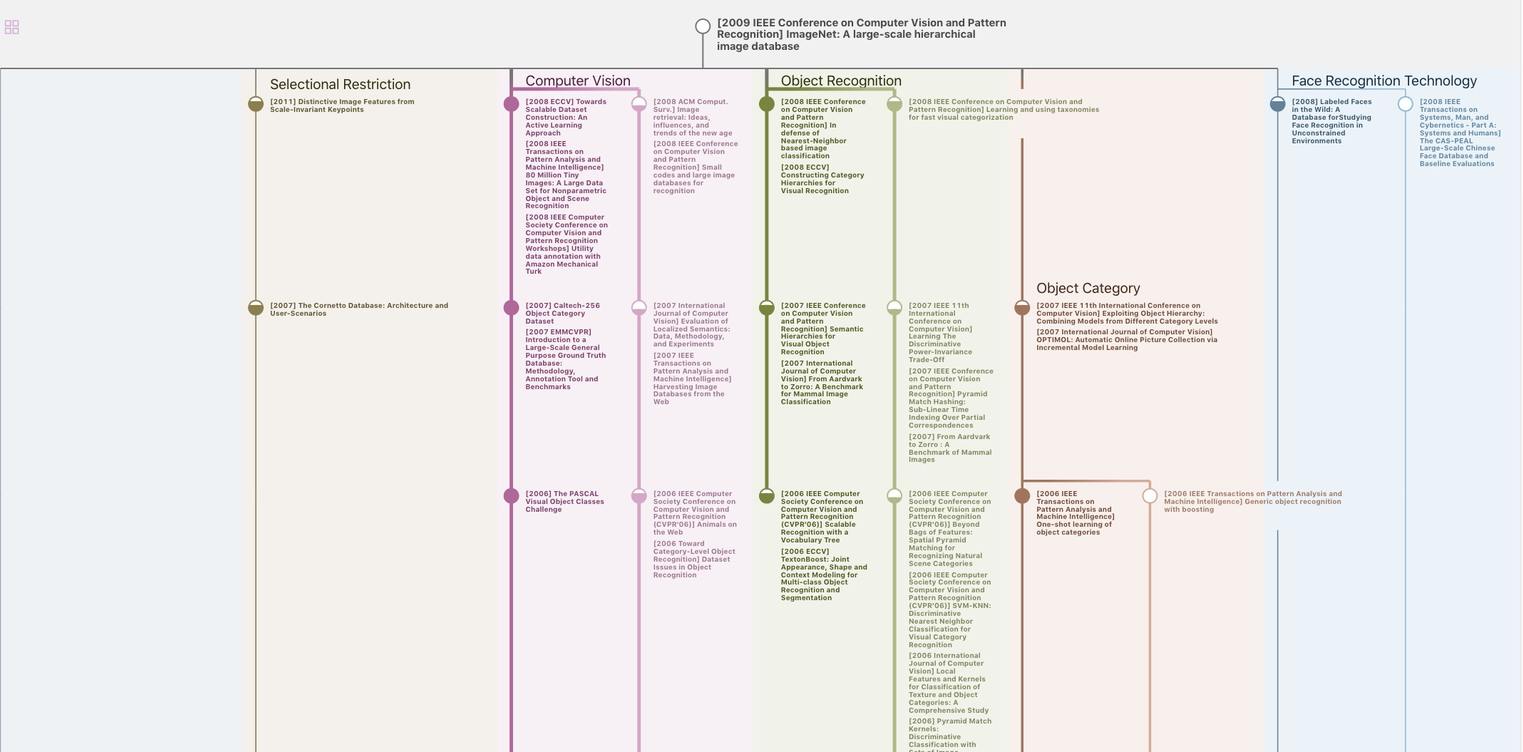
生成溯源树,研究论文发展脉络
Chat Paper
正在生成论文摘要