Joint Graph Embedding and Alignment with Spectral Pivot
Knowledge Discovery and Data Mining(2021)
摘要
ABSTRACTGraphs are powerful abstractions that naturally capture the wealth of relationships in our interconnected world. This paper proposes a new approach for graph alignment, a core problem in graph mining. Classical (e.g., spectral) methods use fixed embeddings for both graphs to perform the alignment. In contrast, the proposed approach fixes the embedding of the 'target' graph and jointly optimizes the embedding transformation and the alignment of the 'query' graph. An alternating optimization algorithm is proposed for computing high-quality approximate solutions and compared against the prevailing state-of-the-art graph aligning frameworks using benchmark real-world graphs. The results indicate that the proposed formulation can offer significant gains in terms of matching accuracy and robustness to noise relative to existing solutions for this hard but important problem.
更多查看译文
关键词
graph alignment, graph embedding, spectral methods, alternating optimization
AI 理解论文
溯源树
样例
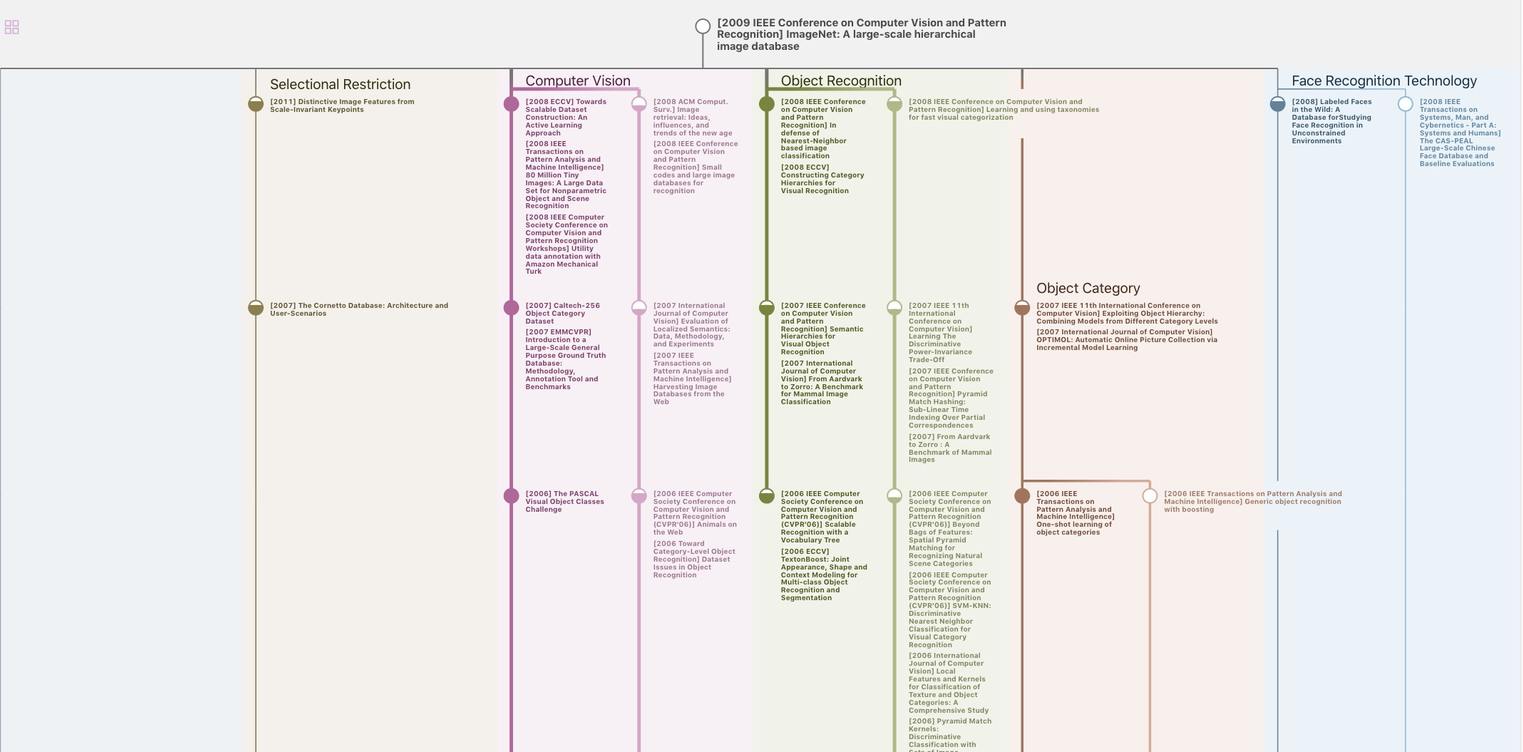
生成溯源树,研究论文发展脉络
Chat Paper
正在生成论文摘要