Energy-Efficient Models for High-Dimensional Spike Train Classification using Sparse Spiking Neural Networks
Knowledge Discovery and Data Mining(2021)
摘要
ABSTRACTSpike train classification is an important problem in many areas such as healthcare and mobile sensing, where each spike train is a high-dimensional time series of binary values. Conventional research on spike train classification mainly focus on developing Spiking Neural Networks (SNNs) under resource-sufficient settings (e.g., on GPU servers). The neurons of the SNNs are usually densely connected in each layer. However, in many real-world applications, we often need to deploy the SNN models on resource-constrained platforms (e.g., mobile devices) to analyze high-dimensional spike train data. The high resource requirement of the densely-connected SNNs can make them hard to deploy on mobile devices. In this paper, we study the problem of energy-efficient SNNs with sparsely-connected neurons. We propose an SNN model with sparse spatio-temporal coding. Our solution is based on the re-parameterization of weights in an SNN and the application of sparsity regularization during optimization. We compare our work with the state-of-the-art SNNs and demonstrate that our sparse SNNs achieve significantly better computational efficiency on both neuromorphic and standard datasets with comparable classification accuracy. Furthermore, compared with densely-connected SNNs, we show that our method has a better capability of generalization on small-size datasets through extensive experiments.
更多查看译文
关键词
spiking neural networks, supervised learning, spatio-temporal coding, sparsity, hard-concrete distribution
AI 理解论文
溯源树
样例
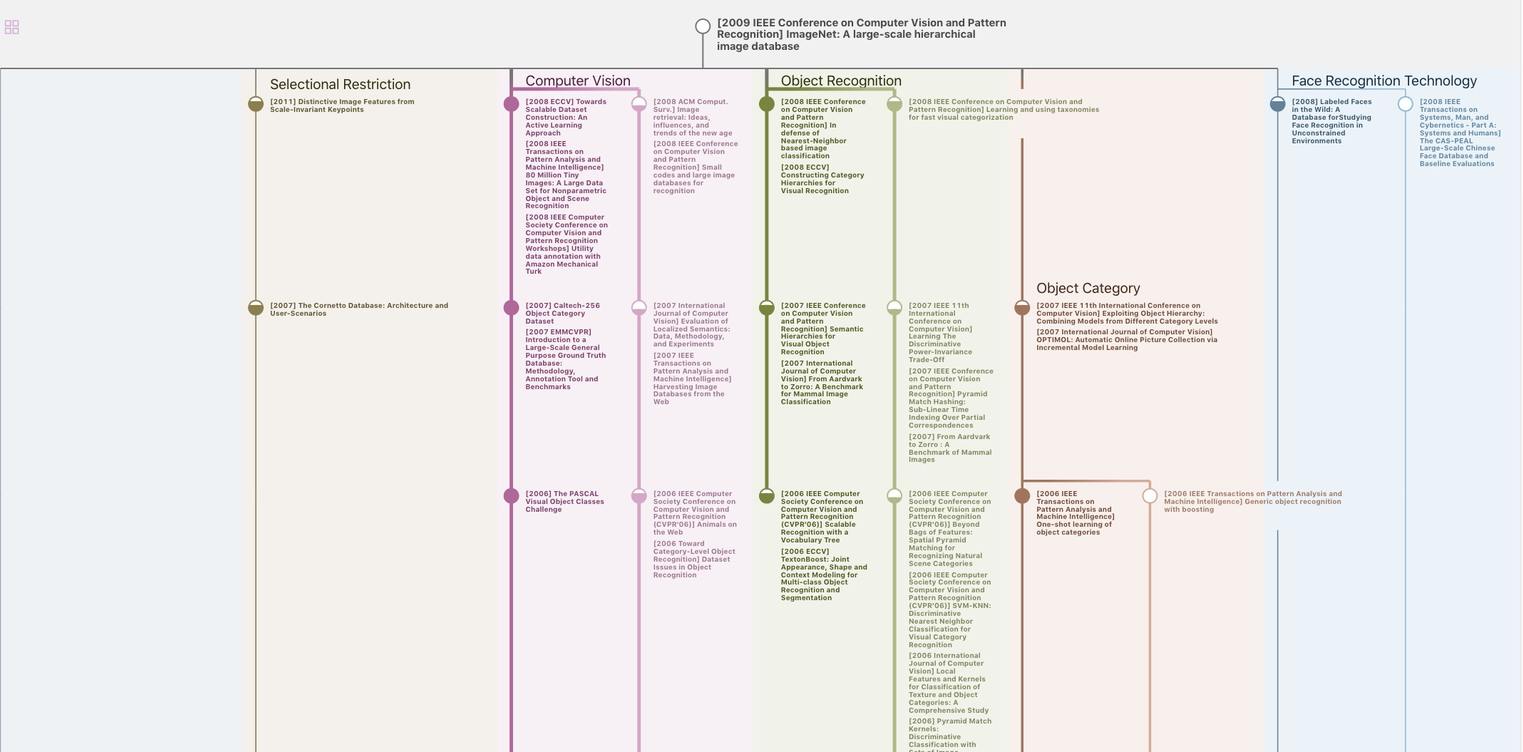
生成溯源树,研究论文发展脉络
Chat Paper
正在生成论文摘要