Gallat: A Spatiotemporal Graph Attention Network For Passenger Demand Prediction
2021 IEEE 37TH INTERNATIONAL CONFERENCE ON DATA ENGINEERING (ICDE 2021)(2021)
摘要
Online ride-hailing services have become an important component of urban transportation in recent years. As a fundamental research problem for such services, the timely prediction of passenger demands in different regions is vital for effective traffic flow control. As both spatial and temporal patterns are indispensable passenger demand prediction, relevant research has evolved from pure time series to graph-structured data for modelling historical passenger demand data, where a snapshot graph is constructed for each time slot by connecting region nodes via different relational edges. Consequently, the spatiotemporal passenger demand records naturally carry dynamic patterns in the constructed graphs, where the edges also encode important information about the directions and volume (i.e., weights) of passenger demands between two connected regions. However, existing graph-based solutions fail to simultaneously consider those three crucial aspects of dynamic, directed and weighted (DDW) graphs, leading to limited expressiveness when learning graph representations for passenger demand prediction. Therefore, we propose a novel spatiotemporal graph attention network, namely Gallat (raph prediction with all attention) as a solution. In Gallat, by comprehensively incorporating those three intrinsic properties of DDW graphs, we build three attention layers to fully capture the spatiotemporal dependencies among different regions across all historical time slots. Our experimental results on real-world datasets demonstrate that Gallat outperforms the state-of-the-art approaches.
更多查看译文
关键词
Dynamic Graph, Representation Learning, Passenger Demand Prediction
AI 理解论文
溯源树
样例
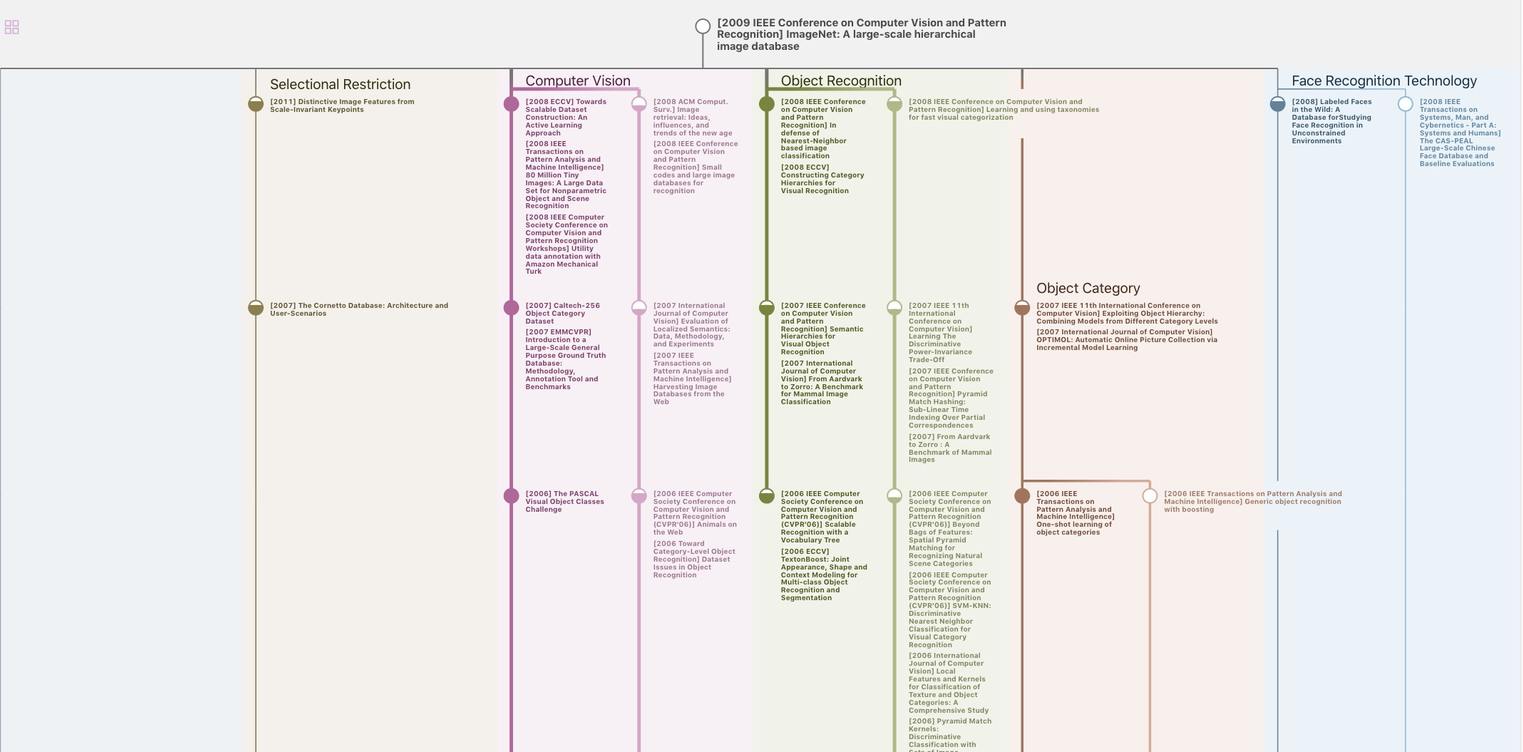
生成溯源树,研究论文发展脉络
Chat Paper
正在生成论文摘要