Explaining Missing Data In Graphs: A Constraint-Based Approach
2021 IEEE 37TH INTERNATIONAL CONFERENCE ON DATA ENGINEERING (ICDE 2021)(2021)
摘要
This paper introduces a constraint-based approach to clarify missing values in graphs. Our method capitalizes on a set Sigma of graph data constraints. An explanation is a sequence of operational enforcement of Sigma towards the recovery of interested yet missing data (e.g., attribute values, edges). We show that constraint-based approach helps us to understand not only why a value is missing, but also how to recover the missing value. We study Sigma-explanation problem, which is to compute the optimal explanations with guarantees on the informativeness and conciseness. We show the problem is in Delta(P)(2) for established graph data constraints such as graph keys and graph association rules. We develop an efficient bidirectional algorithm to compute optimal explanations, without enforcing Sigma on the entire graph. We also show our algorithm can be easily extended to support graph refinement within limited time, and to explain missing answers. Using real-world graphs, we experimentally verify the effectiveness and efficiency of our algorithms.
更多查看译文
关键词
Graphs, Data Constraints, Data Provenance
AI 理解论文
溯源树
样例
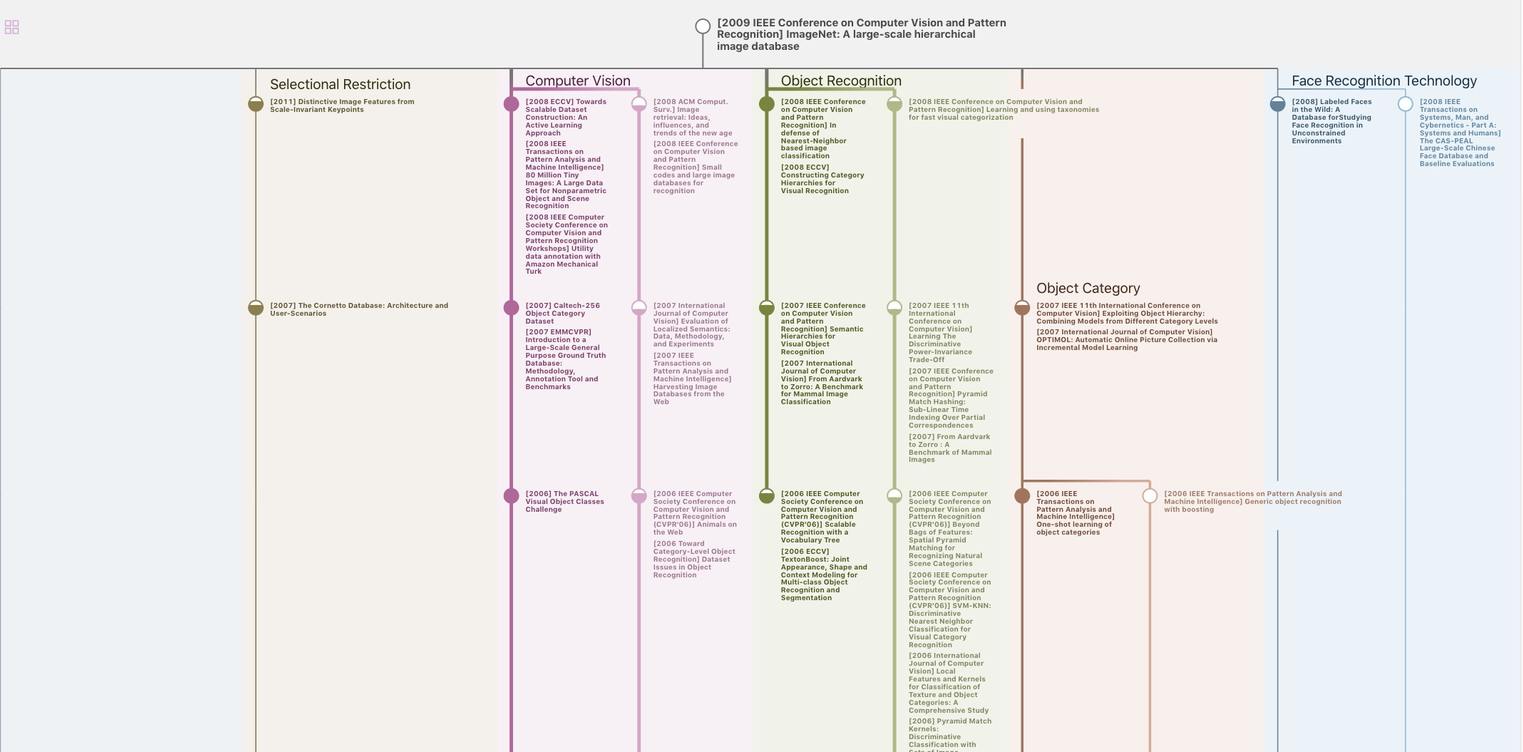
生成溯源树,研究论文发展脉络
Chat Paper
正在生成论文摘要