Scalable Graph Isomorphism: Combining Pairwise Color Refinement And Backtracking Via Compressed Candidate Space
2021 IEEE 37TH INTERNATIONAL CONFERENCE ON DATA ENGINEERING (ICDE 2021)(2021)
摘要
Graph isomorphism is a core problem in graph analysis of various application domains. Given two graphs, the graph isomorphism problem is to determine whether there exists an isomorphism between them. As real-world graphs are getting bigger and bigger, applications demand practically fast algorithms that can run on large-scale graphs. However, existing approaches such as graph canonization and subgraph isomorphism show limited performances on large-scale graphs either in time or space. In this paper, we propose a new approach to graph isomorphism, which is the framework of pairwise color refinement and efficient backtracking. The main features of our approach are: (1) pairwise color refinement and binary cell mapping (2) compressed CS (candidate space), and (3) partial failing set, which together lead to a much faster and scalable algorithm for graph isomorphism. Extensive experiments with real-world datasets show that our approach outperforms state-of-the-art algorithms by up to orders of magnitude in terms of running time.
更多查看译文
关键词
scalable graph isomorphism,compressed candidate space,graph analysis,graph isomorphism problem,real-world graphs,large-scale graphs,graph canonization,subgraph isomorphism,binary cell mapping,pairwise color refinement,backtracking,partial failing set
AI 理解论文
溯源树
样例
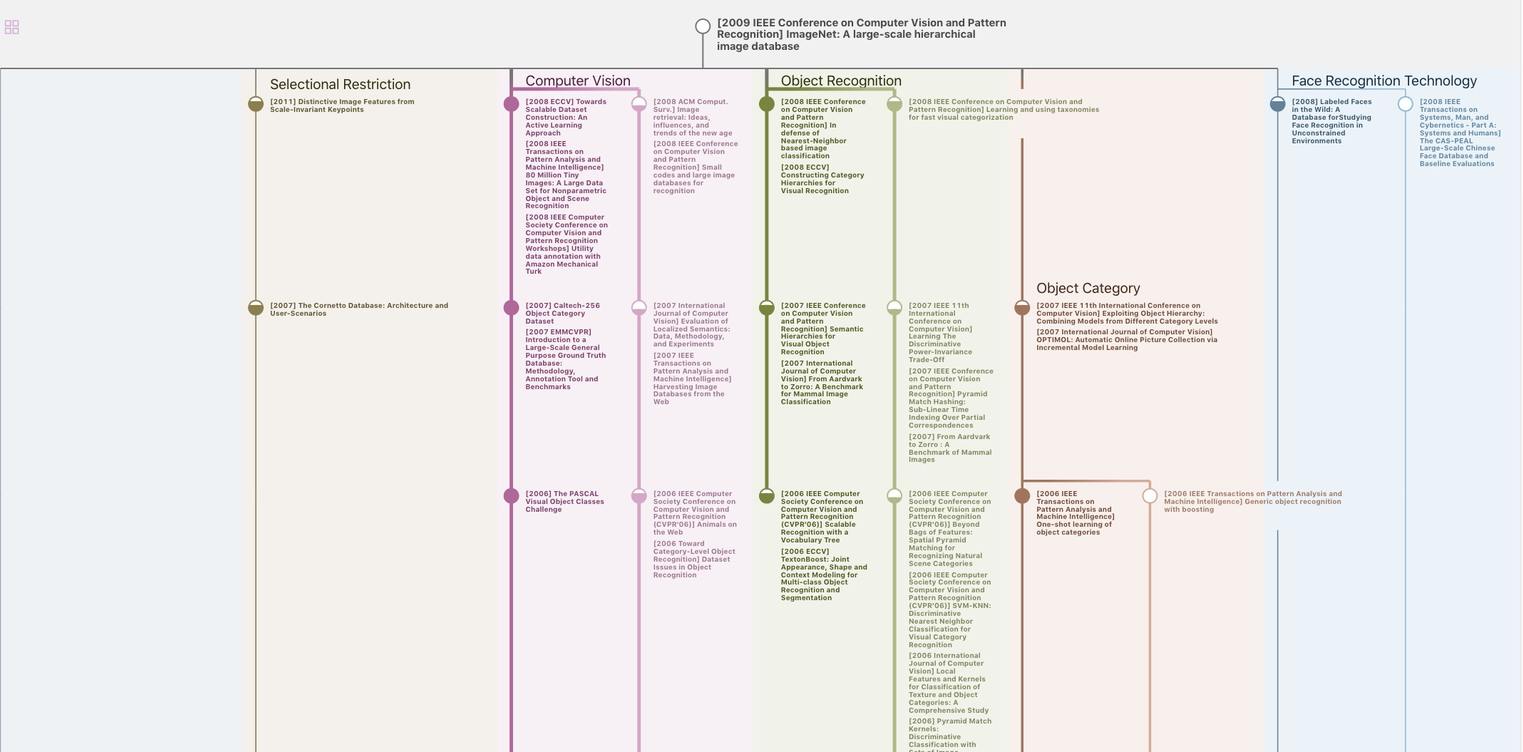
生成溯源树,研究论文发展脉络
Chat Paper
正在生成论文摘要