UniFaceGAN: A Unified Framework for Temporally Consistent Facial Video Editing
IEEE TRANSACTIONS ON IMAGE PROCESSING(2021)
摘要
Recent research has witnessed advances in facial image editing tasks including face swapping and face reenactment. However, these methods are confined to dealing with one specific task at a time. In addition, for video facial editing, previous methods either simply apply transformations frame by frame or utilize multiple frames in a concatenated or iterative fashion, which leads to noticeable visual flickers. In this paper, we propose a unified temporally consistent facial video editing framework termed UniFaceGAN. Based on a 3D reconstruction model and a simple yet efficient dynamic training sample selection mechanism, our framework is designed to handle face swapping and face reenactment simultaneously. To enforce the temporal consistency, a novel 3D temporal loss constraint is introduced based on the barycentric coordinate interpolation. Besides, we propose a region-aware conditional normalization layer to replace the traditional AdaIN or SPADE to synthesize more context-harmonious results. Compared with the state-of-the-art facial image editing methods, our framework generates video portraits that are more photo-realistic and temporally smooth.
更多查看译文
关键词
Faces, Three-dimensional displays, Training, Task analysis, Image reconstruction, Optical losses, Solid modeling, Facial video editing, dynamic training sample selection, 3D temporal loss, region-aware conditional normalization
AI 理解论文
溯源树
样例
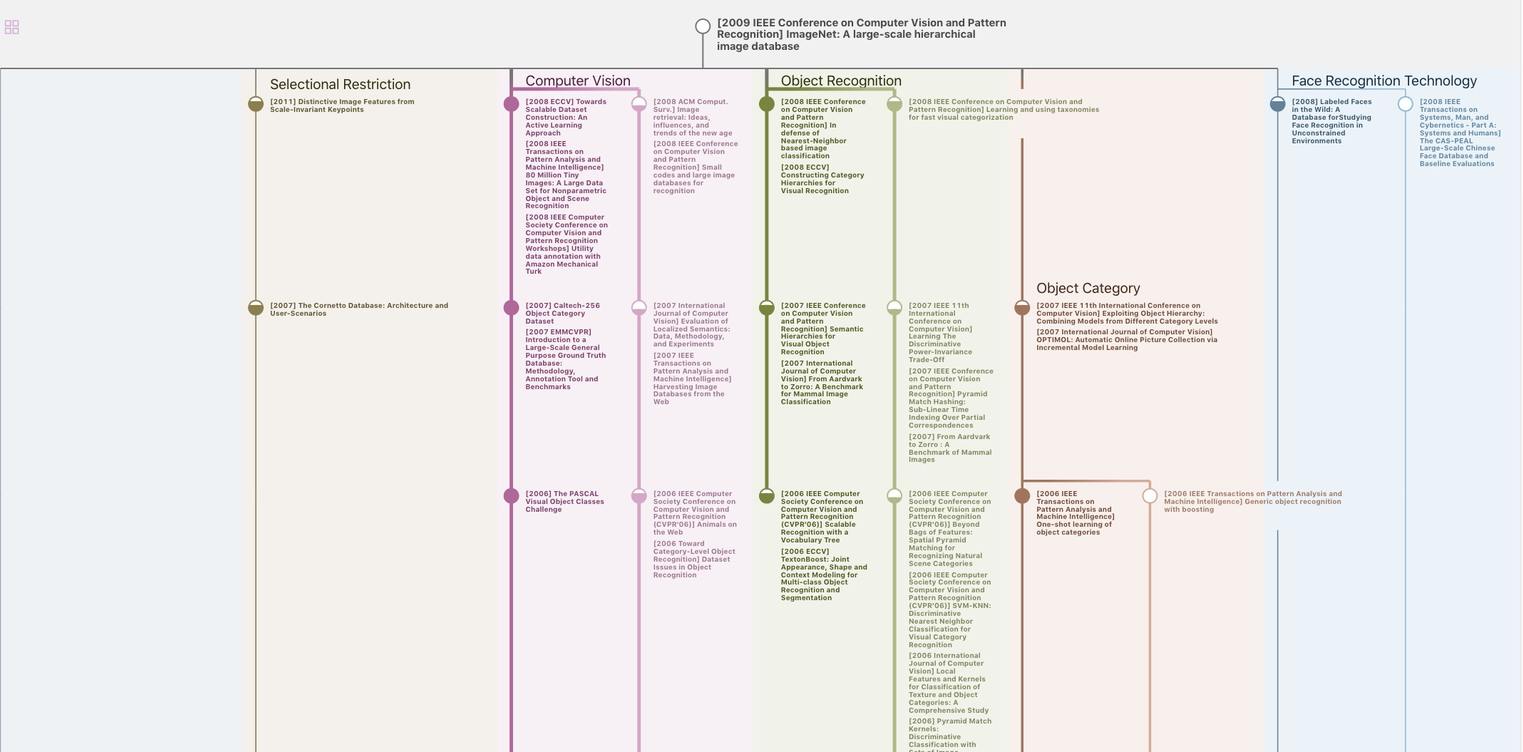
生成溯源树,研究论文发展脉络
Chat Paper
正在生成论文摘要