Adaptive scenario subset selection for min-max black-box continuous optimization
Genetic and Evolutionary Computation Conference(2021)
摘要
ABSTRACTWe handle min-max black-box optimization problems in which the scenario variable to be maximized is discrete and the design variable to be minimized is a continuous vector. To reduce the number of objective function calls, which are assumed to be computationally expensive, we propose an approach that samples a subset of scenarios at each iteration to approximate the worst-case objective function and apply the covariance matrix adaptation evolution strategy to the approximated worst-case objective function. In addition, we develop an adaptation mechanism for the probability of sampling each scenario. Moreover, we introduce the notion of support scenarios to characterize min-max optimization problems with discrete scenario variables and design test problems with various characteristics of support scenarios. Empirical evaluations reveal that the proposed approach learns to sample the set of support scenarios, being more efficient than sampling all the scenarios, especially when the available scenarios outnumber the support scenarios.
更多查看译文
关键词
Min Max Optimization, Discrete Scenarios, Adaptive Scenario Subset Selection, Covariance Matrix Adaptation Evolution Strategy (CMA-FS)
AI 理解论文
溯源树
样例
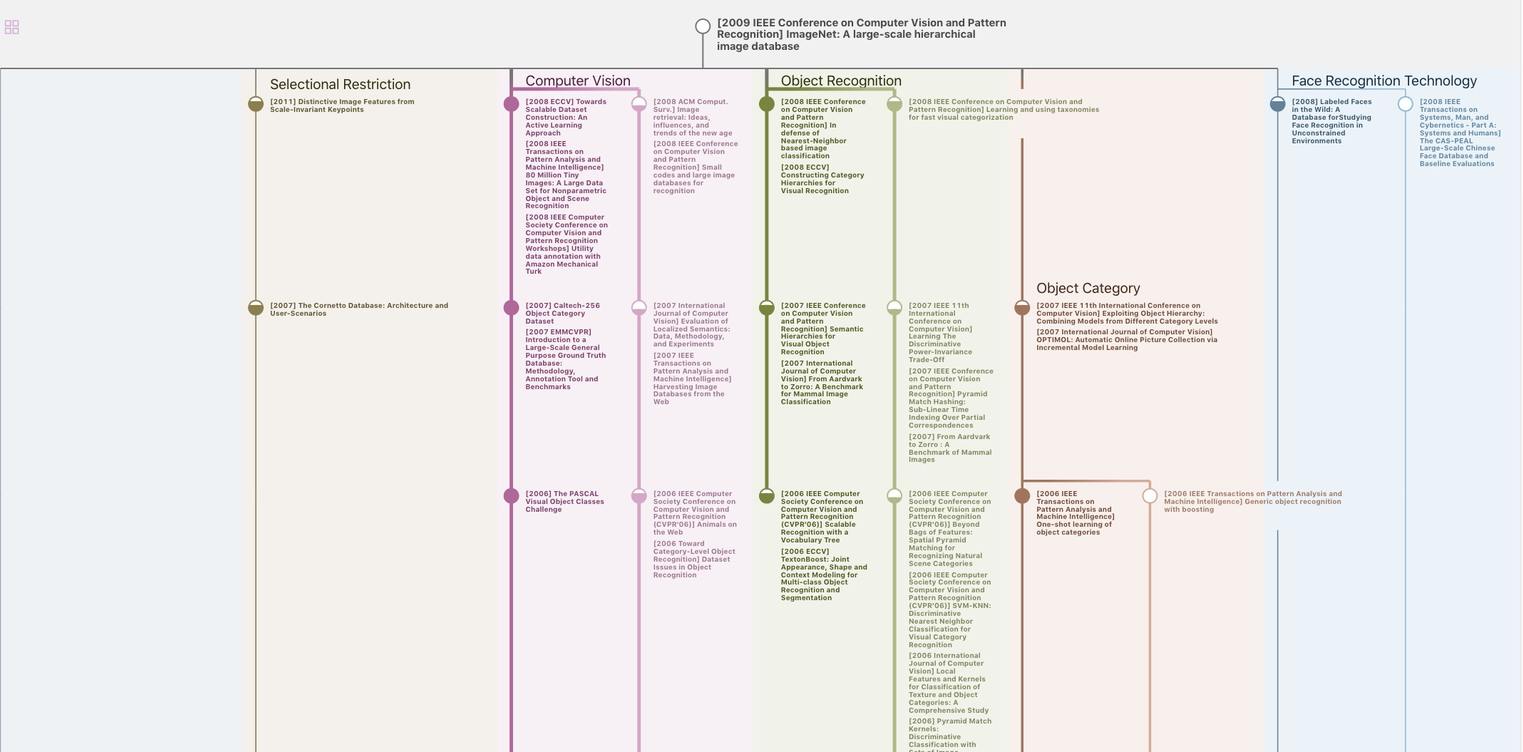
生成溯源树,研究论文发展脉络
Chat Paper
正在生成论文摘要