End-to-End Framework for Imputation and State Discovery in Longitudinal Energy Data.
e-Energy(2021)
摘要
High-resolution signals from micro-phasor measurement units (μPMU) contain crucial information about the health and status of electric equipment in power grids. In this work, we provide an end-to-end framework for fault state discovery in μPMU data. Our proposed method uses a data-driven deep learning method called Latent Ordinary Differential Equations (LatentODE) for data imputation, followed by a Bayesian non-parametric method called distance-dependent Chinese Restaurant Franchise (dd-CRF) for unsupervised discovery of latent states of the energy grid. We applied our framework to analyze one month of μPMU data and were able to correctly binned 60% of the faults within our predicted faulty time segments. We applied our framework to the task of identifying time segments when faults occur. faulty state of the time series. Our experiments show that our method produces comparably better results than traditional interpolation methods and interpretable regions for fault states in power electric systems.
更多查看译文
AI 理解论文
溯源树
样例
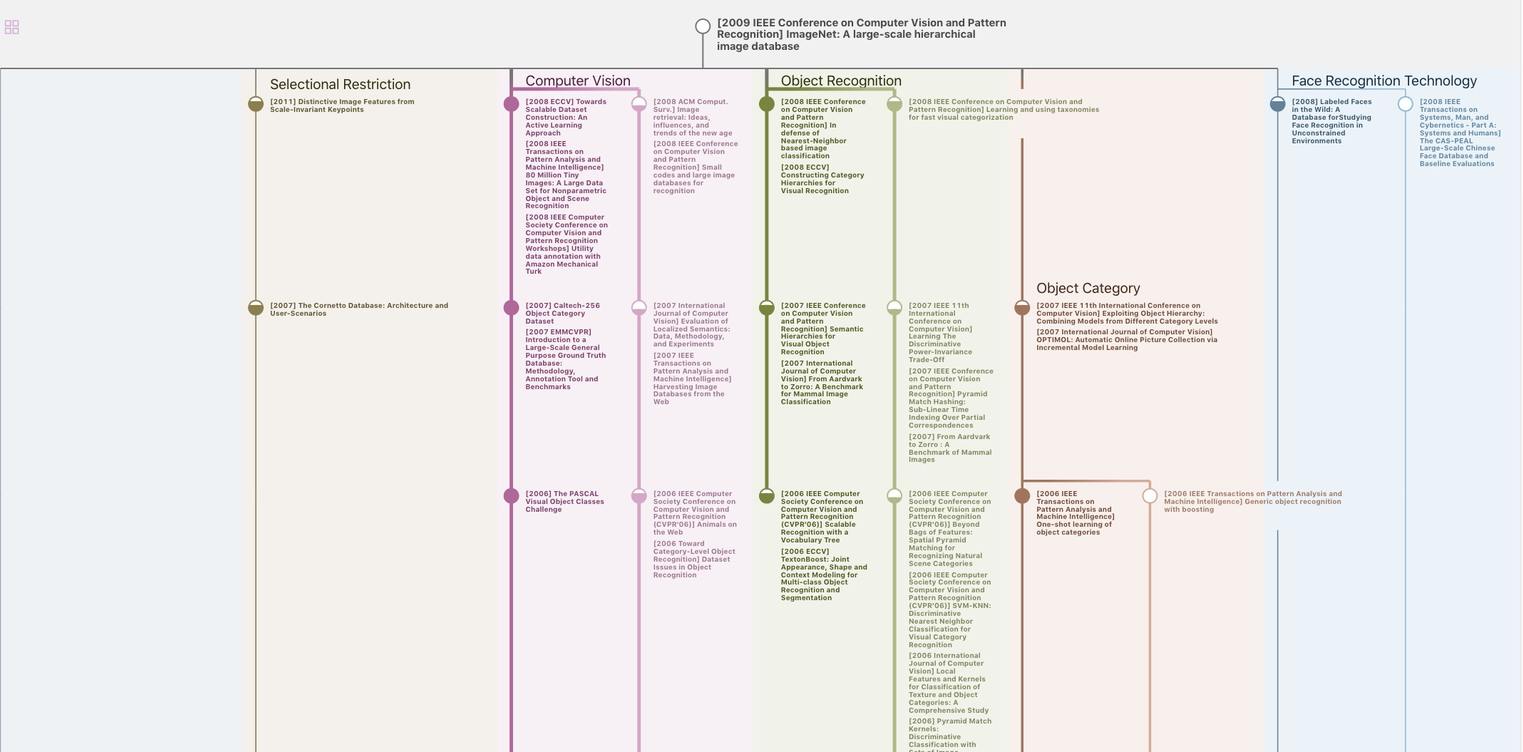
生成溯源树,研究论文发展脉络
Chat Paper
正在生成论文摘要