Task-agnostic Continual Learning with Hybrid Probabilistic Models
arxiv(2021)
摘要
Learning new tasks continuously without forgetting on a constantly changing data distribution is essential for real-world problems but extremely challenging for modern deep learning. In this work we propose HCL, a Hybrid generative-discriminative approach to Continual Learning for classification. We model the distribution of each task and each class with a normalizing flow. The flow is used to learn the data distribution, perform classification, identify task changes, and avoid forgetting, all leveraging the invertibility and exact likelihood which are uniquely enabled by the normalizing flow model. We use the generative capabilities of the flow to avoid catastrophic forgetting through generative replay and a novel functional regularization technique. For task identification, we use state-of-the-art anomaly detection techniques based on measuring the typicality of the model's statistics. We demonstrate the strong performance of HCL on a range of continual learning benchmarks such as split-MNIST, split-CIFAR, and SVHN-MNIST.
更多查看译文
关键词
learning,models,task-agnostic
AI 理解论文
溯源树
样例
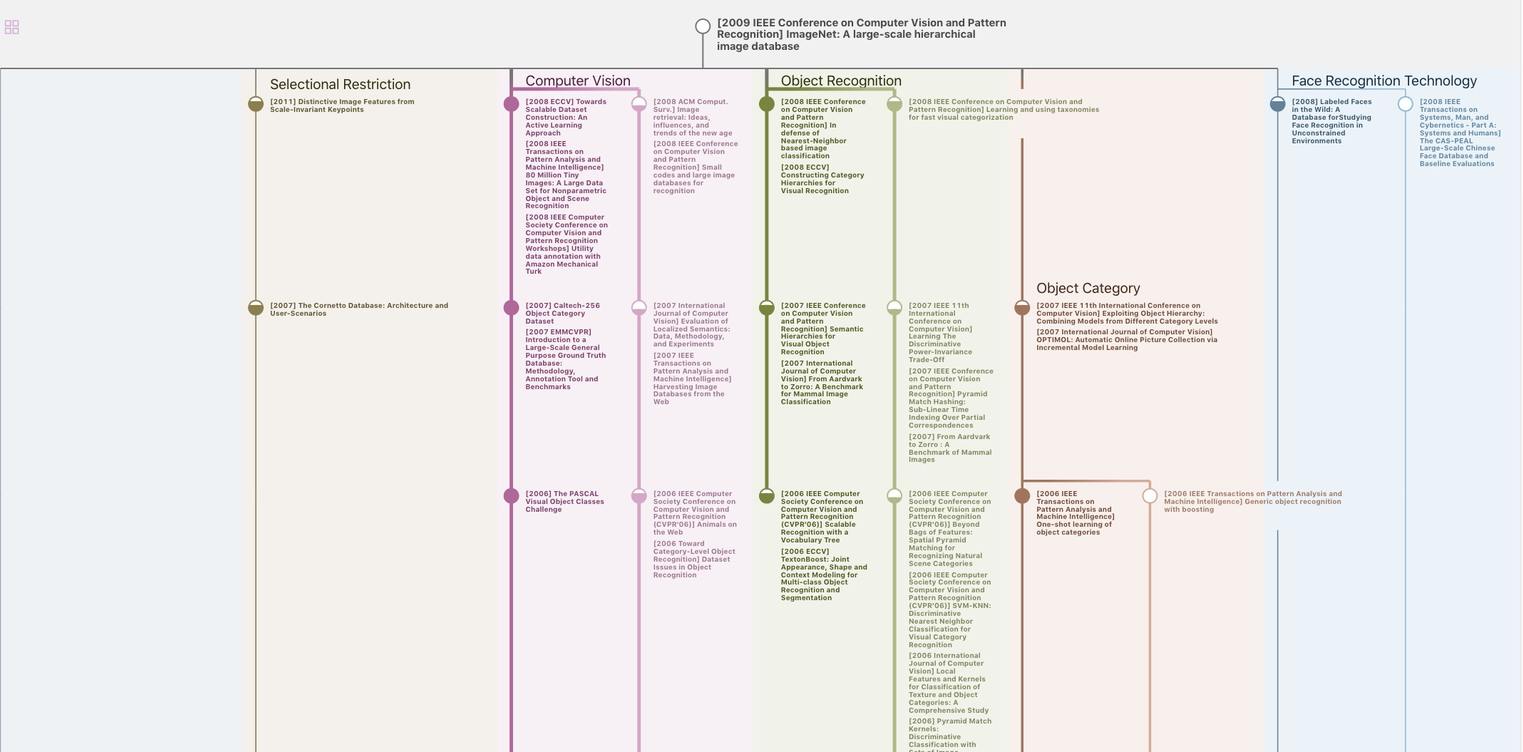
生成溯源树,研究论文发展脉络
Chat Paper
正在生成论文摘要