Leveraging semantically similar queries for ranking via combining representations
arxiv(2021)
摘要
In modern ranking problems, different and disparate representations of the items to be ranked are often available. It is sensible, then, to try to combine these representations to improve ranking. Indeed, learning to rank via combining representations is both principled and practical for learning a ranking function for a particular query. In extremely data-scarce settings, however, the amount of labeled data available for a particular query can lead to a highly variable and ineffective ranking function. One way to mitigate the effect of the small amount of data is to leverage information from semantically similar queries. Indeed, as we demonstrate in simulation settings and real data examples, when semantically similar queries are available it is possible to gainfully use them when ranking with respect to a particular query. We describe and explore this phenomenon in the context of the bias-variance trade off and apply it to the data-scarce settings of a Bing navigational graph and the Drosophila larva connectome.
更多查看译文
关键词
similar queries,ranking,representations
AI 理解论文
溯源树
样例
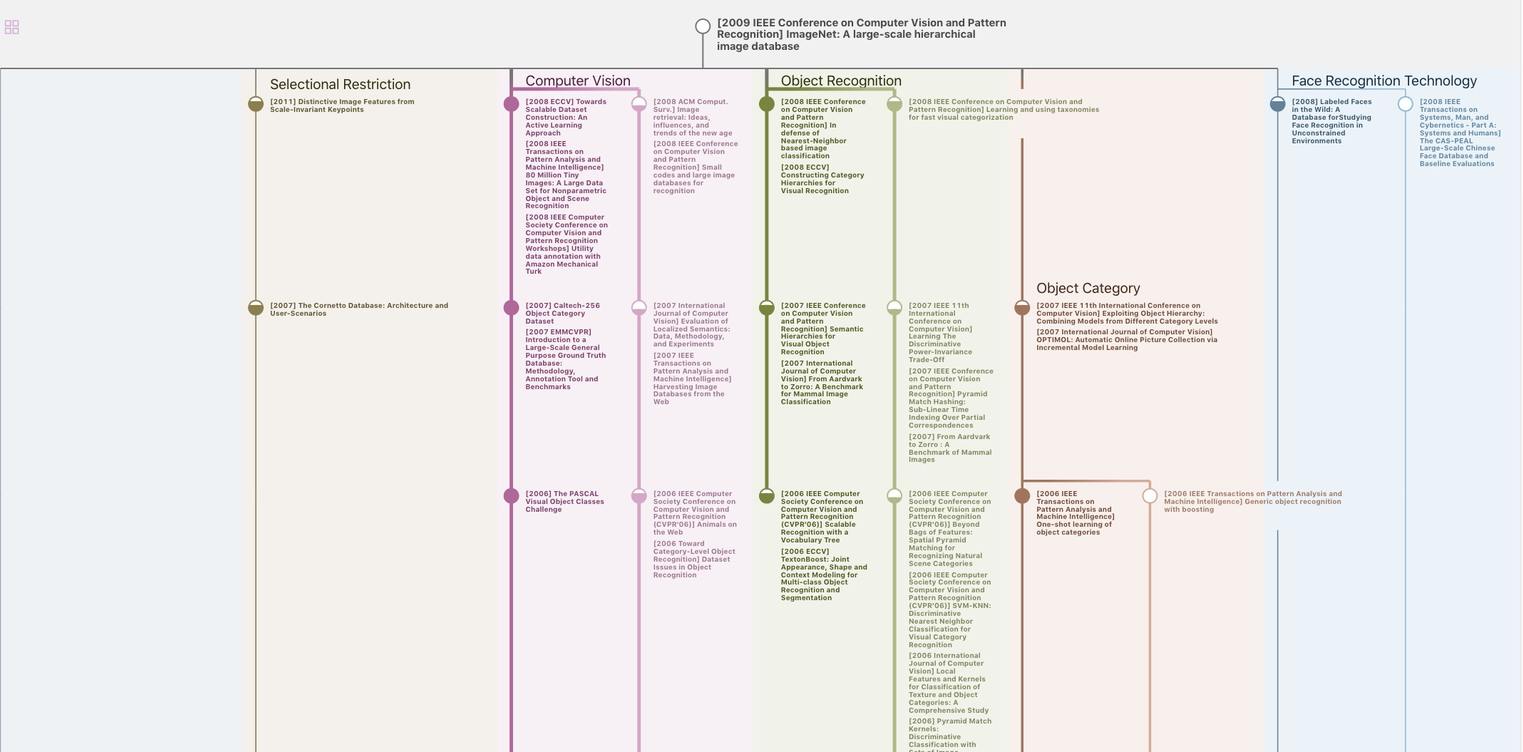
生成溯源树,研究论文发展脉络
Chat Paper
正在生成论文摘要