Understanding The Impact Of Neural Variations And Random Connections On Inference
FRONTIERS IN COMPUTATIONAL NEUROSCIENCE(2021)
摘要
Recent research suggests that in vitro neural networks created from dissociated neurons may be used for computing and performing machine learning tasks. To develop a better artificial intelligent system, a hybrid bio-silicon computer is worth exploring, but its performance is still inferior to that of a silicon-based computer. One reason may be that a living neural network has many intrinsic properties, such as random network connectivity, high network sparsity, and large neural and synaptic variability. These properties may lead to new design considerations, and existing algorithms need to be adjusted for living neural network implementation. This work investigates the impact of neural variations and random connections on inference with learning algorithms. A two-layer hybrid bio-silicon platform is constructed and a five-step design method is proposed for the fast development of living neural network algorithms. Neural variations and dynamics are verified by fitting model parameters with biological experimental results. Random connections are generated under different connection probabilities to vary network sparsity. A multi-layer perceptron algorithm is tested with biological constraints on the MNIST dataset. The results show that a reasonable inference accuracy can be achieved despite the presence of neural variations and random network connections. A new adaptive pre-processing technique is proposed to ensure good learning accuracy with different living neural network sparsity.
更多查看译文
关键词
bio-silicon computer, biological neural network, living neural network, spiking neural network, sparse connections, weight constraint, random network, recurrent network
AI 理解论文
溯源树
样例
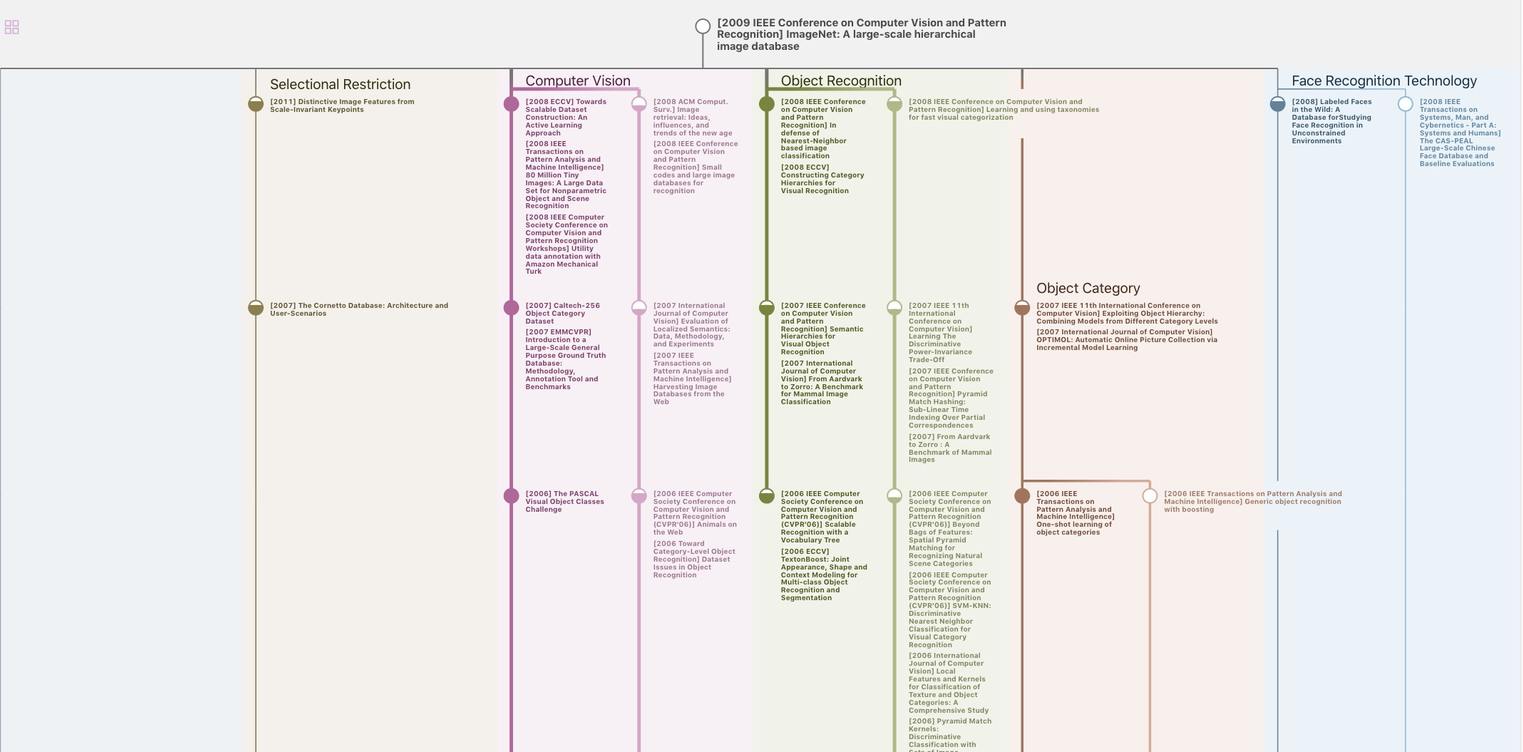
生成溯源树,研究论文发展脉络
Chat Paper
正在生成论文摘要