Not all users are the same: Providing personalized explanations for sequential decision making problems
2021 IEEE/RSJ INTERNATIONAL CONFERENCE ON INTELLIGENT ROBOTS AND SYSTEMS (IROS)(2021)
摘要
There is a growing interest in designing robots that can work alongside humans. Such robots will undoubtedly be expected to explain their behavior and decisions. While generating explanations is an actively researched topic, most works tend to focus on methods that generate explanations that are one size fits all. As in the specifics of the user-model are completely ignored. The handful of works that look at tailoring their explanation to the user’s background rely on having specific models of the users (either analytic models or learned labeling models). The goal of this work is thus to propose an end-to-end adaptive explanation generation system that begins by learning the different types of users that the robot could interact with. Then during the interaction with the target user, it is tasked with identifying the type on the fly and adjust its explanations accordingly. The former is achieved by a data-driven clustering approach while for the latter, we compile our explanation generation problem into a POMDP. We demonstrate the usefulness of our system on two domains using state-of-the-art POMDP solvers. We also report the results of a user study that investigates the benefits of providing personalized explanations in a human-robot interaction setting.
更多查看译文
关键词
analytic models,end-to-end adaptive explanation generation system,personalized explanations,human-robot interaction setting,sequential decision making problems,user-model,POMDP,data-driven clustering,learned labeling models
AI 理解论文
溯源树
样例
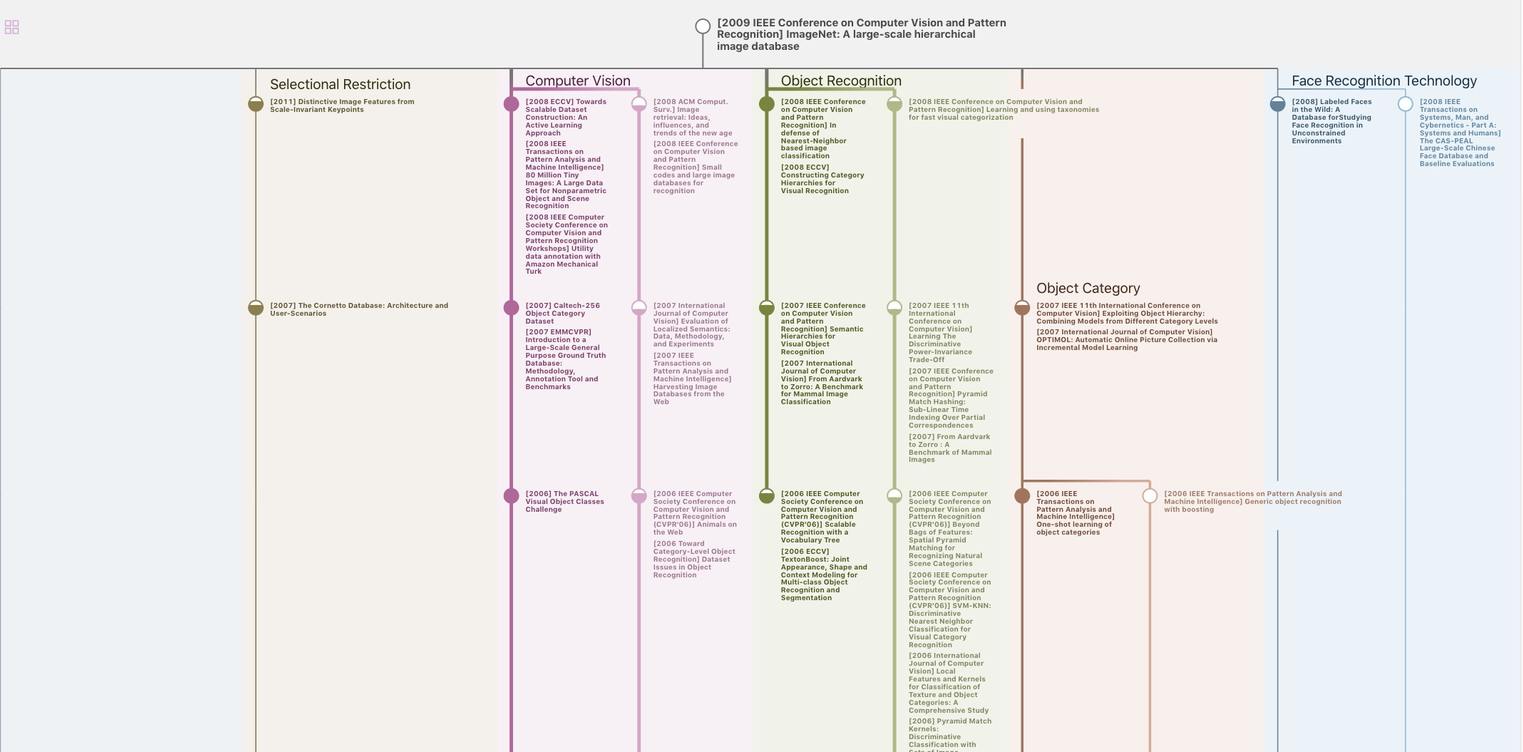
生成溯源树,研究论文发展脉络
Chat Paper
正在生成论文摘要