Adversarial Subsequences For Unconditional Text Generation
COMPUTER SPEECH AND LANGUAGE(2021)
摘要
Generative Adversarial Nets (GAN) has been successfully introduced to unconditional generating text to alleviate exposure bias. However, the discriminator in this model only evaluates the entire sequence, which causes feedback sparsity and mode collapse. To tackle these problems, we propose a novel mechanism. The mechanism first segments the entire sequence into several subsequences. Then, these subsequences, together with the entire sequence, are evaluated individually by the discriminator. Finally, these feedback signals are all used to guide the learning of GAN. This mechanism learns the generation of both the entire sequence and the subsequences simultaneously. Learning to generate subsequences is easy and is helpful in generating an entire sequence. It is easy to improve the existing GAN-based models with this mechanism. Although Li et al. (2017) segments the generated responses in a conditional text generation task, i.e., a dialogue system, they conclude it is weaker than the Monte Carlo search. However, for unconditional text generation, we observe that adversarial learning on subsequences works well. We rebuild three previous models with our mechanism, and the experimental results on two benchmark datasets show these models are improved greatly and outperform the state-of-the-art model.(c) 2021 Elsevier Ltd. All rights reserved.
更多查看译文
关键词
Unconditional text generation, GAN, Subsequences
AI 理解论文
溯源树
样例
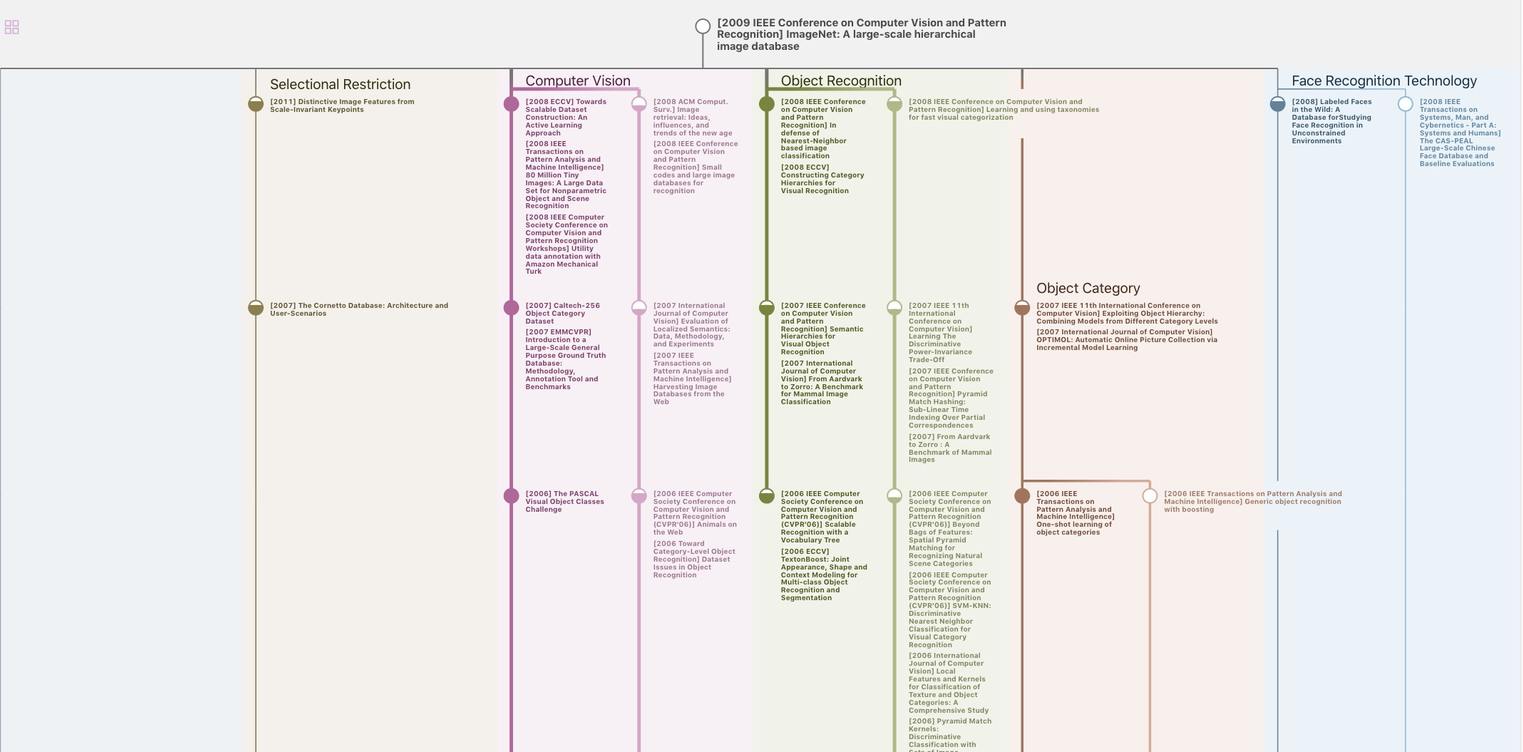
生成溯源树,研究论文发展脉络
Chat Paper
正在生成论文摘要