On Constrained Optimization in Differentiable Neural Architecture Search
arxiv(2021)
摘要
Differentiable Architecture Search (DARTS) is a recently proposed neural architecture search (NAS) method based on a differentiable relaxation. Due to its success, numerous variants analyzing and improving parts of the DARTS framework have recently been proposed. By considering the problem as a constrained bilevel optimization, we propose and analyze three improvements to architectural weight competition, update scheduling, and regularization towards discretization. First, we introduce a new approach to the activation of architecture weights, which prevents confounding competition within an edge and allows for fair comparison across edges to aid in discretization. Next, we propose a dynamic schedule based on per-minibatch network information to make architecture updates more informed. Finally, we consider two regularizations, based on proximity to discretization and the Alternating Directions Method of Multipliers (ADMM) algorithm, to promote early discretization. Our results show that this new activation scheme reduces final architecture size and the regularizations improve reliability in search results while maintaining comparable performance to state-of-the-art in NAS, especially when used with our new dynamic informed schedule.
更多查看译文
关键词
differentiable neural architecture search,constrained optimization
AI 理解论文
溯源树
样例
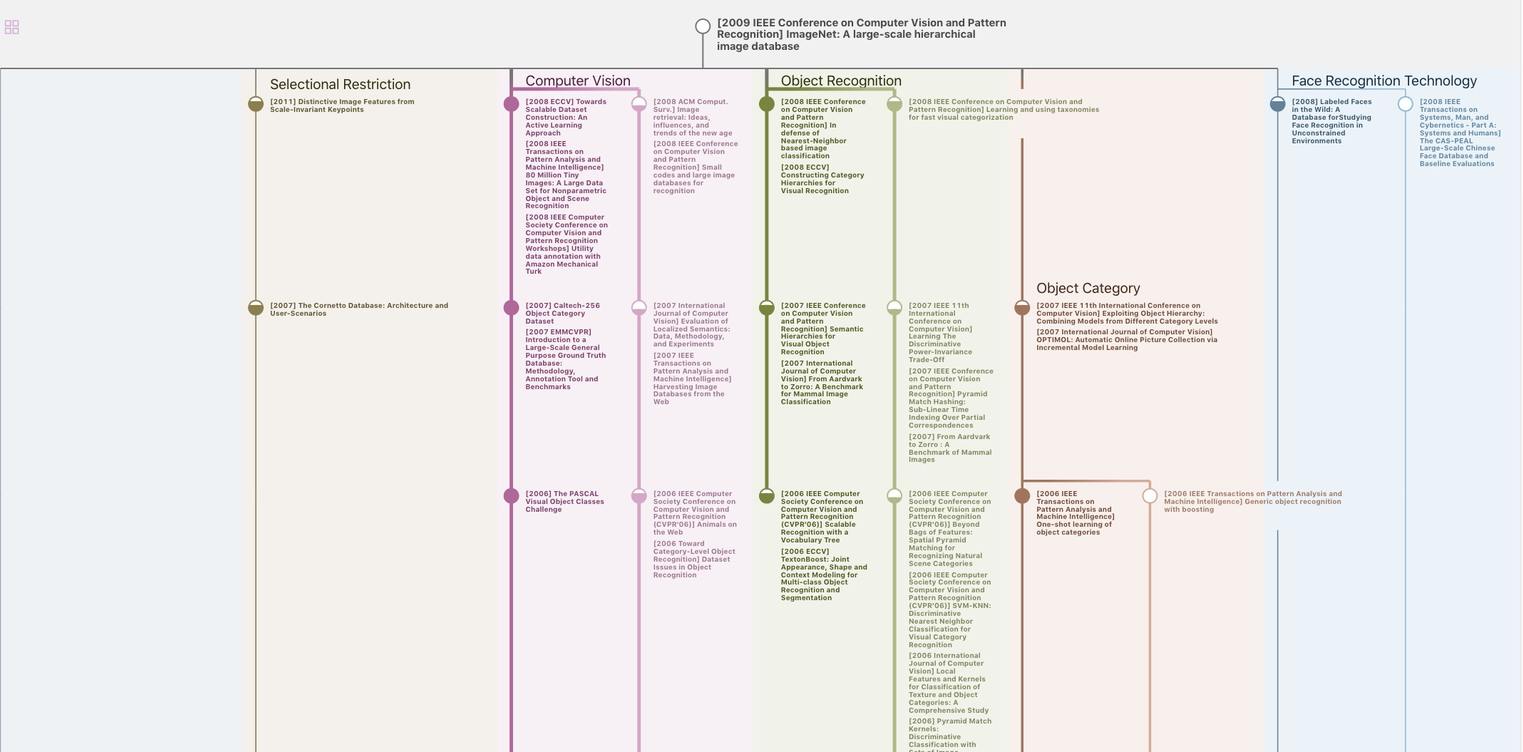
生成溯源树,研究论文发展脉络
Chat Paper
正在生成论文摘要