Local convexity of the tap free energy and amp convergence for z2-synchronization
arxiv(2023)
摘要
We study mean-field variational Bayesian inference using the TAP approach, for Z2-synchronization as a prototypical example of a high -dimensional Bayesian model. We show that for any signal strength & lambda; > 1 (the weak-recovery threshold), there exists a unique local minimizer of the TAP free energy functional near the mean of the Bayes posterior law. Furthermore, the TAP free energy in a local neighborhood of this minimizer is strongly con-vex. Consequently, a natural-gradient/mirror-descent algorithm achieves lin-ear convergence to this minimizer from a local initialization, which may be obtained by a constant number of iterations of Approximate Message Passing (AMP). This provides a rigorous foundation for variational inference in high dimensions via minimization of the TAP free energy.We also analyze the finite-sample convergence of AMP, showing that AMP is asymptotically stable at the TAP minimizer for any & lambda; > 1, and is linearly convergent to this minimizer from a spectral initialization for suffi-ciently large & lambda;. Such a guarantee is stronger than results obtainable by state evolution analyses, which only describe a fixed number of AMP iterations in the infinite-sample limit.Our proofs combine the Kac-Rice formula and Sudakov-Fernique Gaus-sian comparison inequality to analyze the complexity of critical points that satisfy strong convexity and stability conditions within their local neighbor-hoods.
更多查看译文
关键词
Variational inference,Z2 synchronization,TAP free energy,approximate message passing,natural gradient descent,nonconvex optimization,landscape analysis
AI 理解论文
溯源树
样例
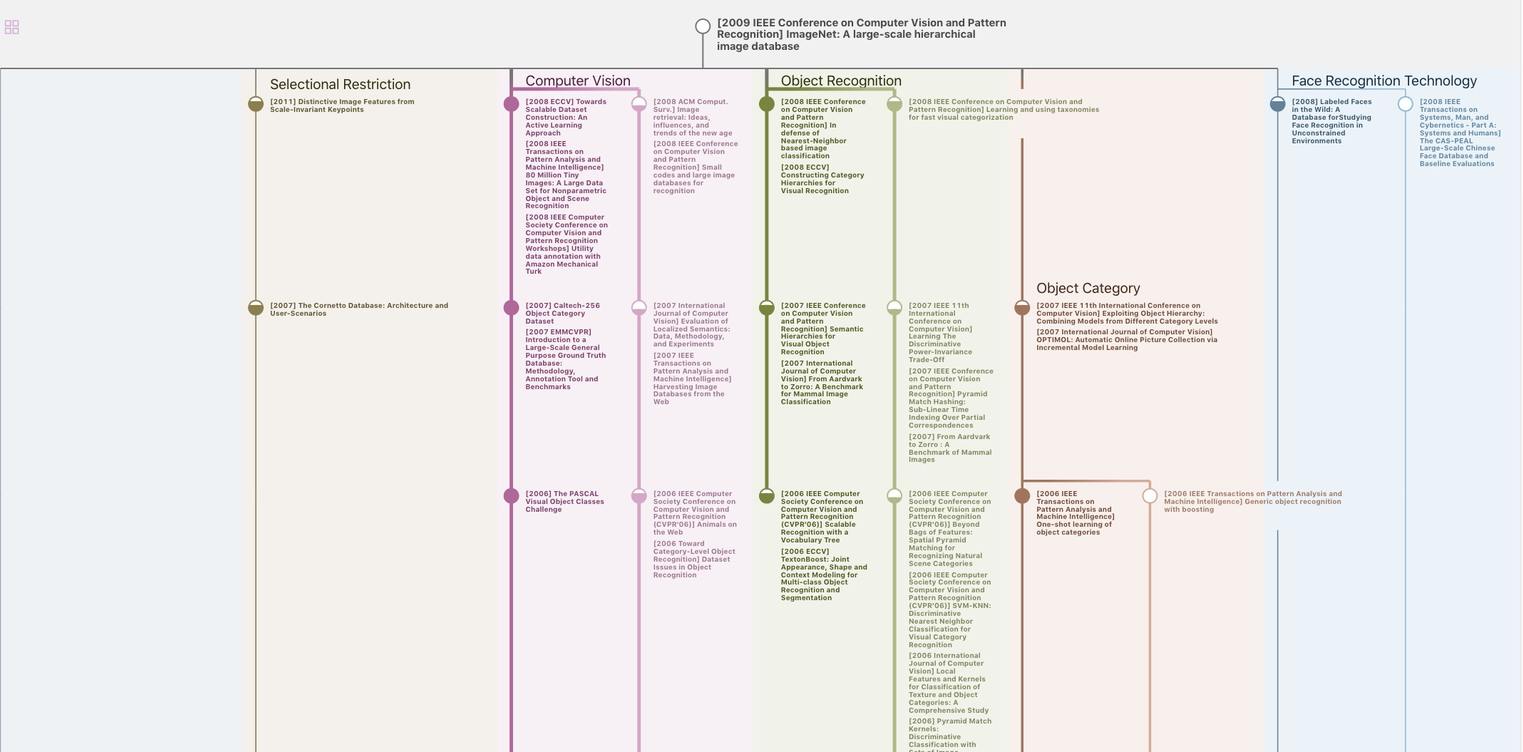
生成溯源树,研究论文发展脉络
Chat Paper
正在生成论文摘要