AOMD: An analogy-aware approach to offensive meme detection on social media
Information Processing & Management(2021)
摘要
This paper focuses on an important problem of detecting offensive analogy meme on online social media where the visual content and the texts/captions of the meme together make an analogy to convey the offensive information. Existing offensive meme detection solutions often ignore the implicit relation between the visual and textual contents of the meme and are insufficient to identify the offensive analogy memes. Two important challenges exist in accurately detecting the offensive analogy memes: i) it is not trivial to capture the analogy that is often implicitly conveyed by a meme; ii) it is also challenging to effectively align the complex analogy across different data modalities in a meme. To address the above challenges, we develop a deep learning based Analogy-aware Offensive Meme Detection (AOMD) framework to learn the implicit analogy from the multi-modal contents of the meme and effectively detect offensive analogy memes. We evaluate AOMD on two real-world datasets from online social media. Evaluation results show that AOMD achieves significant performance gains compared to state-of-the-art baselines by detecting offensive analogy memes more accurately.
更多查看译文
关键词
Offensive meme,Analogy-aware,Multi-modal learning
AI 理解论文
溯源树
样例
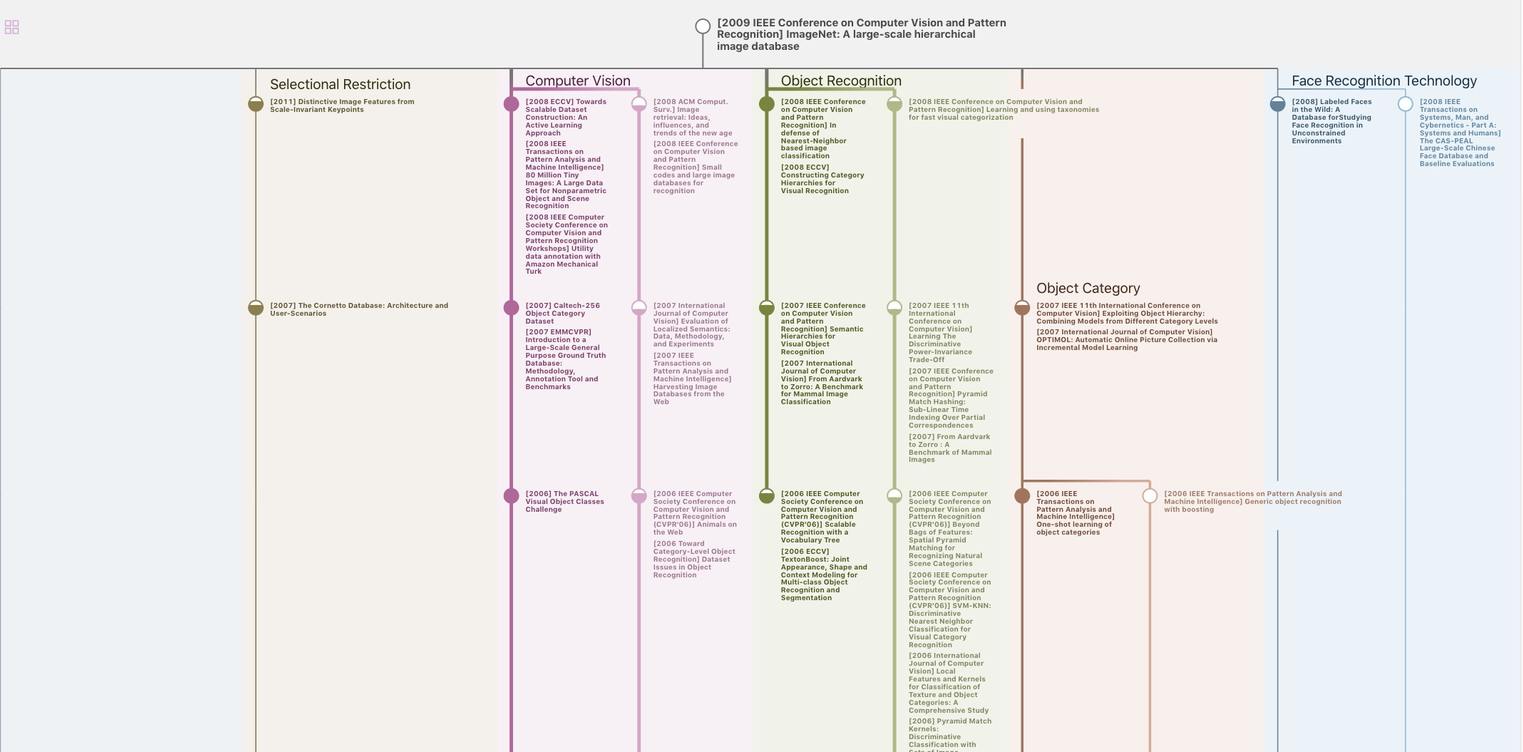
生成溯源树,研究论文发展脉络
Chat Paper
正在生成论文摘要