Approximation capabilities of measure-preserving neural networks
NEURAL NETWORKS(2022)
摘要
Measure-preserving neural networks are well-developed invertible models, however, their approximation capabilities remain unexplored. This paper rigorously analyzes the approximation capabilities of existing measure-preserving neural networks including NICE and RevNets. It is shown that for compact U c R-D with D >= 2, the measure-preserving neural networks are able to approximate arbitrary measure-preserving map psi : U -> R-D which is bounded and injective in the L-p-norm. In particular, any continuously differentiable injective map with +/- 1 determinant of Jacobian is measure-preserving, thus can be approximated. (C) 2021 Elsevier Ltd. All rights reserved.
更多查看译文
关键词
Measure-preserving,Neural networks,Dynamical systems,Approximation theory
AI 理解论文
溯源树
样例
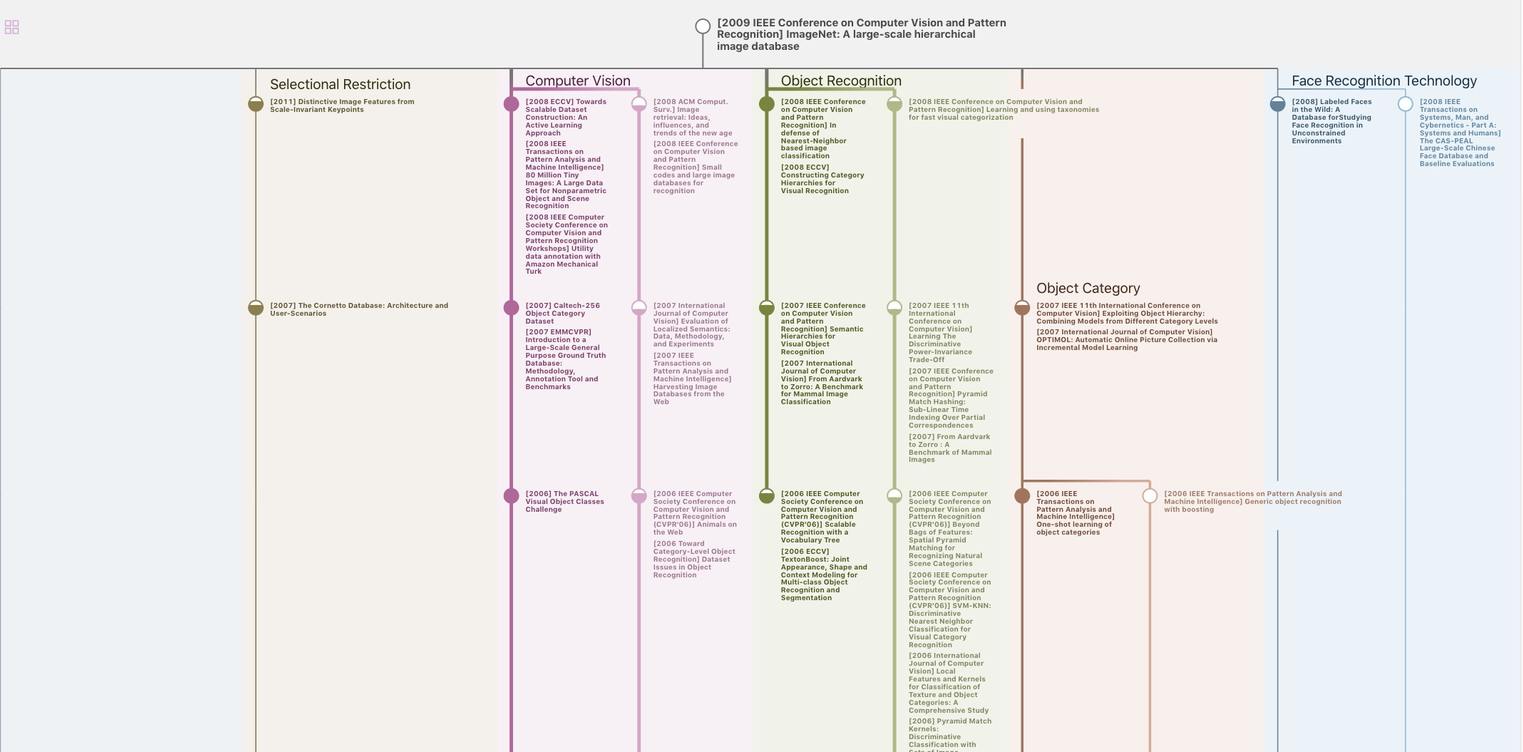
生成溯源树,研究论文发展脉络
Chat Paper
正在生成论文摘要