Trust it or not: Confidence-guided automatic radiology report generation
Neurocomputing(2024)
摘要
Medical imaging plays a pivotal role in diagnosis and treatment in clinical practice. Inspired by the significant progress in automatic image captioning, various deep learning (DL)-based methods have been proposed to generate radiology reports for medical images. Despite promising results, previous works overlook the uncertainties of their models and are thus unable to provide clinicians with the reliability/confidence of the generated radiology reports to assist their decision-making. In this paper, we propose a novel method to explicitly quantify both the visual uncertainty and the textual uncertainty for DL-based radiology report generation. Such multi-modal uncertainties can sufficiently capture the model’s confidence degree at both the report level and sentence level, which can be further leveraged to weight the losses for more comprehensive model optimization. Experimental results have demonstrated that the proposed method for model uncertainty characterization and estimation can produce more reliable confidence scores for radiology report generation, and the modified loss function, which takes into account the uncertainties, leads to better model performance on two public radiology report datasets based on both standard evaluation metrics and experienced radiologists.
更多查看译文
关键词
Radiology report generation,Uncertainty estimation,Image captioning
AI 理解论文
溯源树
样例
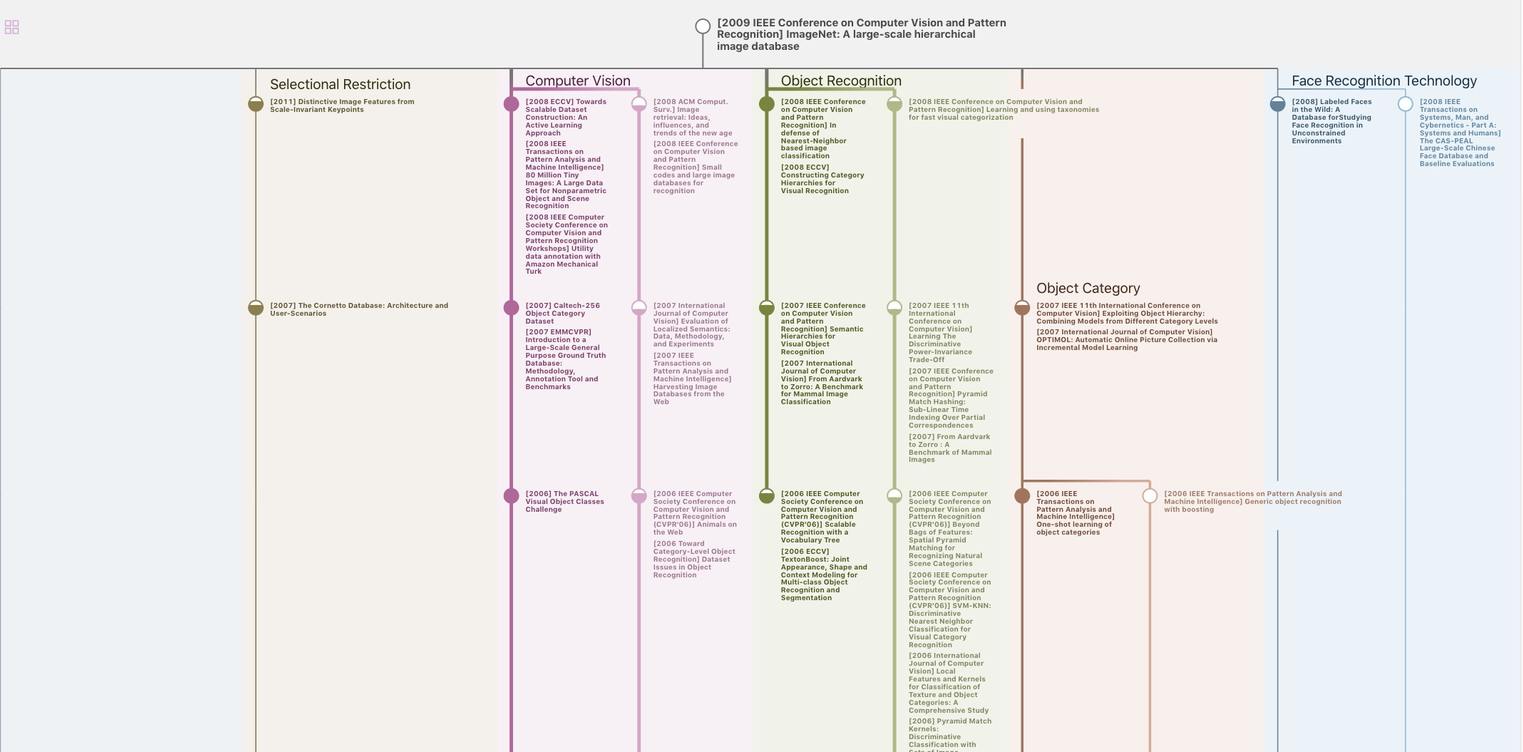
生成溯源树,研究论文发展脉络
Chat Paper
正在生成论文摘要