Bayesian inference for continuous-time hidden Markov models with an unknown number of states
STATISTICS AND COMPUTING(2021)
摘要
We consider the modeling of data generated by a latent continuous-time Markov jump process with a state space of finite but unknown dimensions. Typically in such models, the number of states has to be pre-specified, and Bayesian inference for a fixed number of states has not been studied until recently. In addition, although approaches to address the problem for discrete-time models have been developed, no method has been successfully implemented for the continuous-time case. We focus on reversible jump Markov chain Monte Carlo which allows the trans-dimensional move among different numbers of states in order to perform Bayesian inference for the unknown number of states. Specifically, we propose an efficient split-combine move which can facilitate the exploration of the parameter space, and demonstrate that it can be implemented effectively at scale. Subsequently, we extend this algorithm to the context of model-based clustering, allowing numbers of states and clusters both determined during the analysis. The model formulation, inference methodology, and associated algorithm are illustrated by simulation studies. Finally, we apply this method to real data from a Canadian healthcare system in Quebec.
更多查看译文
关键词
Bayesian model selection,Continuous-time processes,Hidden Markov models,Markov chain Monte Carlo,Reversible jump algorithms,Model-based clustering
AI 理解论文
溯源树
样例
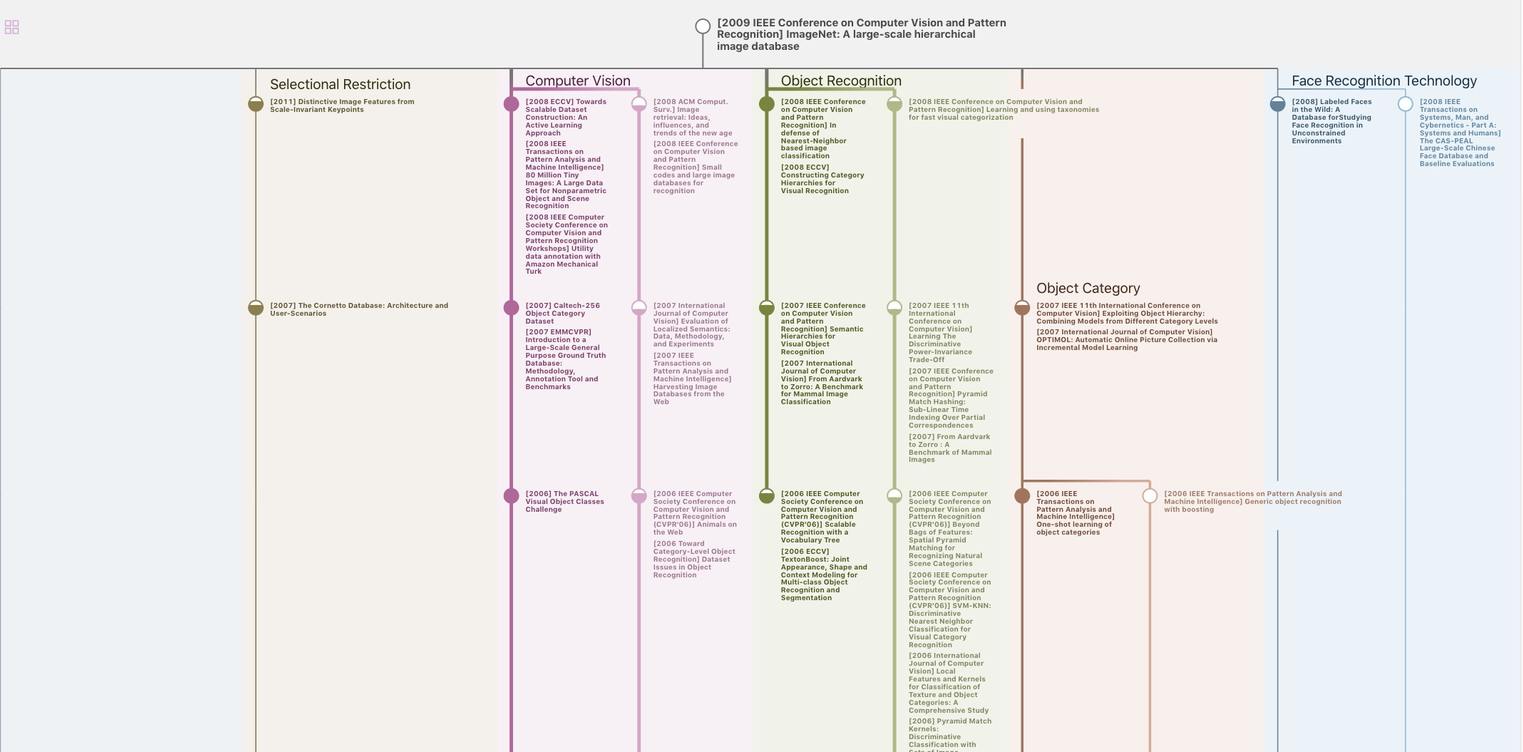
生成溯源树,研究论文发展脉络
Chat Paper
正在生成论文摘要